Automatic Framework of Mapping Impervious Surface Growth With Long-Term Landsat Imagery Based on Temporal Deep Learning Model
IEEE GEOSCIENCE AND REMOTE SENSING LETTERS(2022)
摘要
The impervious surface (IS) cover and its dynamics are key parameters in research about urban and ecology. This letter proposed an automatic framework to map the IS growth end-to-end based on the temporal deep learning (DL) model and long time-series Landsat imagery. First, the training and validating datasets were auto-generated by a joint strategy. Then, a DL network was designed, and the IS growth was predicted in temporal windows. Finally, the results from multi-temporal windows are combined to generate the IS growth map. The data around the core of Beijing, China, is tested, and the result shows that the proposed method could: 1) efficiently model the IS growth; 2) map IS growth with less salt-and-pepper noise and false alarm compared to existing products; and 3) be extended to future data easily.
更多查看译文
关键词
Earth,Remote sensing,Artificial satellites,Training,Deep learning,Charge coupled devices,Spatial resolution,Change detection,deep learning (DL),impervious surface (IS),Landsat,sample generation
AI 理解论文
溯源树
样例
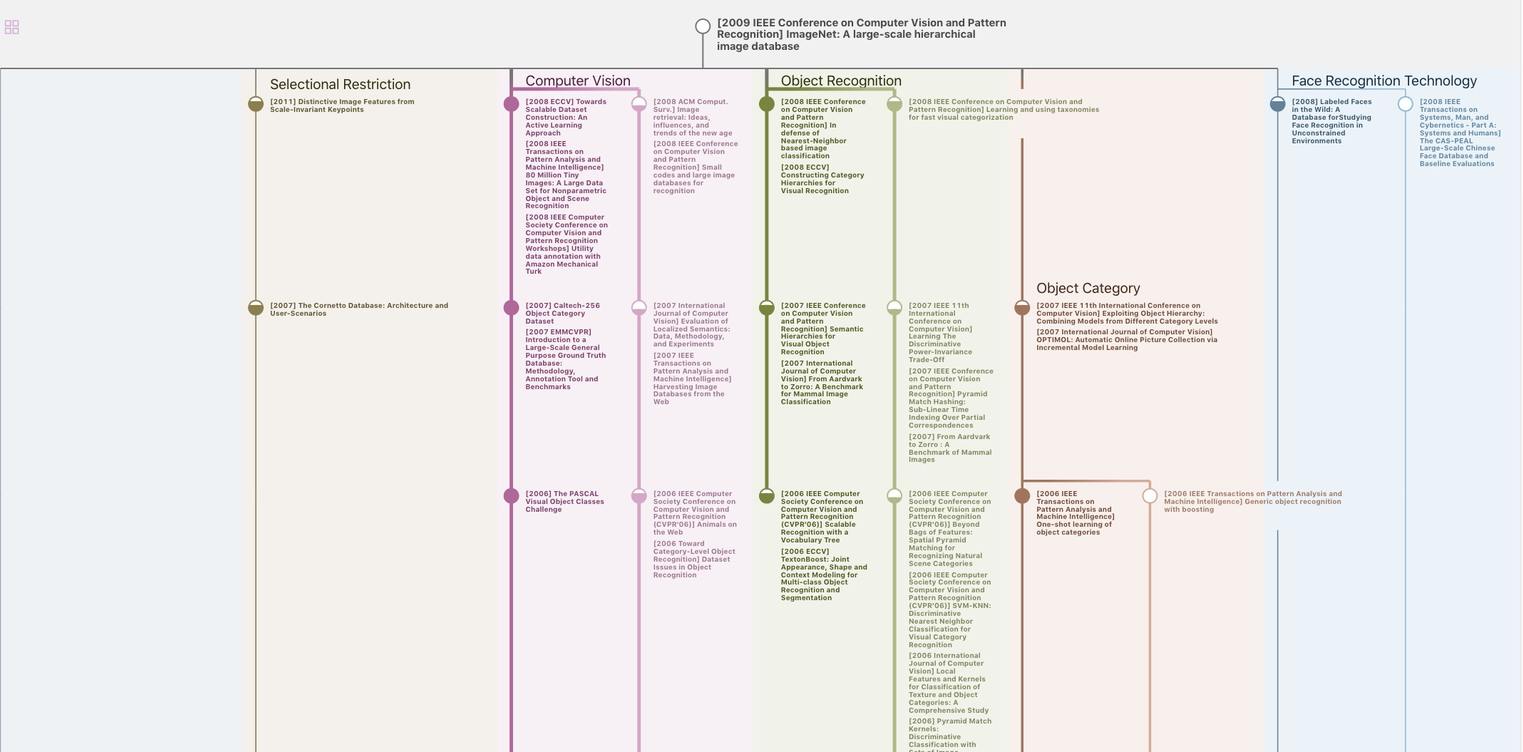
生成溯源树,研究论文发展脉络
Chat Paper
正在生成论文摘要