Achieving Clinical Automation in Emergency Medicine with Machine Learning Medical Directives
Social Science Research Network(2021)
摘要
Background: Increased patient volumes, wait-times, and long lengths of stay in emergency departments (EDs) are common healthcare challenges associated with poor patient outcomes. We propose a practical machine learning (ML) approach to autonomously ordering downstream testing immediately after patient triage to improve ED efficiency. In doing so, test results can be available to physicians during their initial encounter accelerating clinical decision making.
Methods: We created a novel clinical workflow for ED patients through the development of machine learning medical directive (MLMD) models. Logistic regression, random forest, and deep neural network models were trained using retrospective electronic health record (EHR) data collected from the Hospital for Sick Children, a tertiary academic care center in Toronto, Canada. All patients 0-18 years of age presenting to the ED from July 2018 to June 2019 (77,219 total patient visits) were included. MLMD models predict the need for urinary dipstick testing, electrocardiograms, abdominal ultrasounds, testicular ultrasounds, bilirubin testing, and forearm radiographs. Models were optimized to limit the total number of false positives and achieve high positive predictive values (PPVs). Model bias and explainability assessments were also performed to add transparency and trust to our MLMD approach.
Findings: MLMD models obtained high area-under-the-receiver-operator curve (0·89-0·99) and PPVs (0·77-0·94). The implementation of MLMD would streamline care for 22·3% of all patient visits, while making test results available earlier by 165 minutes (weighted average) per affected patient. Model explainability assessments demonstrated clinically relevant features having the most impact on predictions with minimal bias present.
Interpretation: Our work demonstrates a practical approach for EDs to achieve clinical automation using MLMDs. When integrated into patient workflows, MLMDs can safely and autonomously order common ED tests early in a patient visit with explainability provided to patients and clinicians.
Funding: Canadian Institute for Health Research, SickKids Foundation
Declaration of Interest: None to declare.
Ethical Approval: Data was collected from an EHR system at the Hospital for Sick Children, a tertiary care hospital in the city of Toronto, Canada, for all patients presenting to the ED from July 2018 to June 2019 (77,219 total patient visits) with Research Ethics Board approval.
更多查看译文
AI 理解论文
溯源树
样例
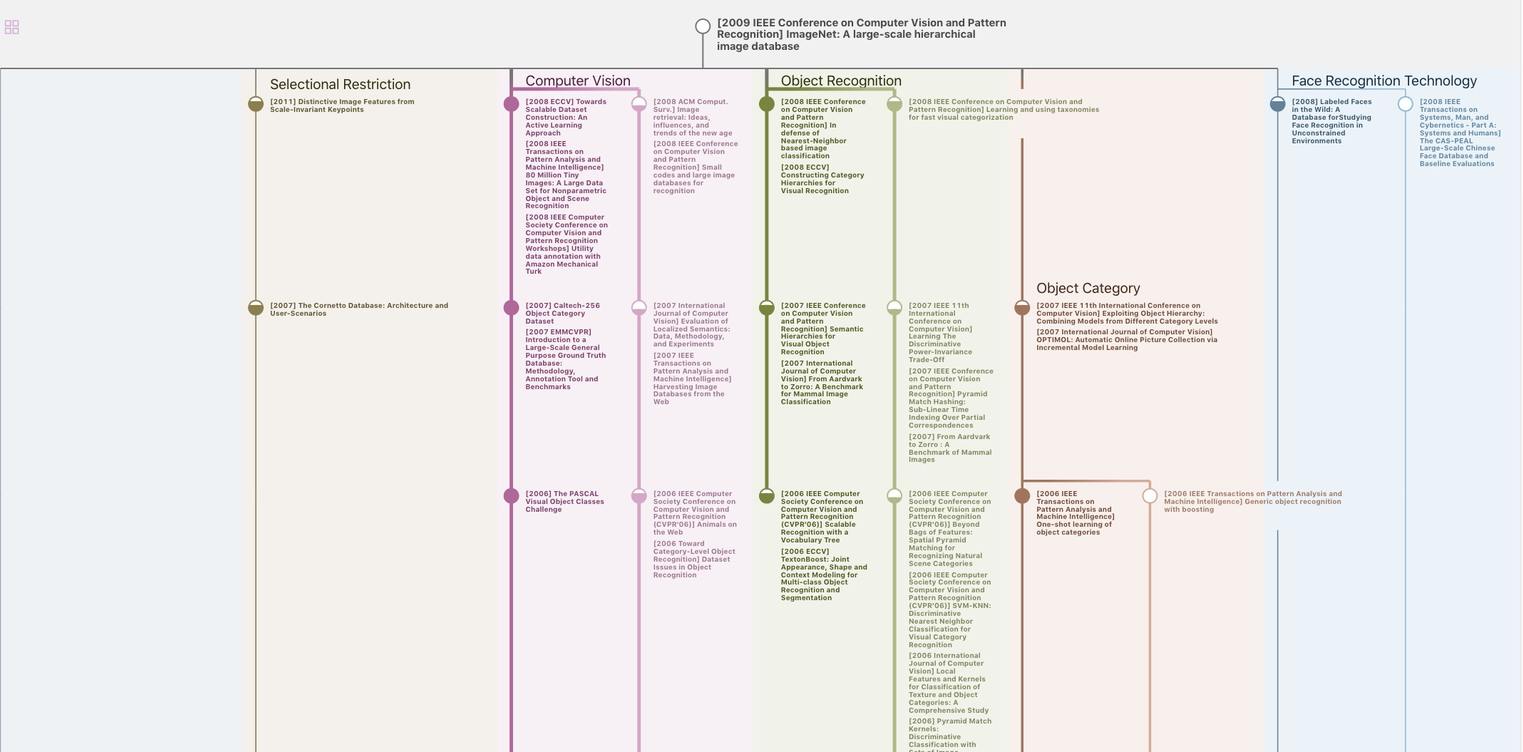
生成溯源树,研究论文发展脉络
Chat Paper
正在生成论文摘要