Synthetic Q-space learning with mixture distribution noise for robust DKI parameter inference
International Forum on Medical Imaging in Asia 2021(2021)
摘要
Diffusional kurtosis imaging (DKI) parameters were conventionally inferred by using signal model fitting. Recent researches by using machine learning showed improvement in parameter inference robustness. Especially the approach of training only with synthetic data, called synthetic Q-space learning (synQSL), has been proved to be have several advantages. In our previous study on synQSL, single level of Rician noise was used for simulating realistic signals in training data, but noise level of clinical data is supposed to be in mixture distribution. In this study, we investigated basic characteristics and usefulness of the mixture distribution noise in training data through experiments with synthetic and real data. Five-layered multi-layer perceptron (MLP) was used for synQSL. The MLP inputs were three values of logarithm of normalized signal decays, that is, we assume The output is single for diffusion coefficient 𝐷 or diffusional kurtosis 𝐾. Two different levels of Rician noise were simulated and were mixed to training dataset. Various levels and mixture ratios of synthetic data for training were examined by using synthetic test data and clinical data to obtain the basic characteristics. Synthetic data experiments showed that noise level matching yields robust inference for 𝐷 and 𝐾 also in mixed distribution of noise level, similar to single noise level training. Clinical data experiments showed that training with mixed noise level yielded less outliers (out-of-range values: 𝐾 2) than single noise level training. Thus, the synQSL with mixture distribution noise has potential to improve robustness for DKI parameter inference in clinical data.
更多查看译文
AI 理解论文
溯源树
样例
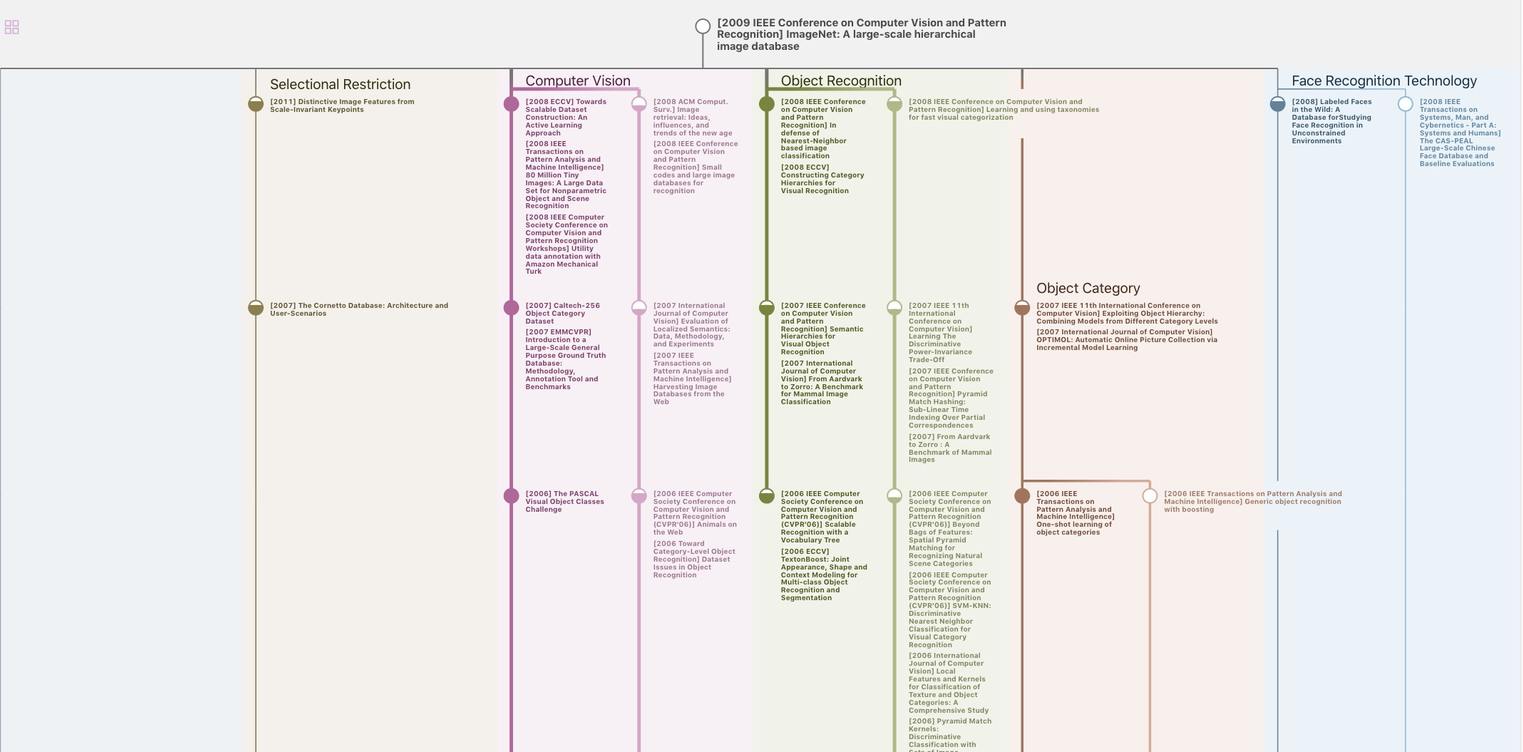
生成溯源树,研究论文发展脉络
Chat Paper
正在生成论文摘要