Development of a QSAR model to predict comedogenic potential of some cosmetic ingredients
Computational Toxicology(2022)
摘要
• Non-animal-based model to evaluate the comedogenic potential of cosmetics. • Random Forest showed the best prediction performance as a classifier. • Cross-validated QSAR modelling results for Mold2 and alvaDesc models are promising. • Learning-based feature selection method allowed finding the significant descriptors. • The predictive ability of alvaDesc model is characterized as good. Comedogenicity is a common adverse reaction to cosmetic ingredients that cause blackheads or pimples by blocking the pores, especially for acne-prone skin. Before animal testing was banned by European Commission in 2013, comedogenic potential of cosmetics were tested on rabbits. However, full replacement of animal tests by alternatives has not been possible yet. Therefore, there is a need for applying new approach methodologies. In this study, we aimed to develop a QSAR model to predict comedogenic potential of cosmetic ingredients by using different machine learning algorithms and types of molecular descriptors. The dataset consists of 121 cosmetic ingredients including such as fatty acids, fatty alcohols and their derivatives and pigments tested on rabbit ears was obtained from the literature. 4837 molecular descriptors were calculated via various software. Different machine learning classification algorithms were used in the modelling studies with WEKA software. The model performance was evaluated by using 10-fold cross validation. All models were compared by the means of classification accuracy, area under the ROC curve, area under the precision-recall curve, MCC, F score, kappa statistic, sensitivity, specificity and the best model was chosen accordingly. The QSAR modelling results for two models are promising for comedogenicity prediction. The random forest models by the means of Mold2 and alvaDesc descriptors gave the successful results with 85.87% and 84.87% accuracy for the cross-validated models and 75.86% and 79.31% accuracy for the test sets. In conclusion, this study is the first step in terms of comedogenicity prediction. In the near future, advances in in silico modelling studies will provide us non-animal based alternative models by regarding animal rights and ethical issues for the safety evaluation of cosmetics.
更多查看译文
关键词
Acne, Machine learning, Cosmetics, QSAR, Comedogenicity, Random forest, In silico
AI 理解论文
溯源树
样例
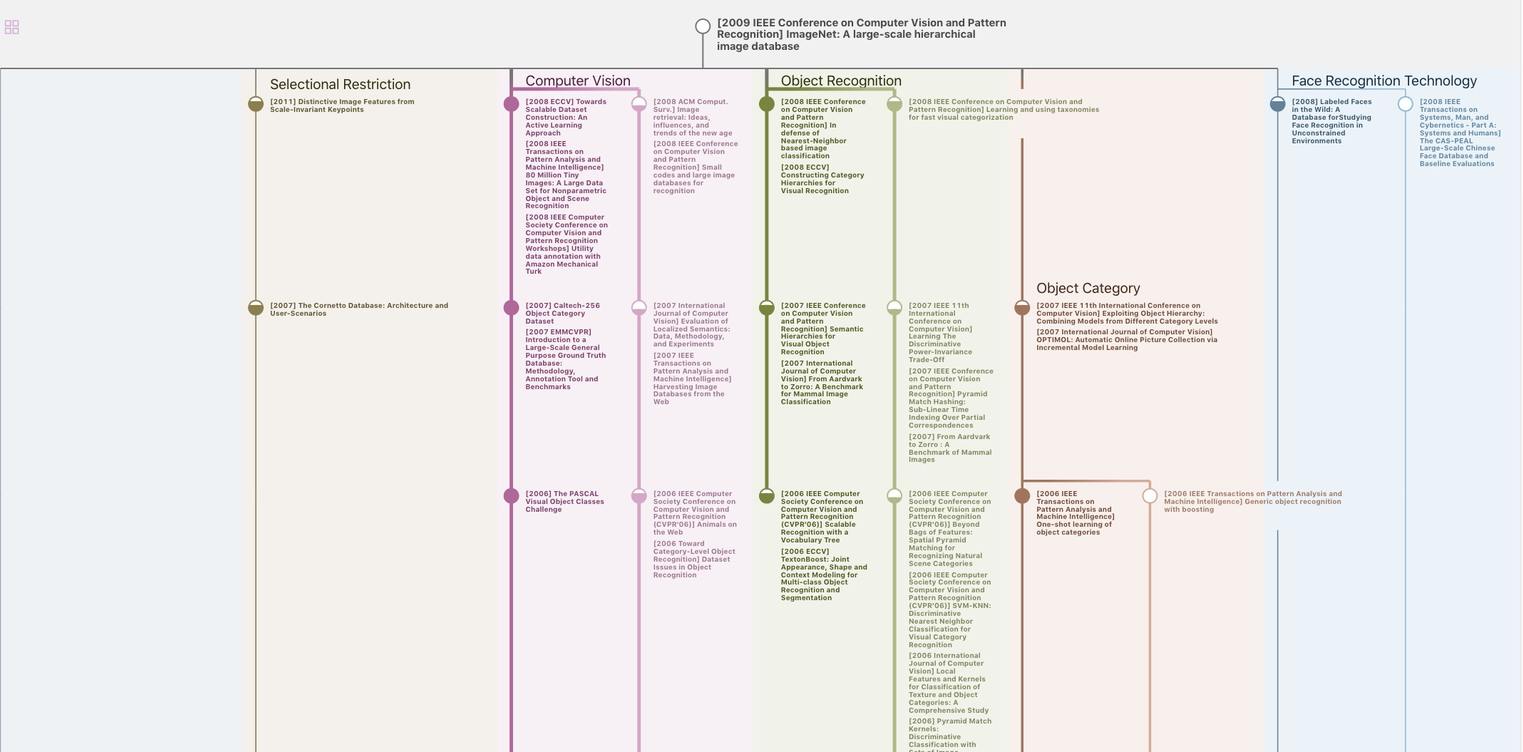
生成溯源树,研究论文发展脉络
Chat Paper
正在生成论文摘要