Dynamic Graph-Level Neural Network for SAR Image Change Detection
IEEE GEOSCIENCE AND REMOTE SENSING LETTERS(2022)
摘要
The graph neural network (GNN) has been widely applied to image analysis and recognition. Recently, a semisupervised graph convolutional network (ssGCN) method has been proposed to change detection and obtains promising performance on very-high-resolution remote sensing images. However, a synthetic aperture radar (SAR) image is subject to speckle noise, and there is no explicit structure. In this letter, an end-to-end dynamic graph-level neural network (DGLNN) is proposed to exploit the local structure of each pixel neighborhood block at a graph level and learn a more discriminative graph for change detection. Moreover, in the training of DGLNN, a K-nearest neighborhood is employed to reconstruct edges between nodes instead of the fixed edges between two nodes so that each node exploits the features from different neighbor nodes. The proposed method is verified by cross-domain SAR image change detection on four sets of SAR images and compared with five state-of-the-art deep-learning-based SAR image change detection methods. The overall experimental results show that the proposed DGLNN obtains outstanding performance.
更多查看译文
关键词
Change detection, cross-dataset, graph neural network (GNN), graph-lever classification, synthetic aperture radar (SAR) Image
AI 理解论文
溯源树
样例
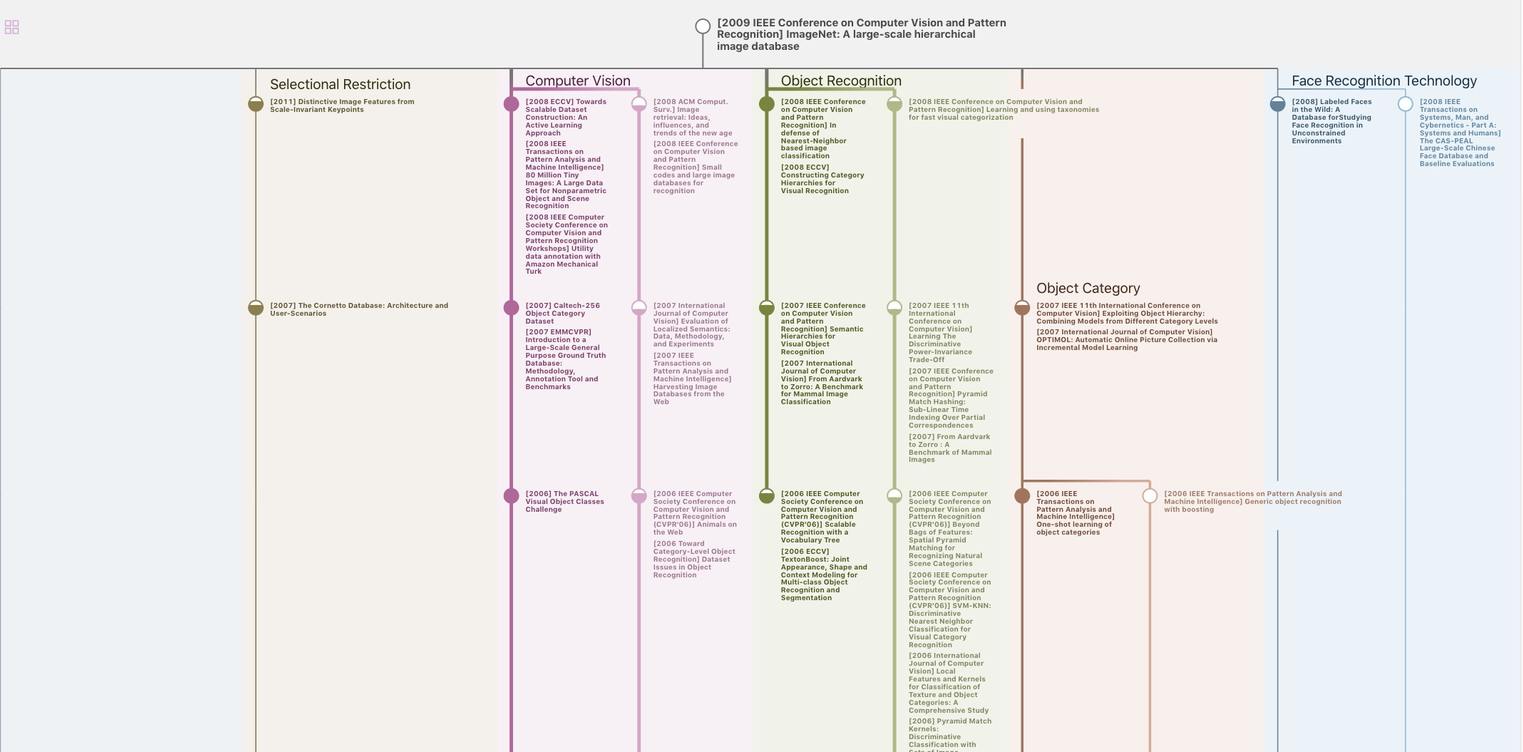
生成溯源树,研究论文发展脉络
Chat Paper
正在生成论文摘要