SAR Target Recognition With Modified Convolutional Random Vector Functional Link Network
IEEE GEOSCIENCE AND REMOTE SENSING LETTERS(2022)
摘要
Deep learning models have achieved remarkable performance in synthetic aperture radar (SAR) target recognition. However, the accuracy of these methods is sensitive to the hyper-parameters and the traditional backpropagation is time consuming. In this letter, we proposed a modified convolutional random vector functional link (IntCRVFL) network for SAR target recognition, which can simplify the SAR target recognition system. The CRVFL network consists of a convolutional neural network and an RVFL network. First, the fixed convolutional layers with randomly initialized parameters extract SAR image features and then the RVFL network performs target recognition. Especially, inspired by hyperdimensional computing, the activations of the hidden layer are obtained through a new encoding manner. Besides, only the connections between hidden and output layers need to train by a closed-form solution for the ultimately precise target recognition. The experimental results on the moving and stationary target acquisition and recognition (MSTAR) dataset demonstrate the proposed IntCRVFL network can obtain a satisfying accuracy with a faster speed.
更多查看译文
关键词
Synthetic aperture radar,Target recognition,Convolutional neural networks,Feature extraction,Radar polarimetry,Computer architecture,Training,Convolutional neural network (CNN),hyperdimensional computing (HDC),random vector functional link (RVFL),synthetic aperture radar (SAR),target recognition
AI 理解论文
溯源树
样例
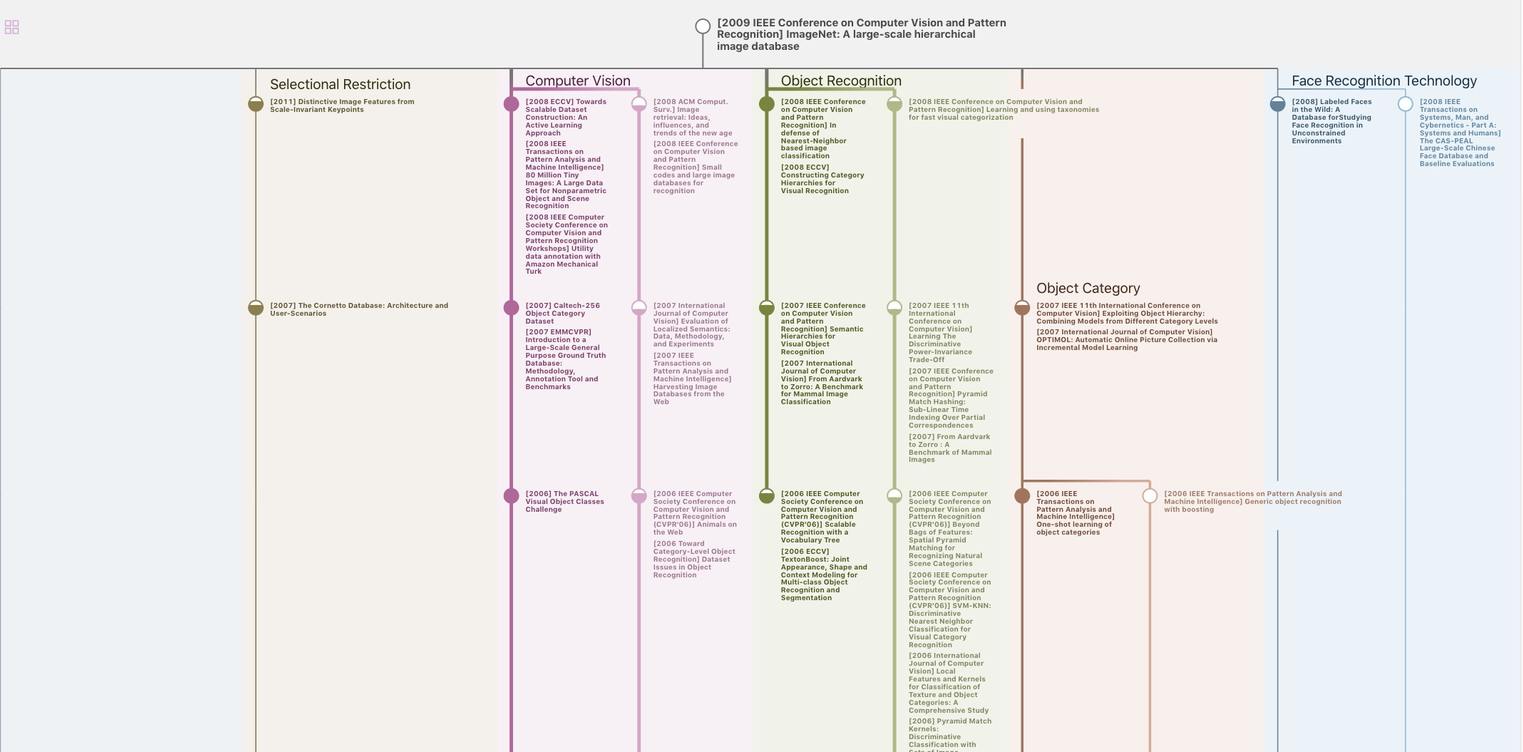
生成溯源树,研究论文发展脉络
Chat Paper
正在生成论文摘要