Data Augmentation Using DCGAN for Improved Fault Detection of High Voltage Shunt Reactor
Journal of physics(2021)
摘要
High voltage shunt reactor is an important equipment of power transmission systems. The accurate assessment of their operating status and the timely and correct diagnosis of faults and defects concern the operation safety of the entire grid. Health assessment of high voltage shunt reactors based on vibration signal, which can be used to characterize the hidden troubles of it, is a topic widely studied in deep learning and fault diagnosis. A large number of samples are needed to train the deep learning model, but it is not easy to acquire enough fault samples in the actual scene. In this paper, we utilize a Deep Convolutional Generative Adversarial Networks (DCGAN) to generate synthetic fault samples and enlarge the fault dataset to train the Convolution Neural Network (CNN) fault detection model. Results reveal that the performance through the CNN model can be improved by 3% with the synthetic samples generated by DCGAN, which is better than that of traditional Synthetic Minority Oversampling Technique (SMOTE) algorithm.
更多查看译文
关键词
dcgan,improved fault detection,data augmentation
AI 理解论文
溯源树
样例
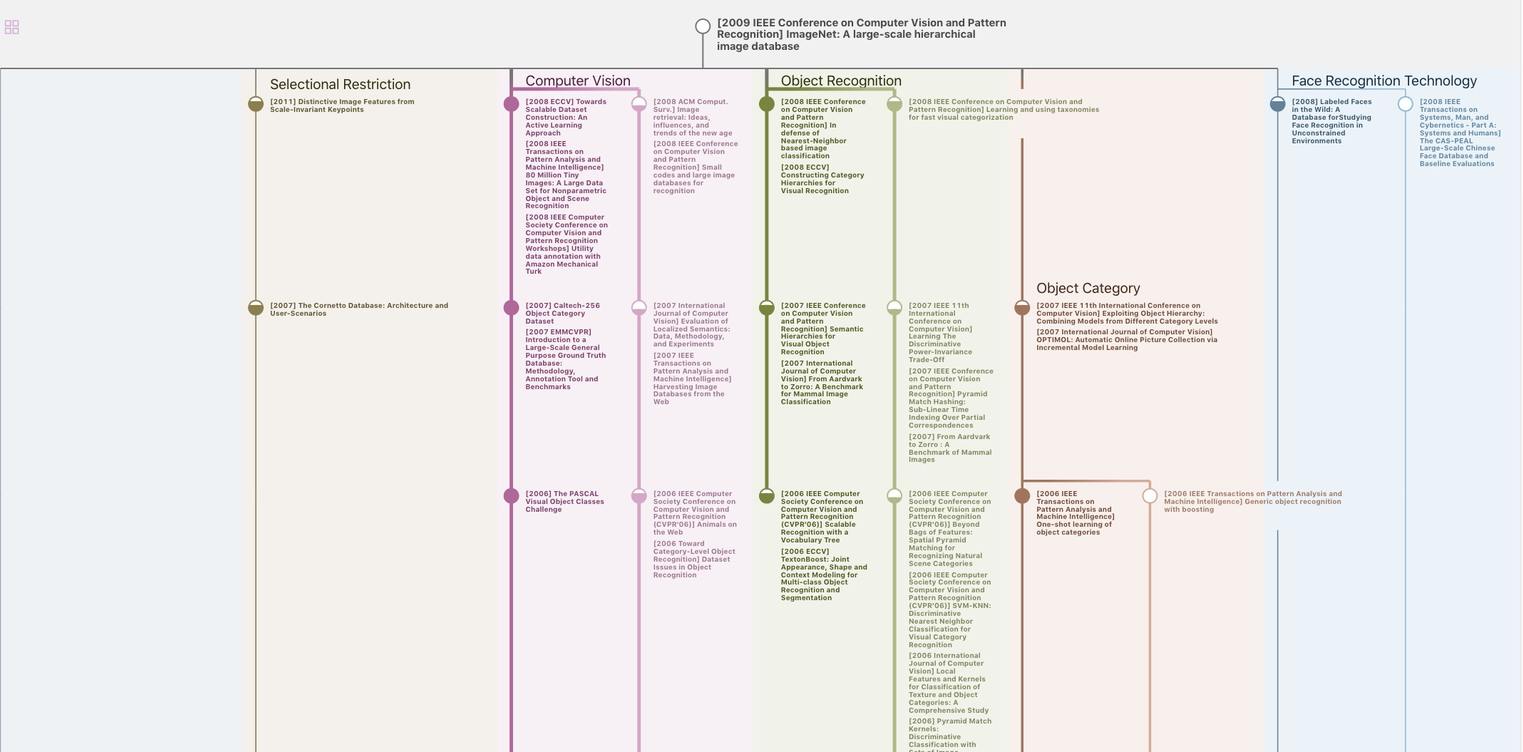
生成溯源树,研究论文发展脉络
Chat Paper
正在生成论文摘要