Identification of Metabolomic Biomarker using Multiple Statistical Techniques and Recursive Feature Elimination
2019 International Conference on Computer, Communication, Chemical, Materials and Electronic Engineering (IC4ME2)(2019)
摘要
Mortality rate of diseases like lung cancer can be decreased significantly by increasing the chance of early diagnosis. Identifying differentially expressed (DE) metabolites may contribute remarkably in this concern, and also in drug design. In the past, several kinds of approaches were attempted to discover biomarkers for diseases. Nonetheless, discovering compact-sized biomarkers while maintaining satisfactory classification performance is still a challenge. Therefore, for further contribution in this sector, we have declared biomarkers from our identified DE metabolites in plasma and serum blood sample of lung cancer. Student’s t-test, Kruskal-Wallis and Mann-Whitney-Wilcoxon test were applied to distinguish the DE metabolites. Cluster heatmap plot and fold change values were used to differentiate between up and down-regulated metabolites. Finally, RFE method was used to order the metabolites and select biomarkers from them. To assess the performance with our DE metabolites or biomarkers, SVM classifier was utilized. We found 28 DE metabolites from plasma dataset and 13 from serum (p-value $\lt 0.05)$. In the end, 8 metabolites were selected from plasma sample and 5 were selected from serum sample as the metabolomic biomarkers. The relevant files and codes of our work can be found at https://github.com/Zeronfinity/LungCancerBiomarkerRFE.
更多查看译文
关键词
Differentially Expressed Metabolites,Biomarkers,T-Test,Kruskal-Wallis,Mann-Whitney-Wilcoxon,Fold Change,Cluster Heatmap,SVM,RFE
AI 理解论文
溯源树
样例
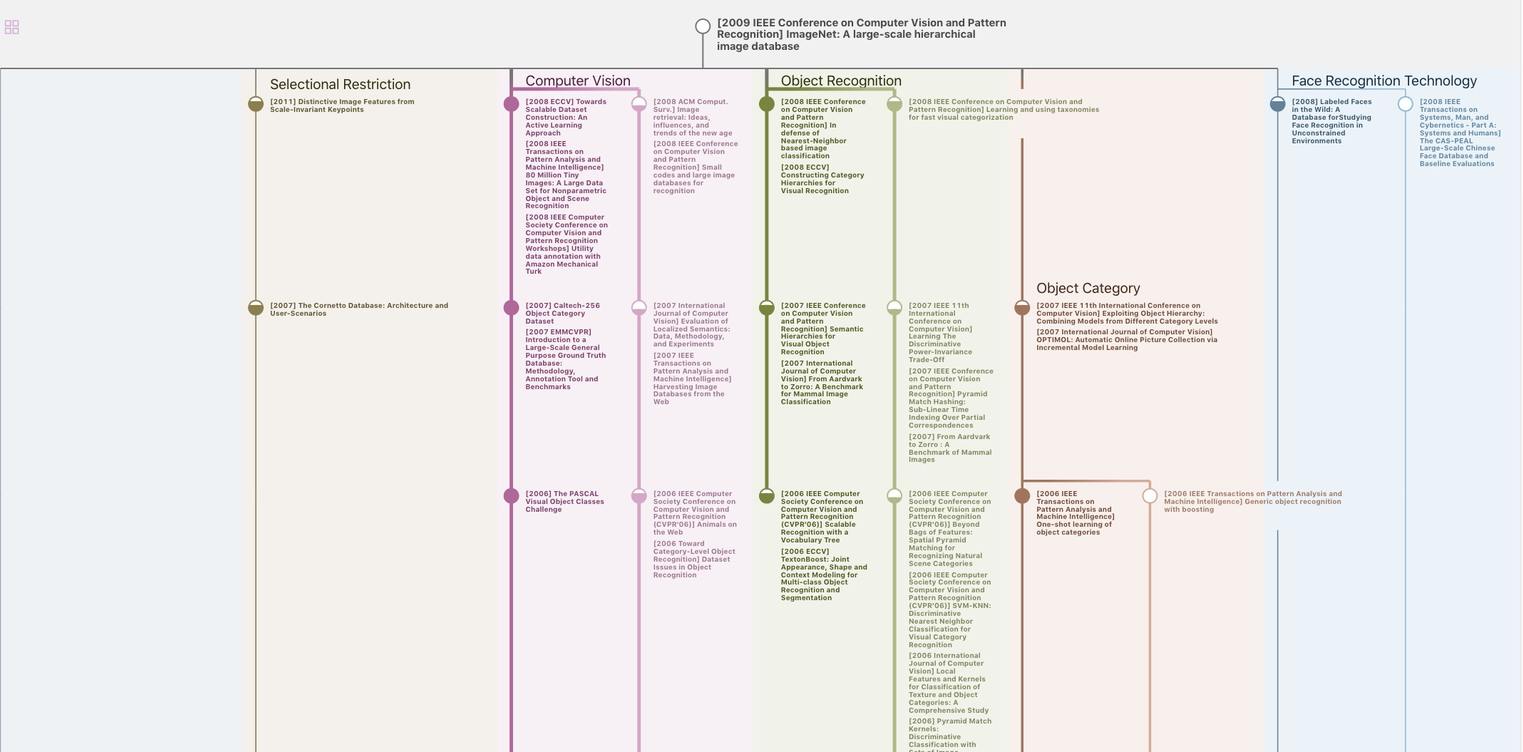
生成溯源树,研究论文发展脉络
Chat Paper
正在生成论文摘要