Marginalized Graph Self-Representation for Unsupervised Hyperspectral Band Selection
IEEE TRANSACTIONS ON GEOSCIENCE AND REMOTE SENSING(2022)
摘要
Unsupervised band selection is an essential step in preprocessing hyperspectral images (HSIs) to select informative bands. Most existing methods exploit the spatial information from the entire HSI while ignoring the difference between diverse homogeneous regions. Moreover, traditional methods utilize the limited size of data for model training that may result in degraded generalization performance. In this article, we propose a marginalized graph self-representation (MGSR) method for unsupervised hyperspectral band selection. To explore the spatial information from diverse homogenous regions, MGSR generates the segmentations of an HSI by superpixel segmentation and records the relationships between adjacent pixels of the same segmentation in a structural graph. Meanwhile, to improve the generalization and robustness, infinite corrupted samples are obtained from the original pixels by introducing noises in spectral bands for model training. To solve the proposed formulation, we design an alternating optimization algorithm to marginalize out the corruption and search for the optimal solution. Experimental studies on HSI datasets demonstrate the effectiveness of the proposed MGSR and the superiority over the state-of-the-art methods. The source code is available at https://github.com/ZhangYongshan/MGSR.
更多查看译文
关键词
Feature extraction,Hyperspectral imaging,Principal component analysis,Image segmentation,Data models,Training,Task analysis,Graph convolution,hyperspectral band selection,marginalized corruption,self-representation (SR),unsupervised learning
AI 理解论文
溯源树
样例
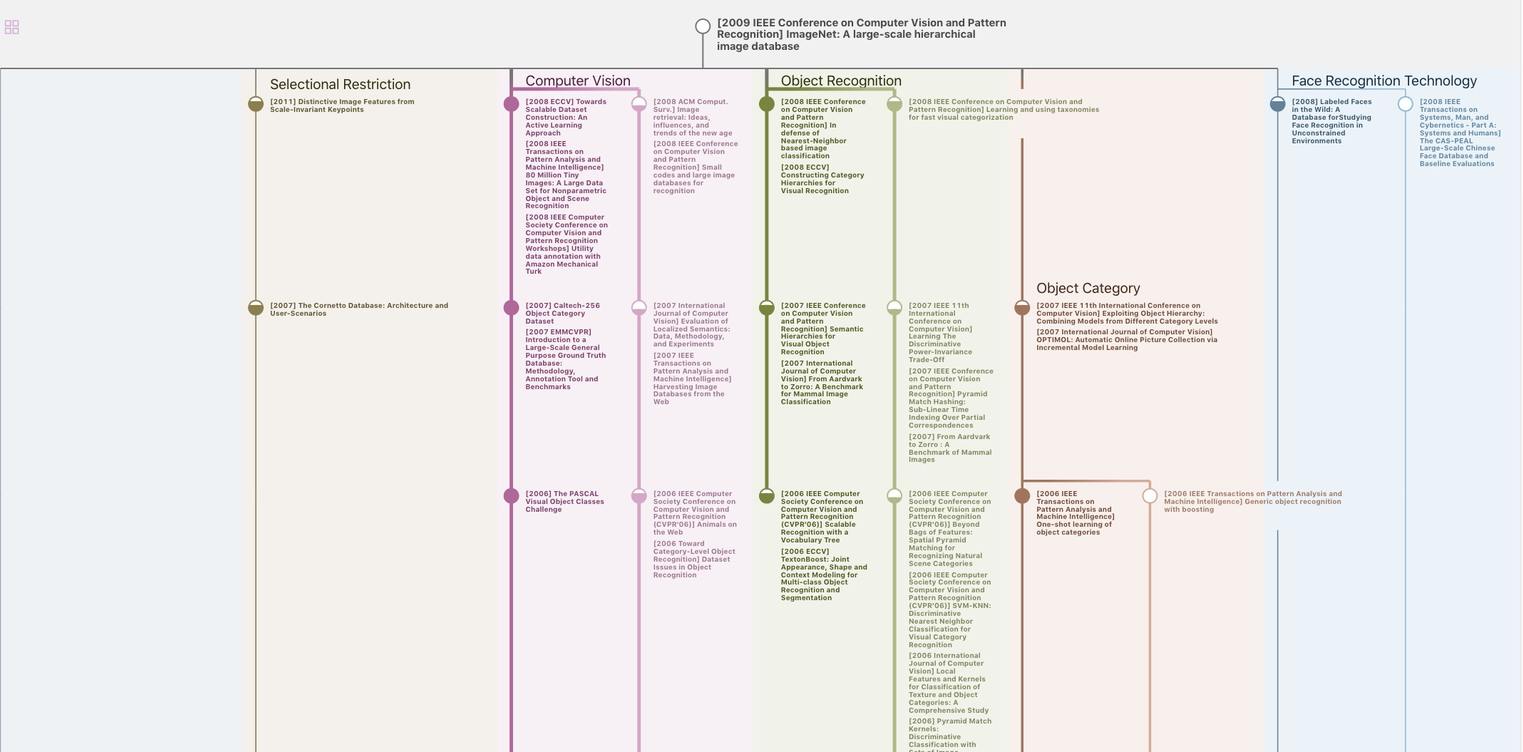
生成溯源树,研究论文发展脉络
Chat Paper
正在生成论文摘要