A Multi-Market Trading Framework for Low-Latency Service Provision at the Edge of Networks
IEEE Transactions on Services Computing(2021)
摘要
Along with the Ultra-Reliable and Low-Latency Communications (URLLC) of 5G, edge computing enables many potential low-latency IoT applications and has recently gained widespread attention. Addressing edge computing’s economic issues is critical as we need to motivate edge devices as resource providers to devote their computing resources to the service. There are multiple users and resource providers in most edge computing service scenarios. The communication delays between users and providers are not the same since their physical distances are different. However, most existing works on edge computing regarding network economics adopt single market models that do not consider the influence of communication delay. Some researchers advocate using multi-market models to overcome this issue by grouping users who connect to the same access point or base station and letting each host its auction for resource trading. However, due to the high cost of deploying 5G ultra-dense small cells, barely a network operator can reach full network coverage. Users cannot rely on a single network, but these models do not allow resource trading between different groups. Thus, this article proposes a novel multi-market trading (MMT) framework to address these shortcomings. The framework combines the double auction at each group and a market selection game to enable the resource providers to participate in multiple auctions and analyze their choice of markets. We present the framework’s detailed design and propose a theory-based learning algorithm to approximate each provider’s optimal strategies. Through extensive simulations using real-world datasets of vehicular networks, we show that the proposed framework can improve the social welfare by
$14.45\%$
and
$36.74\%$
, respectively, compared with classic multi-market and single market models.
更多查看译文
关键词
Double auction,edge computing,multi-market,fictitious play
AI 理解论文
溯源树
样例
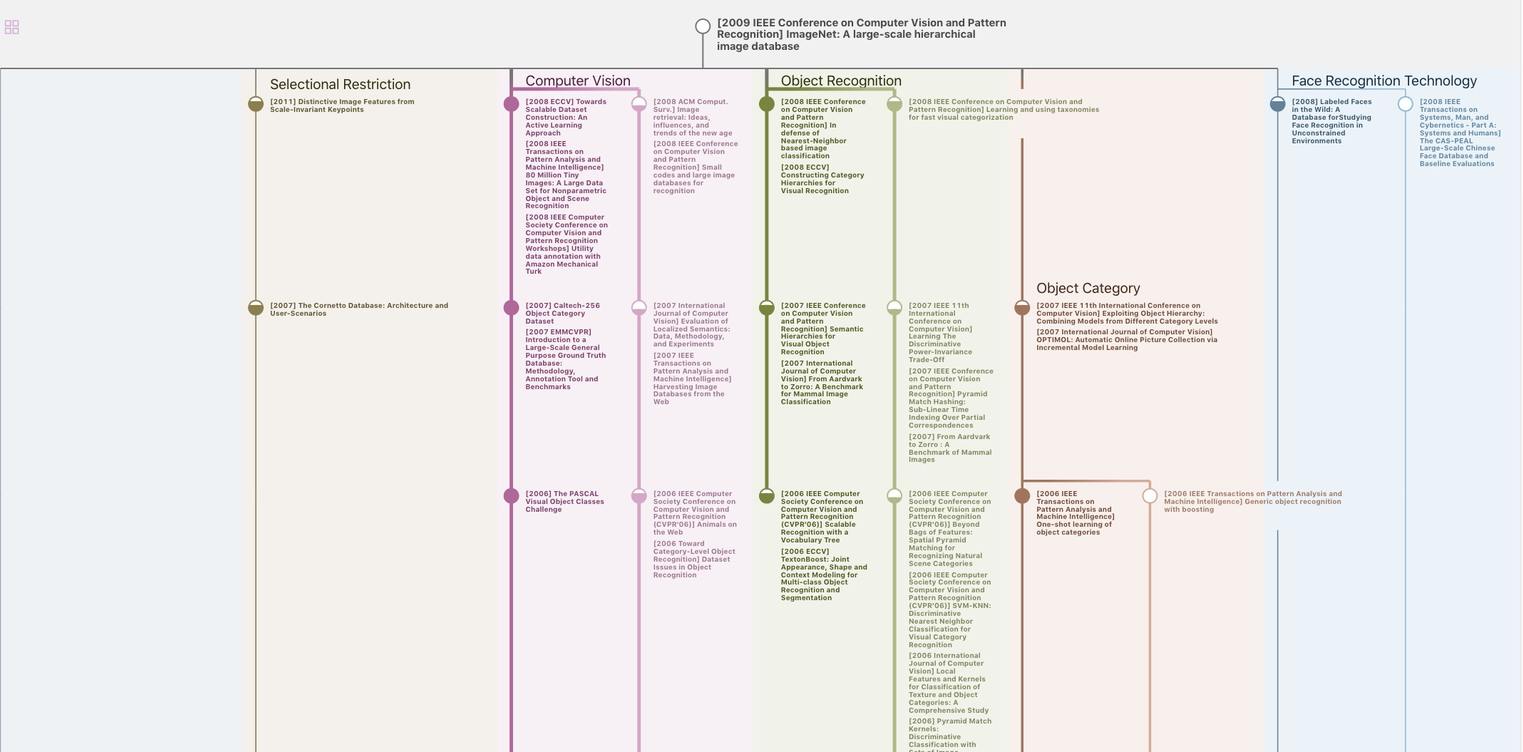
生成溯源树,研究论文发展脉络
Chat Paper
正在生成论文摘要