Machine Learning for Determining Lateral Flow Device Results in Asymptomatic Population: A Diagnostic Accuracy Study
Social Science Research Network(2021)
摘要
Introduction: Rapid antigen tests, in the form of lateral flow devices (LFD) allow testing of a large population for SARS-CoV-2 rapidly either on an asymptomatic or symptomatic basis and have been demonstrated to reduce transmission when used correctly. Compared to real time quantitative PCR (RT-qPCR), LFD have a different sensitivity range to viral load, and can be used to self-test, which can present challenges for lay users to interpret especially at low viral loads. In order to reduce the variability seen in device interpretation, we designed and tested an AI algorithm based on machine learning.
Methods: A machine learning (ML) algorithm was trained on a combination of artificially hybridised LFDs from a range of test conditions and viral dilutions and real-world LFD data linked to RT-qPCR results. Two sub-studies were carried out. In the first, participants were recruited from asymptomatic test sites (ATS) in the community and in the second from health care workers undertaking self-testing using LFDs at home. A panel of trained clinicians were used to resolve discrepancies between the algorithm and the ATS/self-report result. Standard estimates of diagnostic precision were applied, comparing ATS and self-report results to the diagnostic algorithm, with and without resolution of discrepancies.
Results: In total, 115,316 images were returned by the ATS and self-test users (59,450 ATS, 50,999 self-test). In the ATS sub study, use of the AI algorithm increased sensitivity compared to a human reader from 92.08% to 97.6% (95% CI 93.20-99.51%) and specificity from 99.85% to 99.99% (95% CI -99.90%), correctly identifying another 30 positive images compared to the submitted result. In the self-read sub study, sensitivity increased from 16.00% to 100% (95% CI 90.2-100%), predominantly due to detection of faint bands representing low viral loads, and specificity from 99.15% to 99.40% (95% CI 99.33-99.47%), with an additional 32 positive images correctly identified compared to the submitted result.
Conclusion: An ML-based classifier of LFD results outperforms human reads in asymptomatic testing sites and self-reading.
Clinical Trial Registration Details: This study is registered with the ISRCTN registry (reference number 39894, https://www.isrctn.com/ISRCTN30075312.
Funding Information: The study was funded by NHS Track and Trace. ADB is funded by an CRUK Advanced Clinician Scientist Award (ref C31641/A23923).
Declaration of Interests: There are no competing interests.
Ethics Approval Statement: Ethical approval was sought from the Public Health England Research Ethics committee, who confirmed that the study was exempt from ethical approval, as the study was purely observational with the AI result not being returned to the user.
更多查看译文
AI 理解论文
溯源树
样例
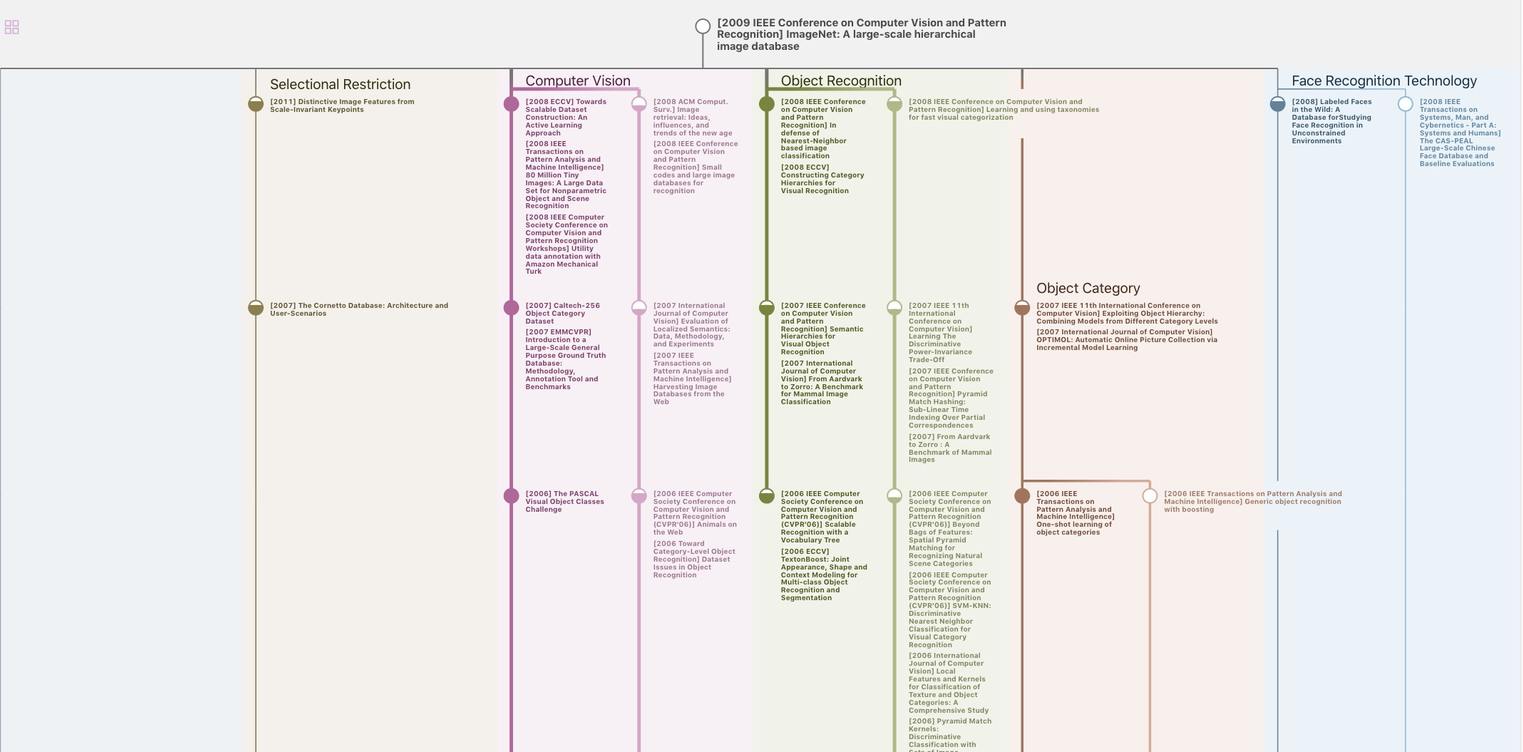
生成溯源树,研究论文发展脉络
Chat Paper
正在生成论文摘要