The impact of label noise on the classification models for hyperspectral images
Image Processing and Communications(2021)
摘要
Supervised classification methods rely heavily on labeled training data. However, errors in the manually labeled data arise inevitably in practice, especially in applications where data labeling is a complex and expensive process, as is often the case in remote sensing. Erroneous labels a ff ect the learning models, deteriorate the classification performances and hinder thereby subsequent image analysis and scene interpretation. In this paper, we analyze the e ff ect of erroneous labels on spectral signatures of landcover classes in remotely sensed hyperspectral images (HSIs). We analyze also statistical distributions of the principal components of HSIs under label noise in order to interpret the deterioration of the classification performance. We compare the behaviour of di ff erent types of classifiers: spectral only and spectral-spatial classifiers based on di ff erent learning models including deep learning. Our analysis reveals which levels of label noise are acceptable for a given tolerance in the classification accuracy and how robust are different learning models in this respect.
更多查看译文
AI 理解论文
溯源树
样例
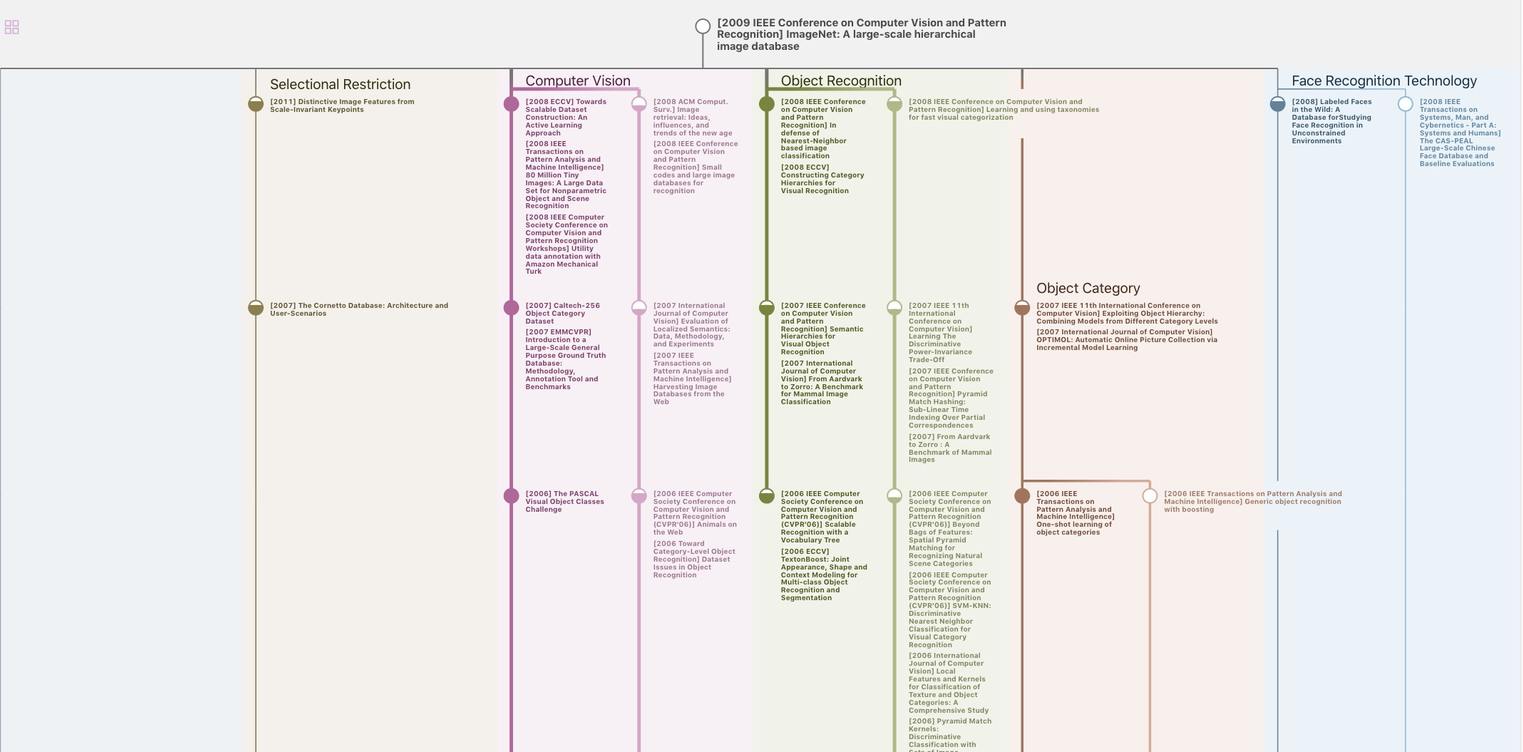
生成溯源树,研究论文发展脉络
Chat Paper
正在生成论文摘要