A multi-network integration approach for measuring disease similarity based on ncRNA regulation and heterogeneous information
BMC Bioinformatics(2022)
摘要
Background Measuring similarity between complex diseases has significant implications for revealing the pathogenesis of diseases and development in the domain of biomedicine. It has been consentaneous that functional associations between disease-related genes and semantic associations can be applied to calculate disease similarity. Currently, more and more studies have demonstrated the profound involvement of non-coding RNA in the regulation of genome organization and gene expression. Thus, taking ncRNA into account can be useful in measuring disease similarities. However, existing methods ignore the regulation functions of ncRNA in biological process. In this study, we proposed a novel deep-learning method to deduce disease similarity. Results In this article, we proposed a novel method, ImpAESim, a framework integrating multiple networks embedding to learn compact feature representations and disease similarity calculation. We first utilize three different disease-related information networks to build up a heterogeneous network, after a network diffusion process, RWR, a compact feature learning model composed of classic Auto Encoder (AE) and improved AE model is proposed to extract constraints and low-dimensional feature representations. We finally obtain an accurate and low-dimensional feature representation of diseases, then we employed the cosine distance as the measurement of disease similarity. Conclusion ImpAESim focuses on extracting a low-dimensional vector representation of features based on ncRNA regulation, and gene–gene interaction network. Our method can significantly reduce the calculation bias resulted from the sparse disease associations which are derived from semantic associations.
更多查看译文
关键词
Non-coding RNA, Disease similarity, Semantic association, Gene functional network
AI 理解论文
溯源树
样例
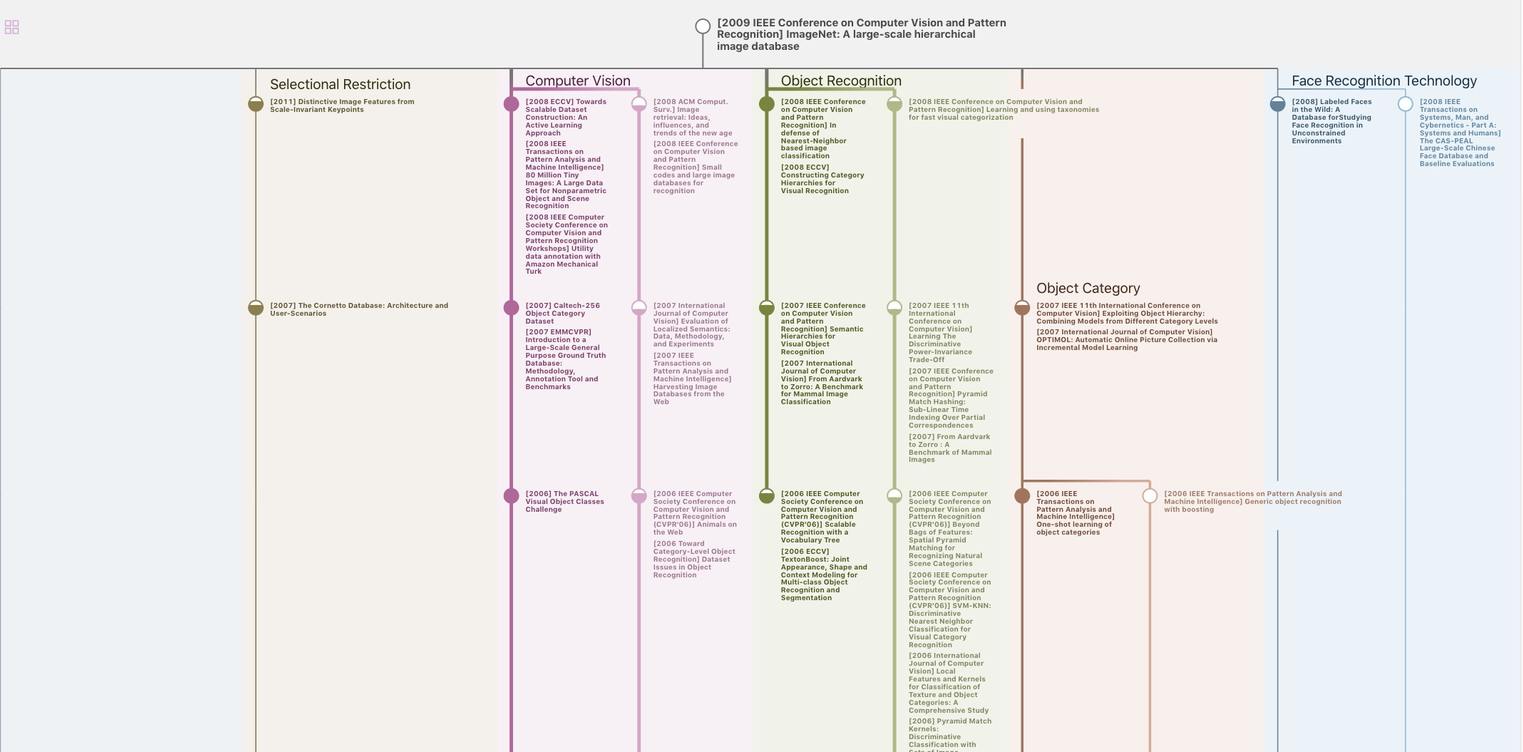
生成溯源树,研究论文发展脉络
Chat Paper
正在生成论文摘要