Robust Least Squares Twin Support Vector Regression With Adaptive FOA and PSO for Short-Term Traffic Flow Prediction
IEEE Transactions on Intelligent Transportation Systems(2022)
摘要
Accurate short-term traffic flow prediction plays an important role in the field of modern Intelligent Transportation Systems. Since various uncontrollable factors (
e.g.
weather, traffic jams or accidents), collected traffic data inevitably contain outliers. This makes it challenge to achieve satisfactory results for traffic flow prediction. Least Squares Twin Support Vector Regression (LSTSVR) has been shown to provide a powerful potential in nonlinear prediction problems. This is especially true when using appropriate heuristic algorithms to determine the parameters of nonlinear LSTSVR. In view of this, a novel LSTSVR model based on the robust
$\text{L}_{2,\mathrm {p}}$
-norm (
$0< p\le 2$
) distance is proposed to alleviate the negative effect of traffic data with outliers, called PLSTSVR. An iterative algorithm is designed to solve the optimization problem of PLSTSVR, which has great potential for solving other relevant optimization problems. To search the parameters of constructed PLSTSVR, this paper constructs two traffic flow prediction models based on PLSTSVR and heuristic algorithms (Fruit Fly Optimization Algorithm and Particle Swarm Optimization), called PLSTSVR-FOA and PLSTSVR-PSO. Extensive experiments demonstrate that the constructed models are more effective and robust than other competing models in various experimental settings.
更多查看译文
关键词
Adaptive PLSTSVR-FOA model,adaptive PLSTSVR-PSO model,least squares twin support vector regression,robust PLSTSVR,short-term traffic flow prediction
AI 理解论文
溯源树
样例
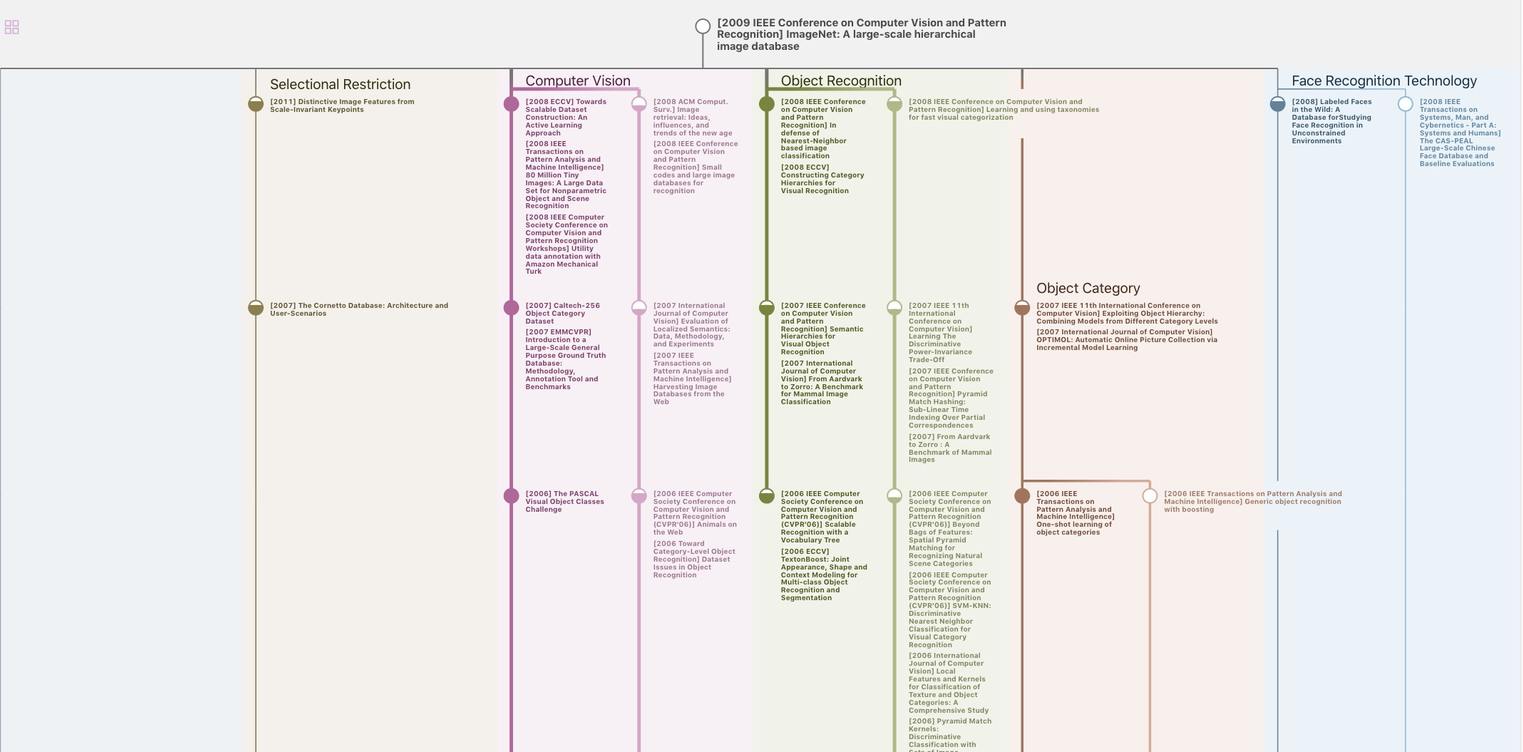
生成溯源树,研究论文发展脉络
Chat Paper
正在生成论文摘要