FILTER PRUNING IN ORDER OF IMPORTANCE OF THE LAYER GROUPS TO ACCELERATE CONVOLUTIONAL NEURAL NETWORKS
The Herald of KSUCTA, №3, 2021The herald of KSUCTA n a N Isanov(2021)
摘要
Various acceleration approaches have been studied to deploy convolutional neural networks in embedded devices. Among them, filter pruning is the most active research because it is easy to implement in hardware and keeps high accuracy while reducing the computational and memory cost. In this paper, we propose a method of grouping layers, finding the importance of each group, and groupwise pruning according to the order of importance to achieve high FLOPs reduction while retaining high accuracy. First, we divide the layers of the pre-trained network into groups according to the size of the output feature map. Next, we calculate the importance score per group using first-order Taylor expansion. Finally, filter pruning is performed in order from the group with the highest importance score. When pruning VGG and ResNet trained on CIFAR-10, our proposed method shows superior performance in accuracy and FLOPs compared to the state-of-art methods. Notably, on ResNet-50, we achieve 70.85% FLOPs reduction by removing 50% of the filters, with a slight loss of 0.41% in the baseline accuracy.
更多查看译文
AI 理解论文
溯源树
样例
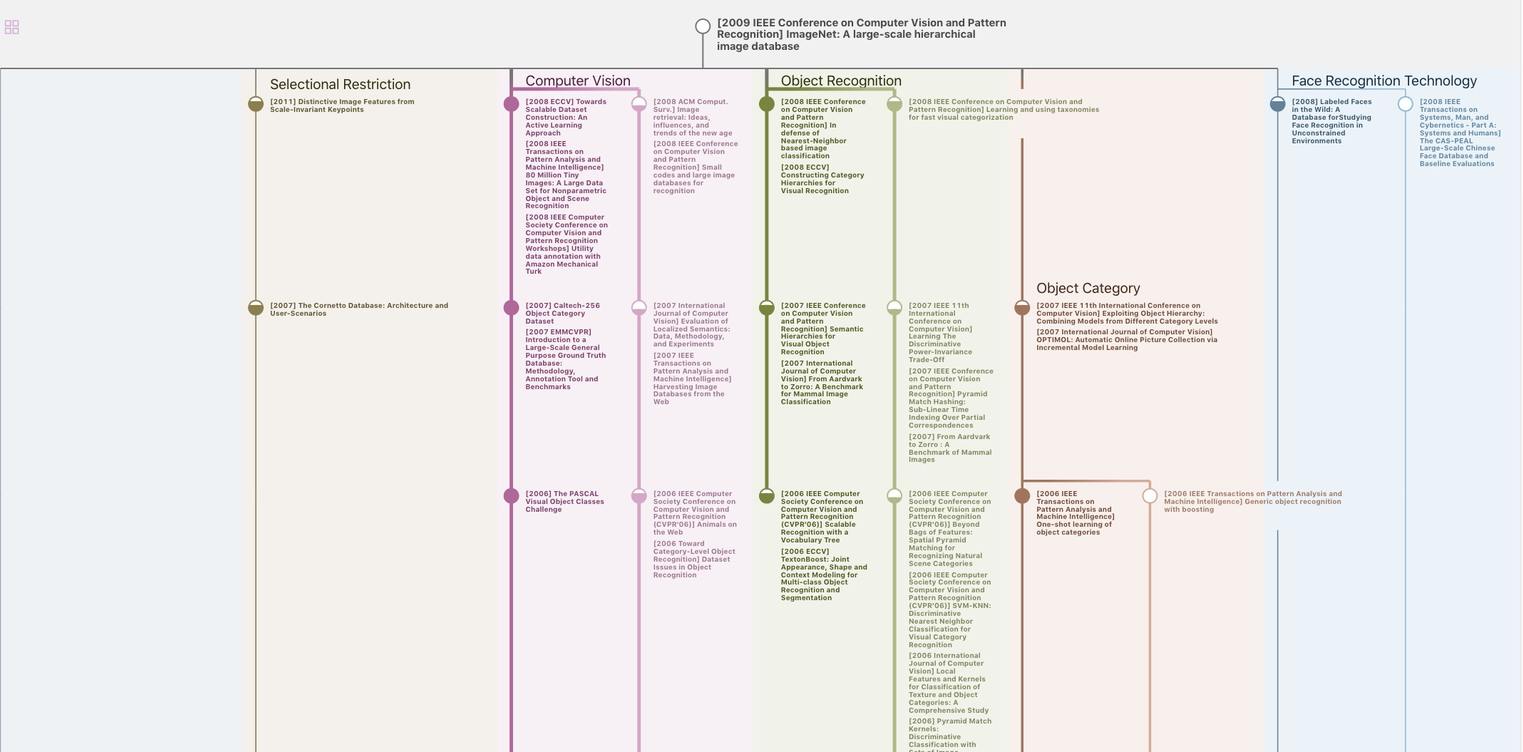
生成溯源树,研究论文发展脉络
Chat Paper
正在生成论文摘要