Dual Branch Relation Network with Feature Weighting for Few-Shot Learning
2021 7th International Conference on Computer and Communications (ICCC)(2021)
摘要
Few-shot learning is proposed to enable classifier to rapidly generalize to novel classes with limited exemplars on the basis of prior knowledge. Nevertheless, the majority of models which base on the metric learning still have mediocre performance because of the neglect of the critical part misalignment in spatial position between images. In order to address this issue, We design a metric learning based model with two branches called dual branch relation network (DBRN). One branch measure the similarity between support image and query image directly by using relation network as the measurement network. Another branch measures the similarity of image pairs in consideration of the relationship between every local region of query image and support image. The final matching score is the fusion of results from two branches. Before the two branches measuring similarity, we introduce a spatial-wise feature weighting method to enhance the similar local features between images and a channel-wise feature weighting method to weight the channels of features by using class attentive weighting vector. In experiments, our model achieve the state-of-the-art performance on two major benchmark datasets in few-shot image recognition.
更多查看译文
关键词
few-shot learning,feature weighting,primary object alignment
AI 理解论文
溯源树
样例
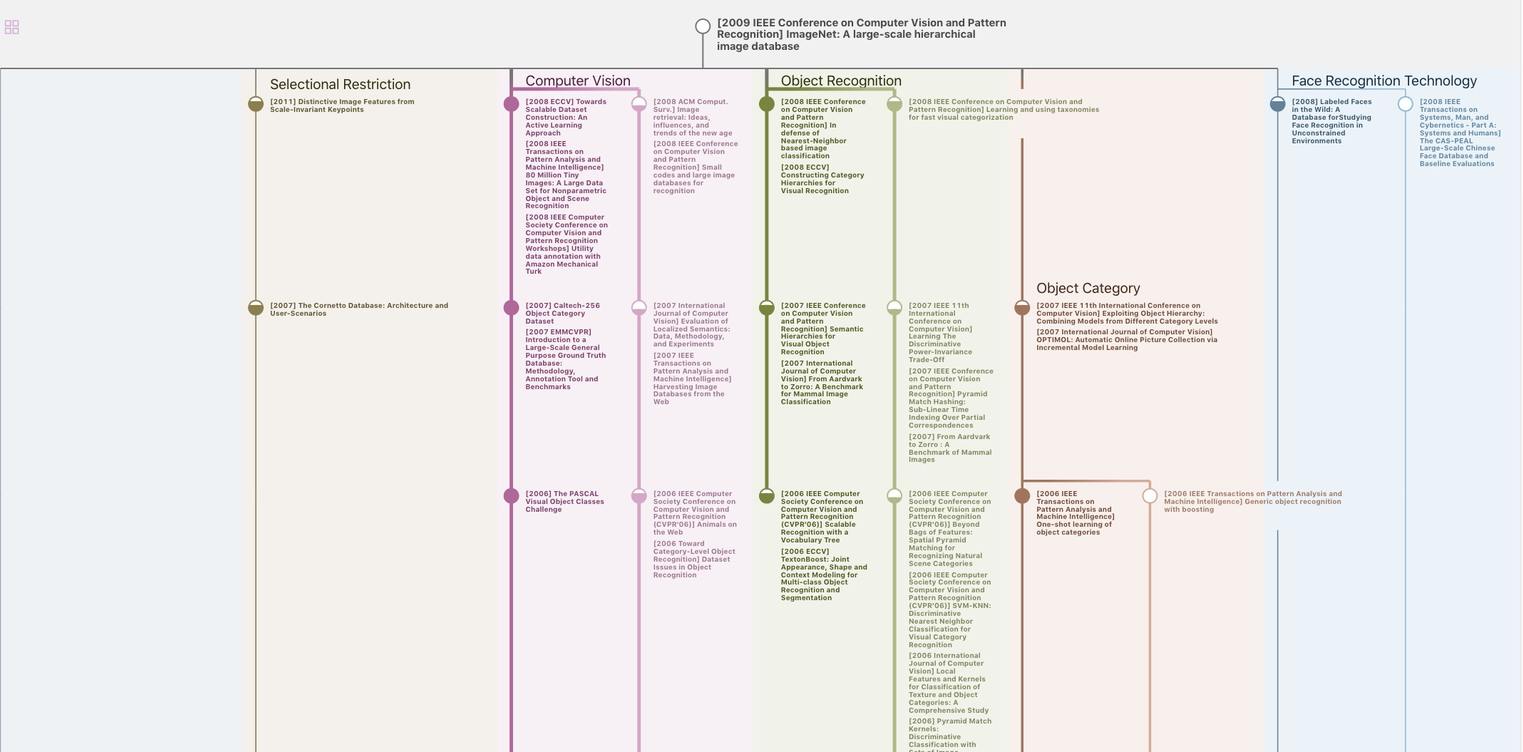
生成溯源树,研究论文发展脉络
Chat Paper
正在生成论文摘要