Learning adversarial point-wise domain alignment for stereo matching
Neurocomputing(2022)
摘要
The state-of-the-art stereo matching models trained on synthetic datasets have difficulty in generalizing to real-world datasets. One major reason is that illumination and texture in the real world are hard to be simulated, resulting in big differences between synthetic and real-world data. In this study, instead of narrowing the image-level appearance difference, we focus on aligning both data domains in feature space in an unsupervised manner and propose an end-to-end domain alignment stereo network (DAStereo). A domain alignment module (DAM) is introduced by learning a point-wise linear transformation. We demonstrate that DAM can maintain sufficient alignment capacity with fewer parameters than the globally nonlinear mapping. To explicitly promote the point-wise domain alignment, adversarial learning is further introduced using a cost volume discriminator in a hybrid training manner. Experimental results show that DAStereo outperforms the state-of-the-art unsupervised and adaptive methods and even achieves comparable performance to some supervised methods.
更多查看译文
关键词
Stereo Matching,Domain adaptation,Point-wise linear transformation,Adversarial learning
AI 理解论文
溯源树
样例
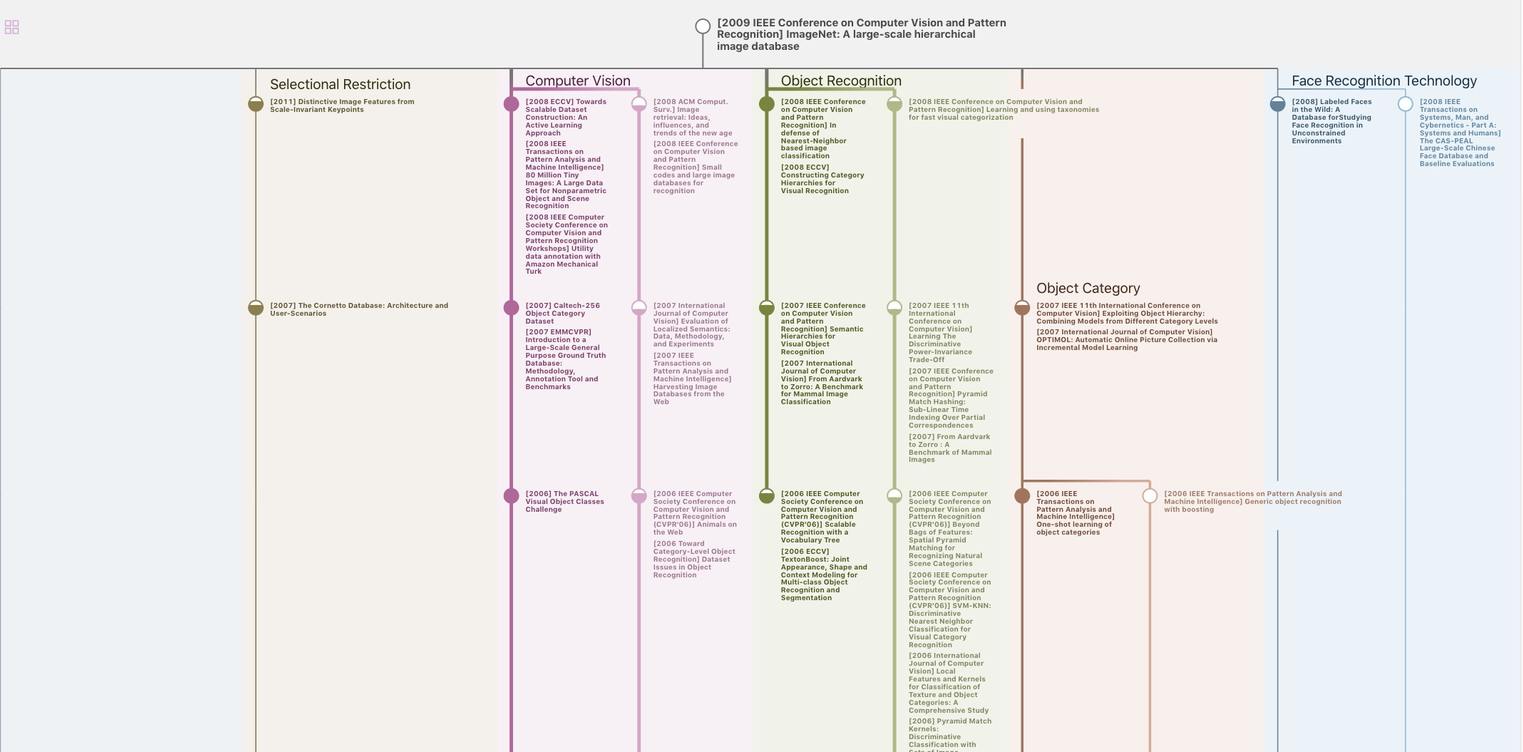
生成溯源树,研究论文发展脉络
Chat Paper
正在生成论文摘要