Field inversion and machine learning strategies for improving RANS modelling in turbomachinery
14th European Conference on Turbomachinery Fluid Dynamics and Thermodynamics(2021)
摘要
The simulation of turbulent flows in turbomachinery is challenging because of the presence of complex phenomena like laminar-turbulent transition and separation which deeply affect the performances of the system. The use of scale-resolving simulations like Large Eddy Simulations and Direct Numerical Simulations offers the possibility to correctly capture important physical phenomena by reducing the amount of modellisation. However, the computational cost of scale-resolving simulations limits their applicability in the design process which requires the evaluation of several geometrical configurations. In this framework, several research efforts have been devoted to the development of machine learning algorithms which can be used to analyze high-fidelity data (from experiments or scale-resolving simulations) and to obtain correcting terms which can be included in Reynolds-averaged Navier-Stokes (RANS) models. Among the different techniques, field inversion represents a promising strategy: the method requires the solution of an optimisation problem which provides a field of corrections which improve the prediction with respect to the baseline RANS model. The goal function of the optimisation problem is based on the error between the predicted numerical results and the available high-fidelity data. The success of this approach is critically linked to the following step: generalisation through machine learning algorithms. This second step is necessary because the field inversion procedure is applied to a well-defined geometry with particular working conditions. In order to generalise the correction to other problems and to get predictive capabilities, the correction field obtained by the field inversion must be analysed in order to identify correlations between the correction field and some flow features which can be used as inputs. While the original works on the field inversion were based on the use of Artificial Neural Networks, several alternative regression strategies are possible. Among them, Random Forests and Gene Expression Programming represent promising approaches. In this work, different regression algorithms are investigated and compared in order to evaluate the obtained correlations in terms of fitting performances and prediction capability. The database used for the training is generated by the application of the field inversion procedure to the T106c low pressure gas turbine cascade and the optimisation process is driven by the errors with respect to the available experimental data.
更多查看译文
AI 理解论文
溯源树
样例
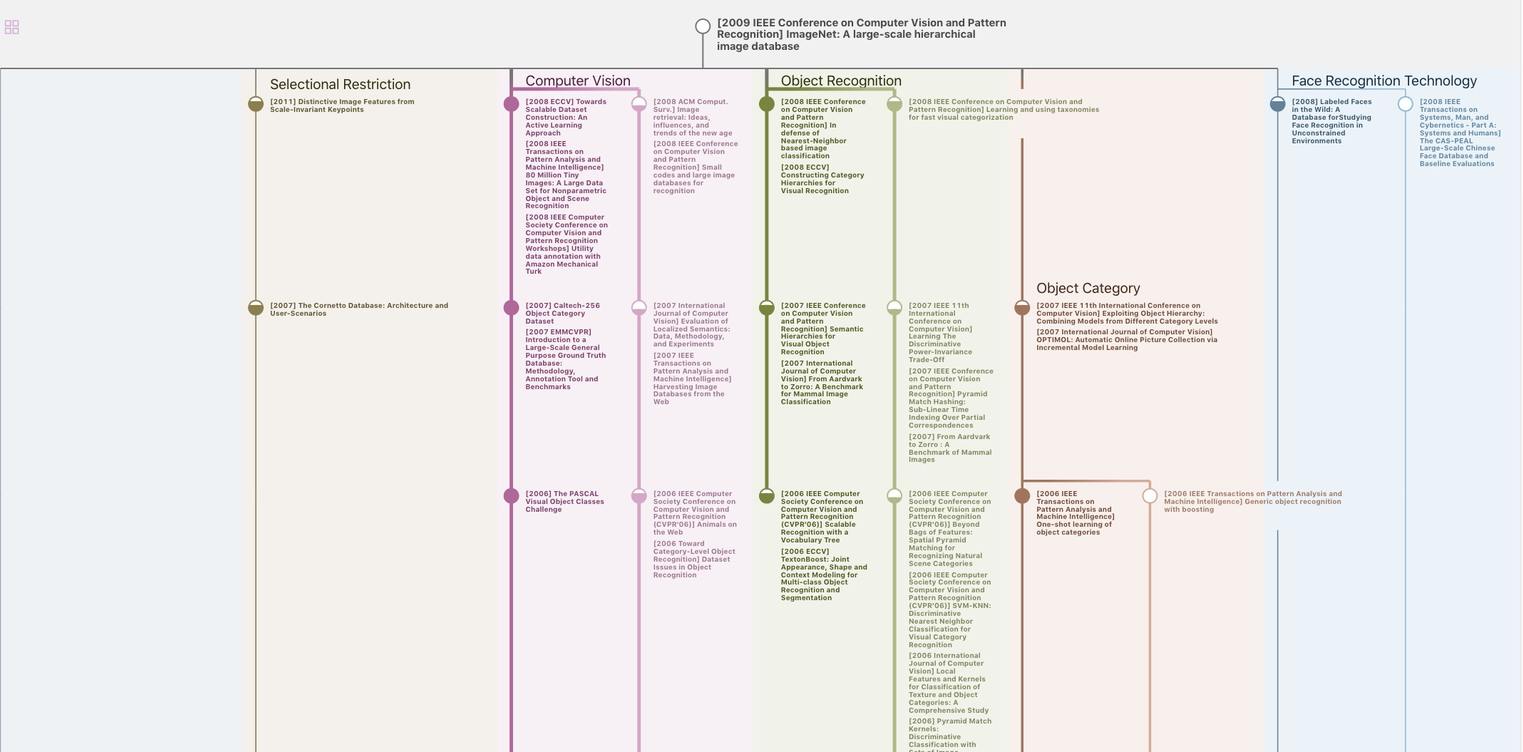
生成溯源树,研究论文发展脉络
Chat Paper
正在生成论文摘要