A probabilistic framework for online structural health monitoring: active learning from machining data streams
Journal of physics(2019)
摘要
A critical issue for data-based engineering is a lack of descriptive labels for the measured data. For many engineering systems, these labels are costly and/or impractical to obtain, and as a result, conventional supervised learning is not feasible. This paper suggests a probabilistic framework for the investigation and labelling of engineering datasets; specifically, acoustic emission data streams recorded online from a turning machine. Two alternative probabilistic measures are suggested to select the most informative observations. During machining operations, these data would then be investigated and annotated by an engineer, in order to maximise the classification performance of a statistical model used to predict tool wear.
更多查看译文
AI 理解论文
溯源树
样例
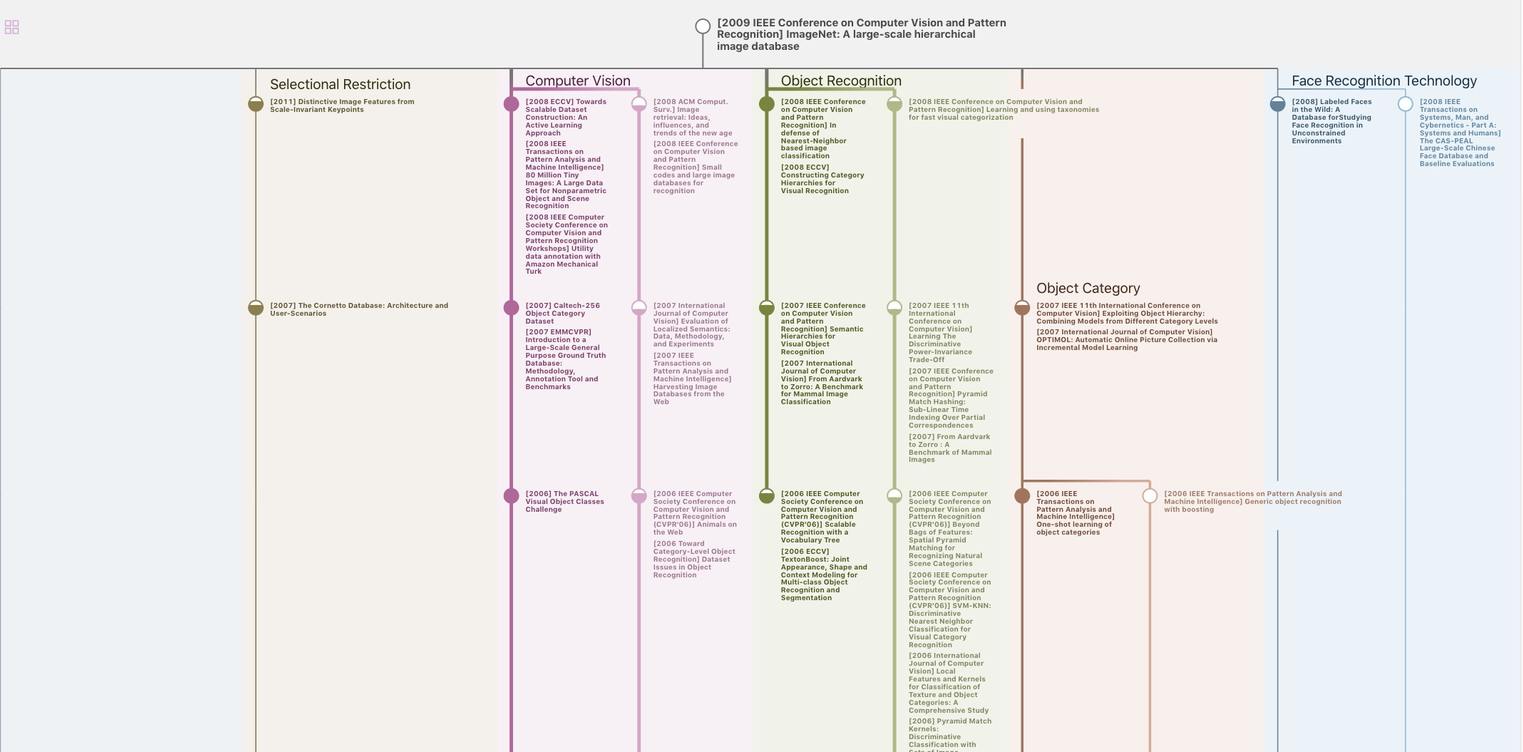
生成溯源树,研究论文发展脉络
Chat Paper
正在生成论文摘要