A Conformal Regressor With Random Forests for Tropical Cyclone Intensity Estimation
IEEE TRANSACTIONS ON GEOSCIENCE AND REMOTE SENSING(2022)
摘要
Tropical cyclone (TC) intensity estimation is critical for disaster forecasting and severe weather warning. In recent years, the performance of various TC intensity estimation models has been gradually enhanced, but the accuracy still needs to be improved. In this article, 71 intensity-related features are extracted from satellite infrared images of TCs. These features are grouped by eye features, circle features, texture features, and time-series features. Using the random forest model as the underlying algorithm of conformal prediction (CP), an intensity applicable CP framework is proposed. On the one hand, the proposed network can achieve point estimation of the TC intensity. On the other hand, it is also possible to realize the intensity interval estimation that satisfies a given significance level. In the experiments, the root mean square error of the point estimation algorithm is 7.86 kt (1 kt approximate to 0.51 m/s), and the performance of the proposed algorithm is better than the comparison algorithms. In addition, interval estimation enriches decision-making information. The experimental results show that the proposed model is a competitive and promising method for estimating the TC intensity.
更多查看译文
关键词
Estimation,Tropical cyclones,Feature extraction,Satellites,Prediction algorithms,Training,Predictive models,Conformal prediction (CP),machine learning,random forests (RFs),statistical learning,tropical cyclones (TCs)
AI 理解论文
溯源树
样例
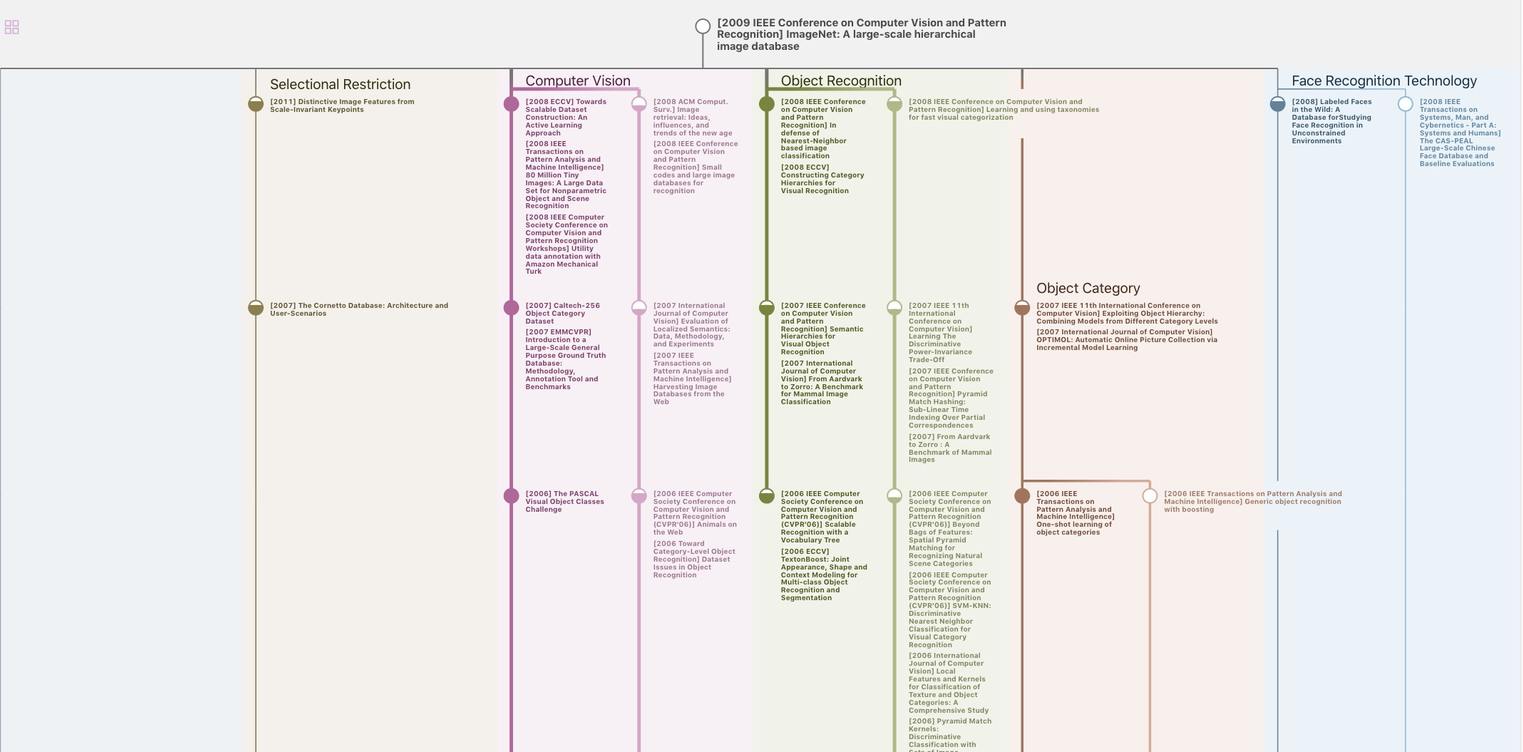
生成溯源树,研究论文发展脉络
Chat Paper
正在生成论文摘要