Privacy-Aware Forecasting of Quality of Service in Mobile Edge Computing
IEEE Transactions on Services Computing(2021)
摘要
We propose a novel privacy-aware Quality of Service (QoS) forecasting approach in the mobile edge environment – Edge-PMAM (
Edge
QoS forecasting with
P
ublic
M
odel and
A
ttention
M
echanism). Edge-PMAM can make real-time, accurate and personalized QoS forecasting on the premise of user privacy preservation. Edge-PMAM comprises a public model for privacy-aware QoS forecasting in an edge region and a private model for personalized QoS forecasting for an individual user. An attention mechanism atop Long Short-Term Memory and an automated edge region division solution are devised to enhance the prediction accuracy of the public and private models. We conduct a series of experiments based on public and self-collected data sets. The results demonstrate that our approach can effectively improve forecasting performance and protect user privacy.
更多查看译文
关键词
Mobile edge computing,joint training,independent learning,privacy-aware forecasting
AI 理解论文
溯源树
样例
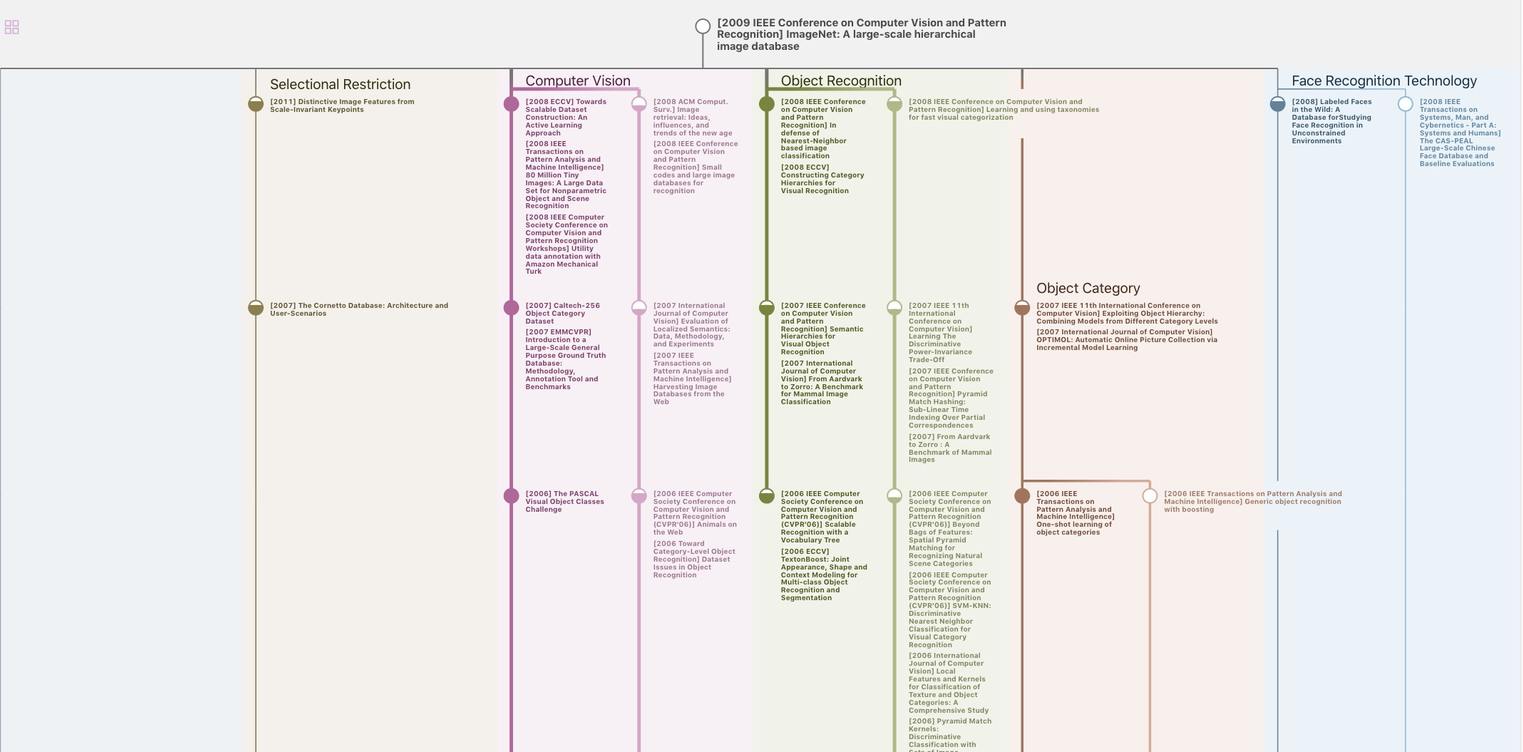
生成溯源树,研究论文发展脉络
Chat Paper
正在生成论文摘要