A Complete Software Stack for IoT Time-Series Analysis that Combines Semantics and Machine Learning—Lessons Learned from the Dyversify Project
Applied sciences(2021)
摘要
Companies are increasingly gathering and analyzing time-series data, driven by the rising number of IoT devices. Many works in literature describe analysis systems built using either data-driven or semantic (knowledge-driven) techniques. However, little to no works describe hybrid combinations of these two. Dyversify, a collaborative project between industry and academia, investigated how event and anomaly detection can be performed on time-series data in such a hybrid setting. We built a proof-of-concept analysis platform, using a microservice architecture to ensure scalability and fault-tolerance. The platform comprises time-series ingestion, long term storage, data semantification, event detection using data-driven and semantic techniques, dynamic visualization, and user feedback. In this work, we describe the system architecture of this hybrid analysis platform and give an overview of the different components and their interactions. As such, the main contribution of this work is an experience report with challenges faced and lessons learned.
更多查看译文
关键词
time series, data analytics, machine learning, semantic web, reasoning, microservice architecture
AI 理解论文
溯源树
样例
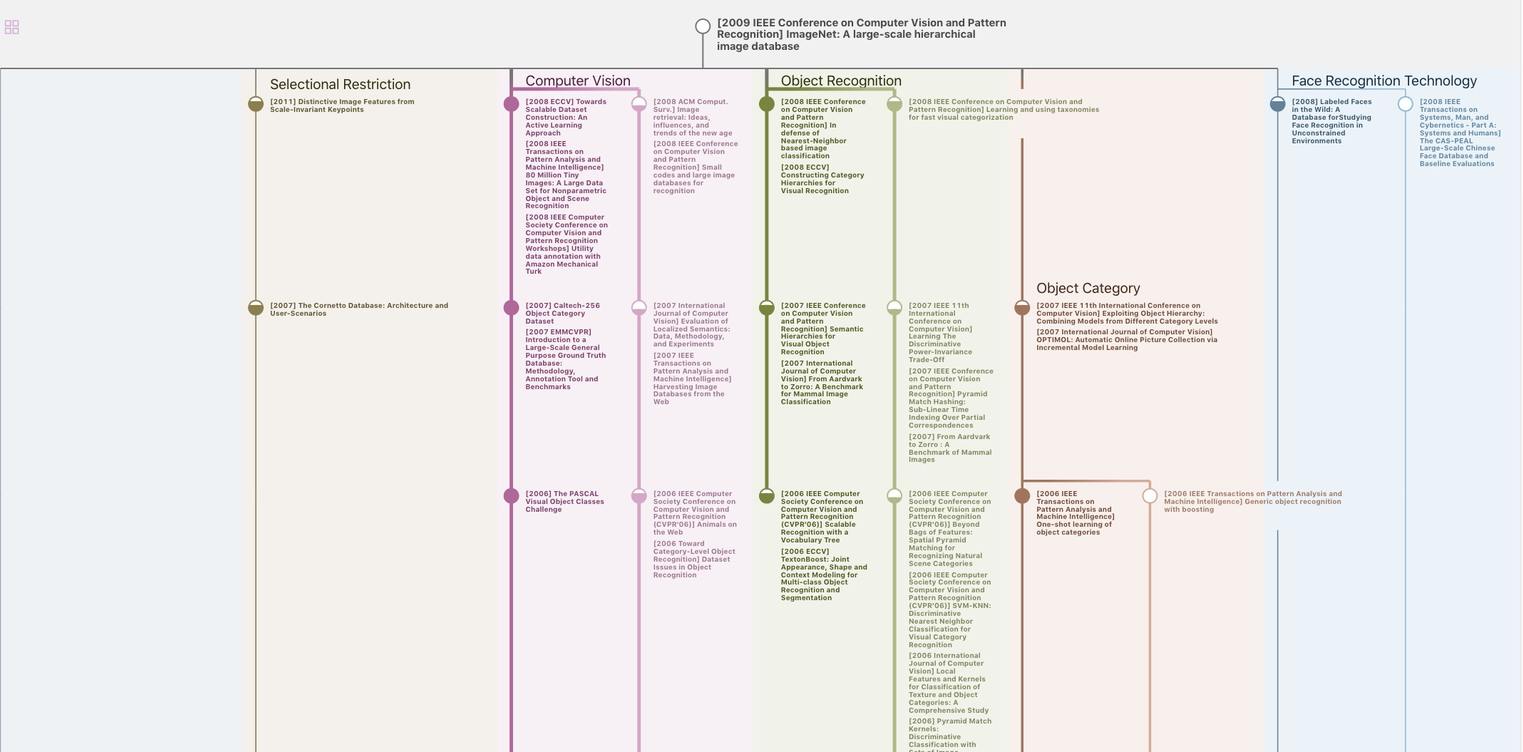
生成溯源树,研究论文发展脉络
Chat Paper
正在生成论文摘要