A pseudo-inverse decomposition-based self-organizing modular echo state network for time series prediction
Applied Soft Computing(2022)
摘要
Echo state network (ESN) refers to a popular recurrent neural network with a largely and randomly generated reservoir for its rapid learning ability. However, it is difficult to design a reservoir that matches a specific task. To solve the structure design of the reservoir, a pseudo-inverse decompositionbased self-organizing modular echo state (PDSM-ESN) is proposed. PDSM-ESN is constructed by growing-pruning method, where the error and condition number are used, respectively. Since the self-organizing process may negatively affect the learning speed, the pseudo-inverse decomposition is adopted to improve learning speed, which means the output weights are learned by an iterative incremental method. Meanwhile, to solve the ill-posed problem, the modular sub-reservoirs corresponding to the high condition number are pruned. Simulation results indicate that PDSM-ESN has better prediction performance and run-time complexity compared with the traditional ESN models. (c) 2021 Elsevier B.V. All rights reserved.
更多查看译文
关键词
Echo state network, Structure design, Self-organizing, Pseudo-inverse decomposition, Ill-posed problem
AI 理解论文
溯源树
样例
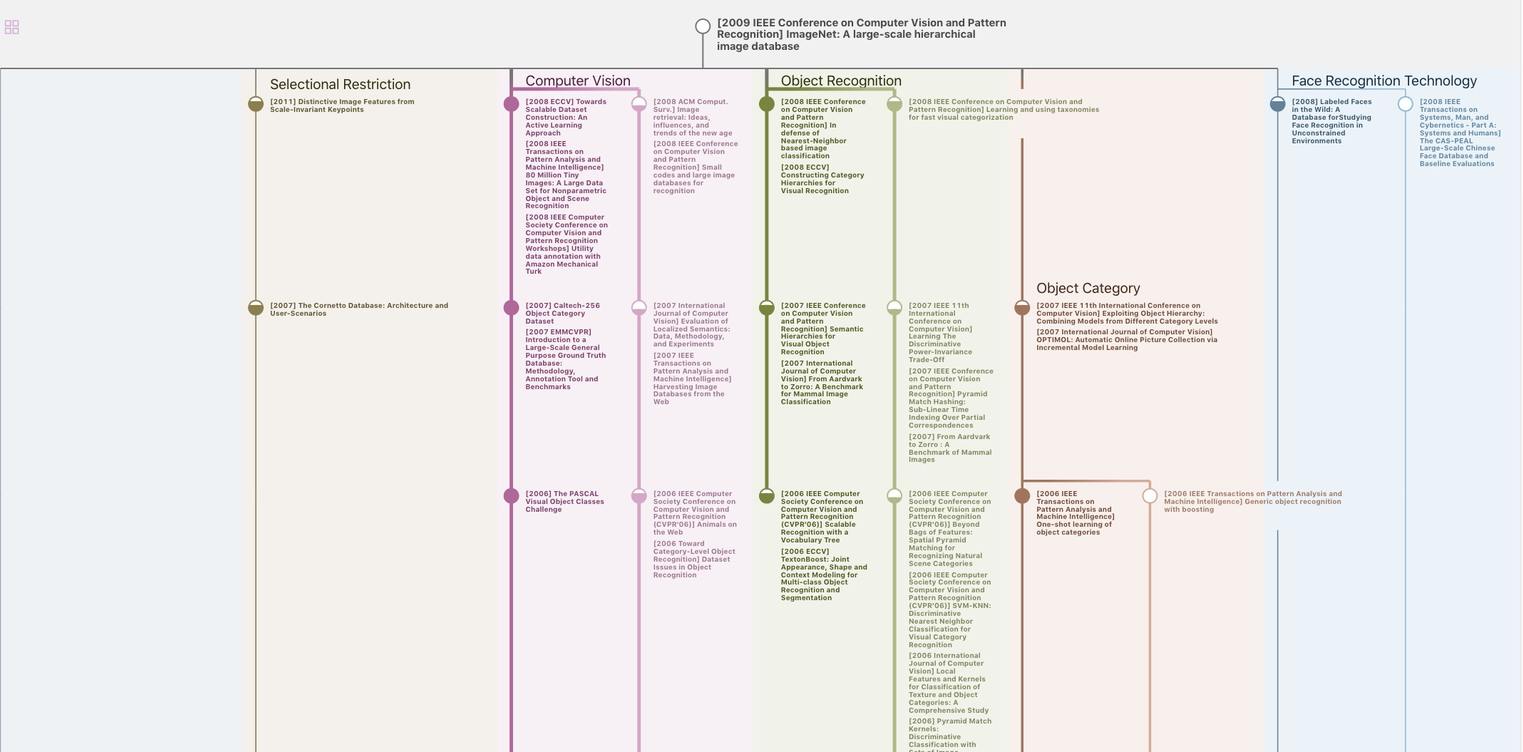
生成溯源树,研究论文发展脉络
Chat Paper
正在生成论文摘要