Joint Supervised Classification and Reconstruction of Irregularly Sampled Satellite Image Times Series
IEEE TRANSACTIONS ON GEOSCIENCE AND REMOTE SENSING(2022)
摘要
Recent satellite missions have led to a huge amount of Earth observation data, most of them being freely available. In such a context, satellite image time series have been used to study land use and land cover information. However, optical time series, such as Sentinel-2 or Landsat ones, are provided with an irregular time sampling for different spatial locations, and images may contain clouds and shadows. Thus, preprocessing techniques are usually required to properly classify such data. The proposed approach is able to deal with irregular temporal sampling and missing data directly in the classification process. It is based on Gaussian processes and allows to perform jointly the classification of the pixel labels as well as the reconstruction of the pixel time series. The method complexity scales linearly with the number of pixels, making it amenable in large-scale scenarios. Experimental classification and reconstruction results show that the method does not compete yet with state-of-the-art classifiers but yields reconstructions that are robust with respect to the presence of undetected clouds or shadows and does not require any temporal preprocessing.
更多查看译文
关键词
Image reconstruction,Time series analysis,Satellites,Remote sensing,Earth,Spatial resolution,Recurrent neural networks,Classification,Earth observation,Gaussian processes (GPs),irregular sampling,reconstruction,remote sensing,satellite image time series (SITS),Sentinel-2
AI 理解论文
溯源树
样例
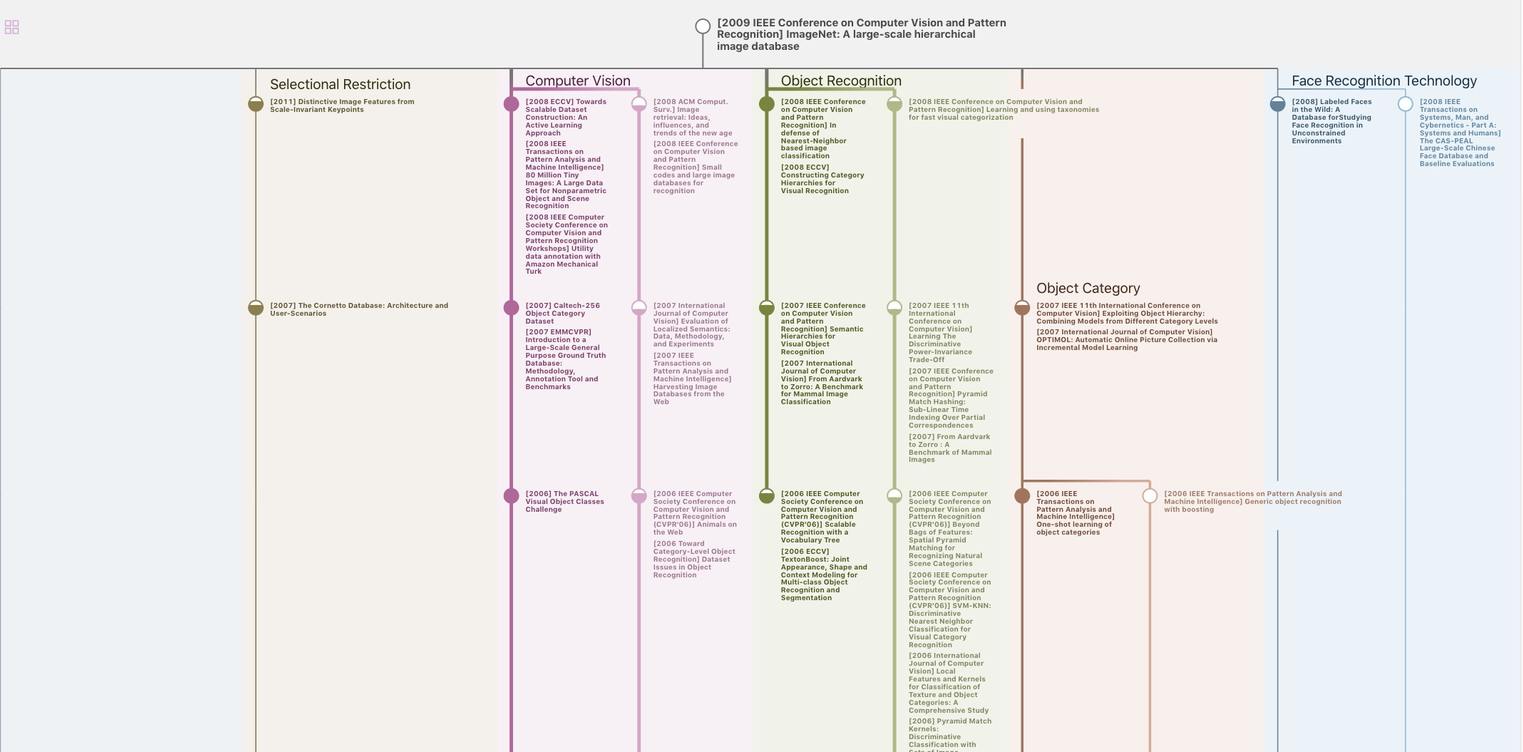
生成溯源树,研究论文发展脉络
Chat Paper
正在生成论文摘要