Enhanced Full-Resolution Residual Network for Image Super-Resolution
chinese control conference(2021)
摘要
Convolutional neural network (CNN) has played a critical role in promoting image super-resolution (SR) performance, and researchers have proposed various models in recent years. Although these models can improve the resolution of the image, the sharpness is not ideal. In this paper, We modify a semantic segmentation network: Full-Resolution Residual Network (FRRN) and propose an Enhanced Full-Resolution Residual Network for Image Super-Resolution (EFRN) model to improve the image clarity. Our network mainly applies a long skip connection to the direct fusion of low-level and high-level features. Besides, we also introduce the attention mechanism into the model to enhance the network’s repair capability. Moreover, experimental results confirm that EFRN has achieved better accuracy and visual effects than state-of-the-art methods.
更多查看译文
关键词
Enhanced Full-Resolution Residual Block,Spatial Attention,Channel Attention,Image Super-Resolution
AI 理解论文
溯源树
样例
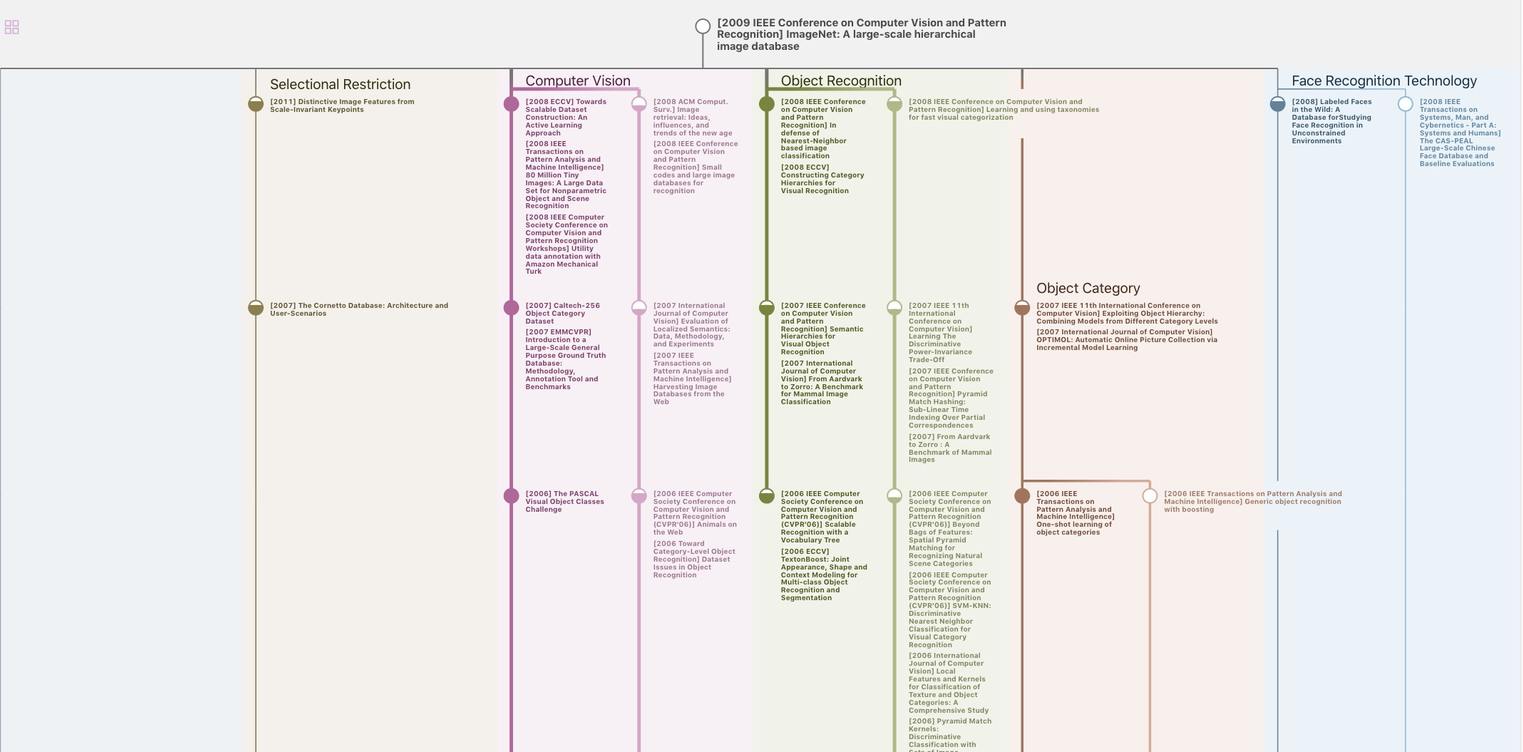
生成溯源树,研究论文发展脉络
Chat Paper
正在生成论文摘要