Federated Multi-task Learning for HyperFace
IEEE transactions on artificial intelligence(2021)
摘要
Multitask learning (MTL) is a promising field in machine learning owing to its capability to improve the generalization performance of all tasks by sharing knowledge among the related tasks. MTL has attracted a large amount of attention in the multitask-related community. In recent years, with the rapid development of distributed machine learning, MTL in a distributed environment becomes a hot research topic. Although MTL can reap great fruit in a distributed environment, the sensitive information, such as photos and voice recordings of the owner, in distributed data may be leaked during training. Consequently, in the distributed settings, one prominent challenge of MTL is to prevent the disclosure of sensitive private data. In this article, we propose a novel approach, federated multitask learning (FMTL), which applies federated learning to MTL in a distributed environment in order to protect the MTL model from being leaked and to better optimize the utility of the MTL model. In FMTL, all the participants independently train their local models on their own dataset in parallel and only transmit their model updates to the central server for aggregation at every epoch. As a result, their learning accuracy is enhanced beyond what is achieved only on their own training data. In this manner, it can achieve the best tradeoff between the utility and privacy: participants not only preserve the privacy of their own data, but also benefit from the models of other participants. The experimental results on annotated facial landmarks in the wild (AFLW) and annotated faces in the wild (AFW) benchmark datasets verify the effectiveness of our framework.
更多查看译文
关键词
Distributed multitask learning,federated multitask learning (FMTL),privacy preserving,sensitive and private information
AI 理解论文
溯源树
样例
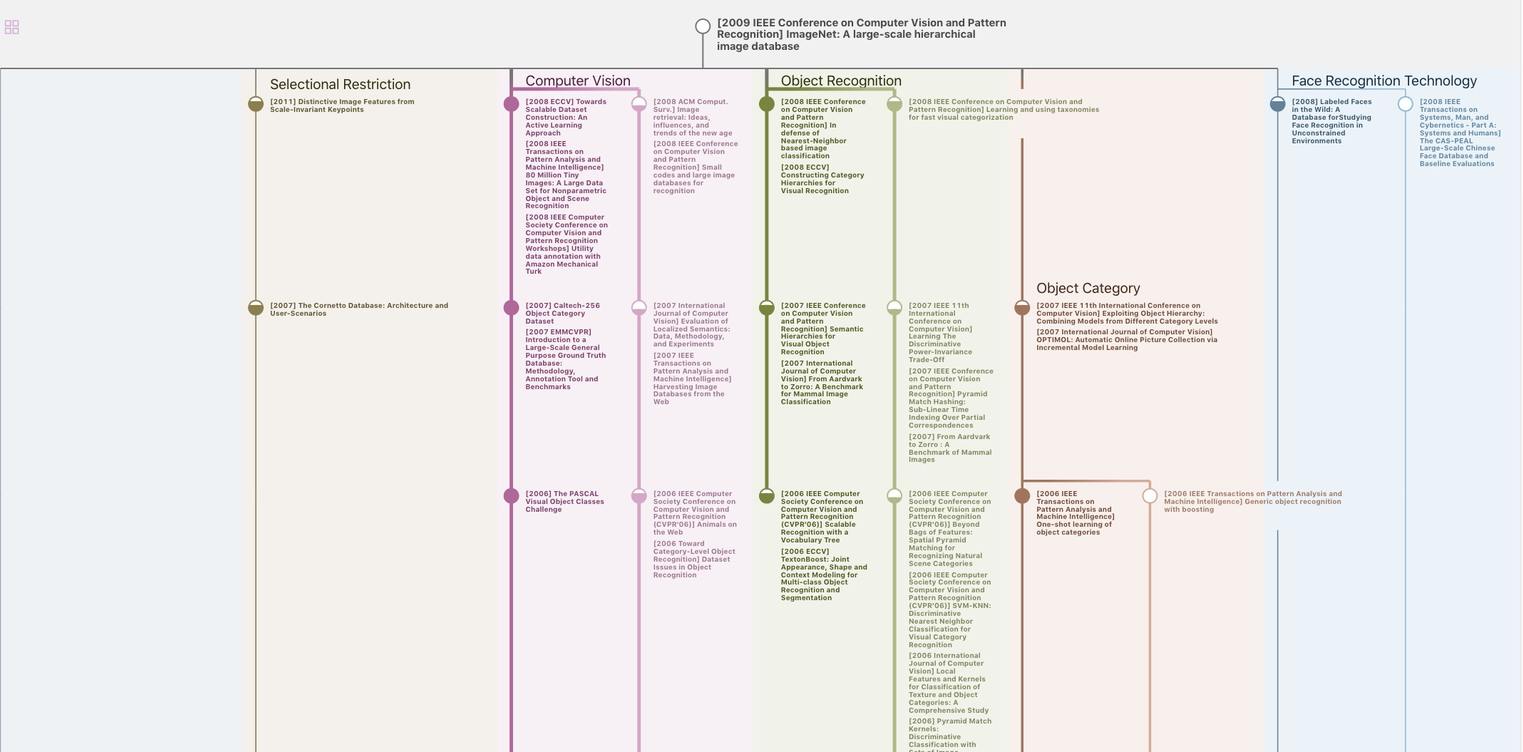
生成溯源树,研究论文发展脉络
Chat Paper
正在生成论文摘要