Deep High-Order Tensor Convolutional Sparse Coding for Hyperspectral Image Classification
IEEE TRANSACTIONS ON GEOSCIENCE AND REMOTE SENSING(2022)
摘要
Most hyperspectral image (HSI) data exist in the form of tensor; the tensor representation preserves the potential spatial–spectral structure information compared with the vector representation, which can help improve the classification performance of HSI. In this article, a deep high-order tensor convolutional sparse coding (CSC) model is proposed, which can be used to train deep high-order filters. Based on the deep high-order tensor CSC model, a deep feature extraction network (DHTCSCNet) is constructed, which is used for feature extraction of HSIs. By combining the spectral–spatial feature and the features extracted by the proposed DHTCSCNet at each layer, a combined feature that incorporates shallow, deep, spectral, and spatial features can be obtained. Then, the graph-based learning (GSL) methods are used to classify the combined feature. Experimental results show that the DHTCSCNet can obtain better classification performance compared with other HSI classification methods.
更多查看译文
关键词
Feature extraction,Tensors,Convolutional codes,Hyperspectral imaging,Kernel,Image coding,Convolution,Deep high-order tensor convolutional sparse coding (CSC),deep learning,graph-based learning (GSL),hyperspectral image (HSI) classification
AI 理解论文
溯源树
样例
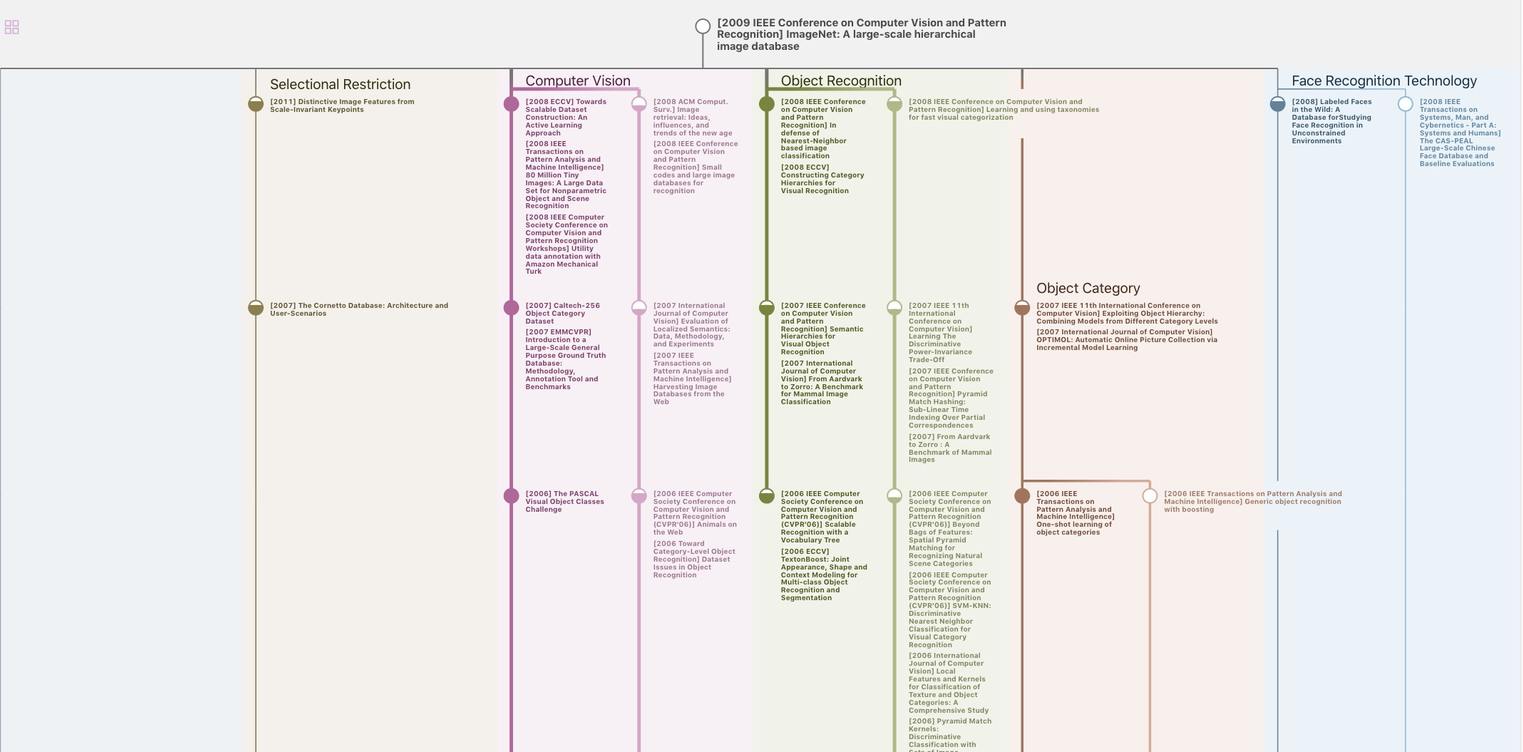
生成溯源树,研究论文发展脉络
Chat Paper
正在生成论文摘要