Active power correction strategies based on deep reinforcement learning—part II: A distributed solution for adaptability
CSEE Journal of Power and Energy Systems(2021)
摘要
This article is the second part of Active Power Correction Strategies Based on Deep Reinforcement Learning. In Part II, we consider the renewable energy scenarios plugged into the large-scale power grid and provide an adaptive algorithmic implementation to maintain power grid stability. Based on the robustness method in Part I, a distributed deep reinforcement learning method is proposed to overcome the influence of the increasing renewable energy penetration. A multi-agent system is implemented in multiple control areas of the power system, which conducts a fully cooperative stochastic game. Based on the Monte Carlo tree search mentioned in Part I, we select practical actions in each sub-control area to search the Nash equilibrium of the game. Based on the QMIX method, a structure of offline centralized training and online distributed execution is proposed to employ better practical actions in the active power correction control. Our proposed method is evaluated in the modified global competition scenario cases of “2020 Learning to Run a Power Network - Neurips Track 2”.
更多查看译文
关键词
Active power correction strategies,distributed deep reinforcement learning,Nash equilibrium,renewable energies,stochastic game
AI 理解论文
溯源树
样例
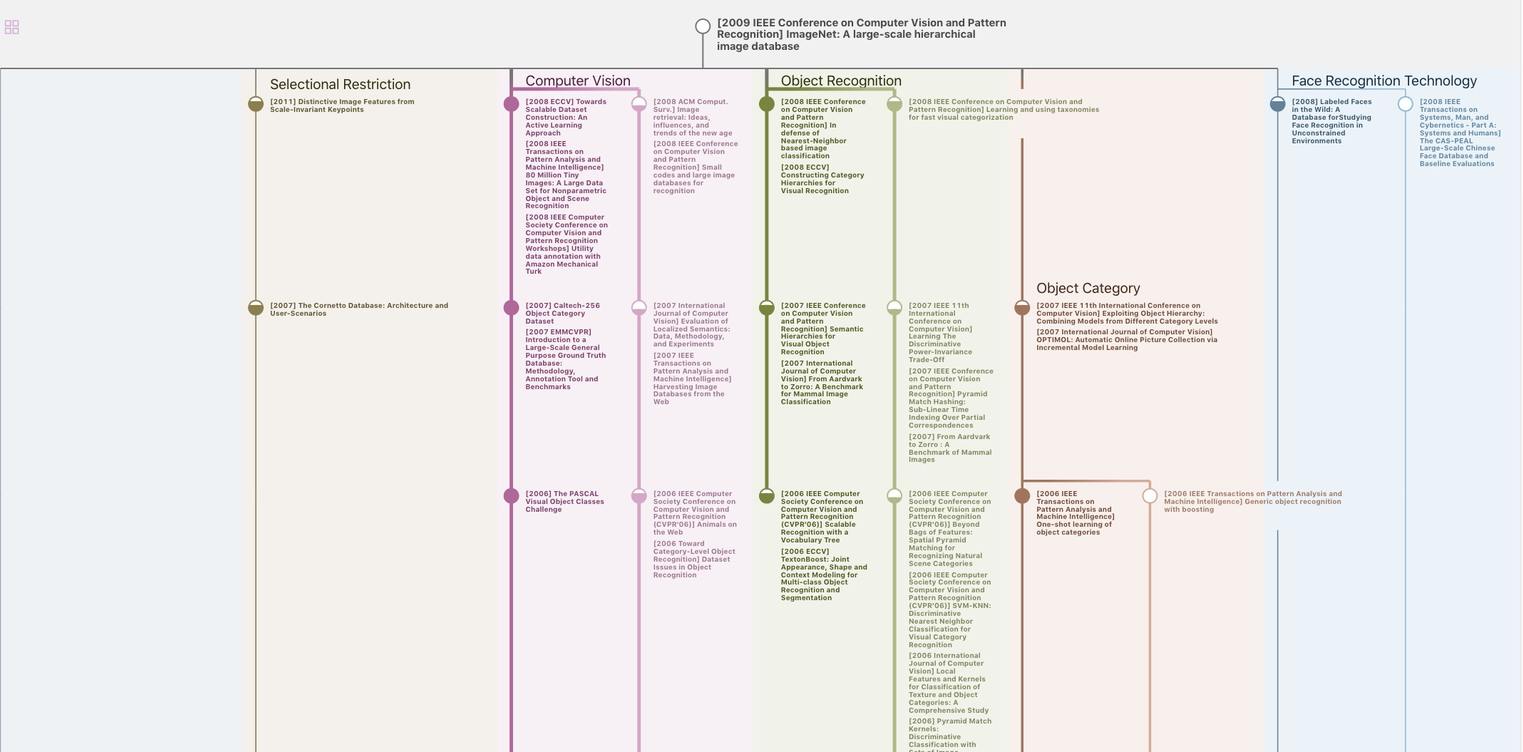
生成溯源树,研究论文发展脉络
Chat Paper
正在生成论文摘要