Bayesian statistics and modelling
Nature Reviews Methods Primers(2021)
摘要
Bayesian statistics is an approach to data analysis based on Bayes’ theorem, where available knowledge about parameters in a statistical model is updated with the information in observed data. The background knowledge is expressed as a prior distribution and combined with observational data in the form of a likelihood function to determine the posterior distribution. The posterior can also be used for making predictions about future events. This Primer describes the stages involved in Bayesian analysis, from specifying the prior and data models to deriving inference, model checking and refinement. We discuss the importance of prior and posterior predictive checking, selecting a proper technique for sampling from a posterior distribution, variational inference and variable selection. Examples of successful applications of Bayesian analysis across various research fields are provided, including in social sciences, ecology, genetics, medicine and more. We propose strategies for reproducibility and reporting standards, outlining an updated WAMBS (when to Worry and how to Avoid the Misuse of Bayesian Statistics) checklist. Finally, we outline the impact of Bayesian analysis on artificial intelligence, a major goal in the next decade.
更多查看译文
关键词
Scientific community,Statistics,Physics,general
AI 理解论文
溯源树
样例
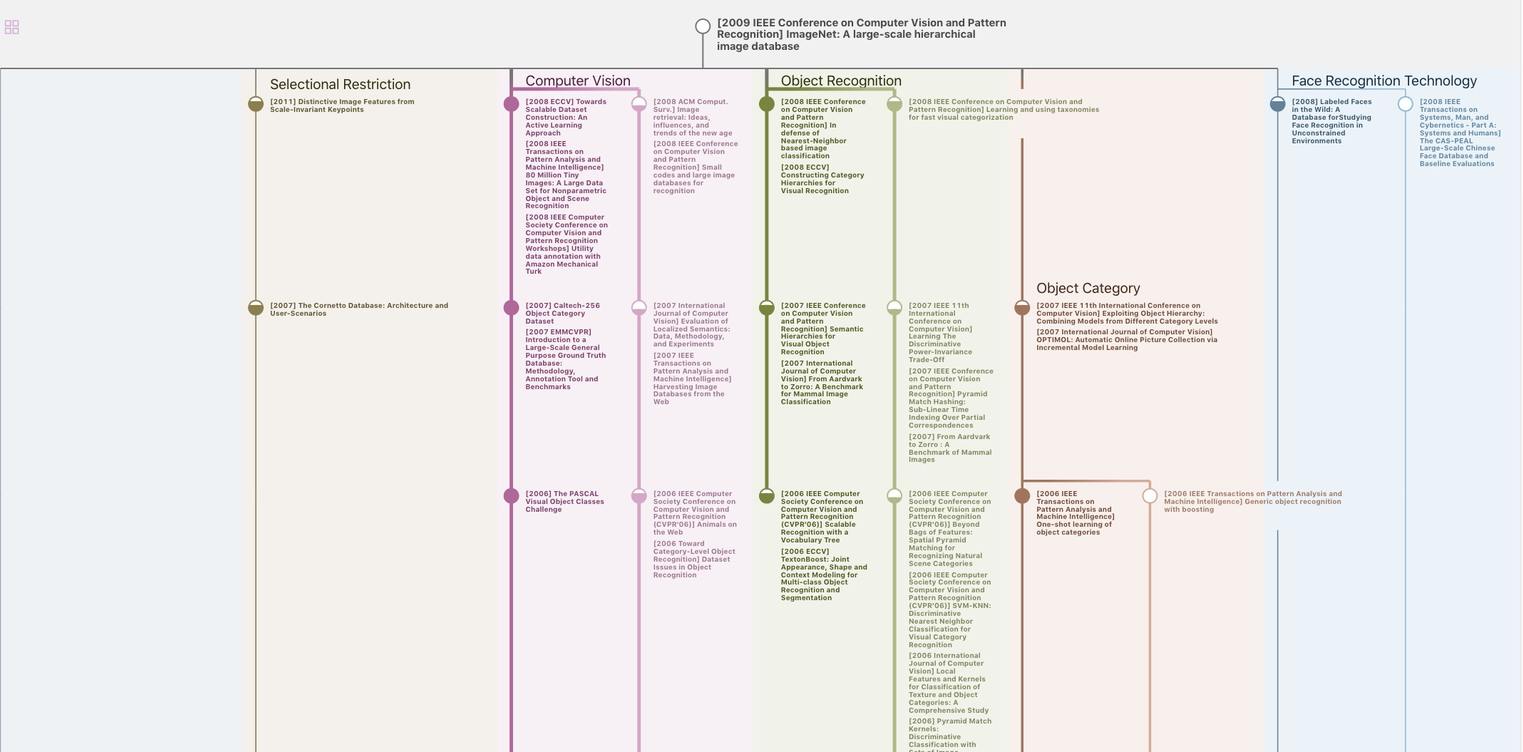
生成溯源树,研究论文发展脉络
Chat Paper
正在生成论文摘要