Transient Electromagnetic Inversion: An ICDE-Trained Kernel Principal Component OSELM Approach
IEEE TRANSACTIONS ON GEOSCIENCE AND REMOTE SENSING(2022)
摘要
The traditional extreme learning machine (ELM) inversion of transient electromagnetic method (TEM) based on random initial weights is known to be inept for its low-computational efficiency and poor generalization performance. To solve these problems, a kernel principal component online sequential extreme learning machine (KPCOSELM) trained by an improved chaotic differential evolution (ICDE) method is proposed. An additional kernel principal component (KPC) layer is used, which reduces the dimension of TEM data and enhances the computational efficiency of online sequential extreme learning machine (OSELM). Moreover, a novel ICDE algorithm is presented for improving the learning ability and generalization performance of OSELM inversion. In the proposed ICDE, the tent chaotic sequence is adopted to enhance the global exploitation ability, and a constraint factor is added to ensure better convergence. The feasibility and effectiveness of the proposed inversion method are evaluated via four groups of experiments. The inversion results of the synthetic and field examples show that the proposed approach outperformed other methods in terms of computational efficiency and prediction accuracy, and realized satisfactory performance in TEM inversion, which provides a new strategy for the application of neural networks in TEM inversion.
更多查看译文
关键词
Neural networks,Training,Conductivity,Principal component analysis,Electromagnetics,Transient analysis,Kernel,Chaotic sequence,differential evolution (DE),Kernel principal component (KPC),online sequential extreme learning machine (OSELM),transient electromagnetic inversion
AI 理解论文
溯源树
样例
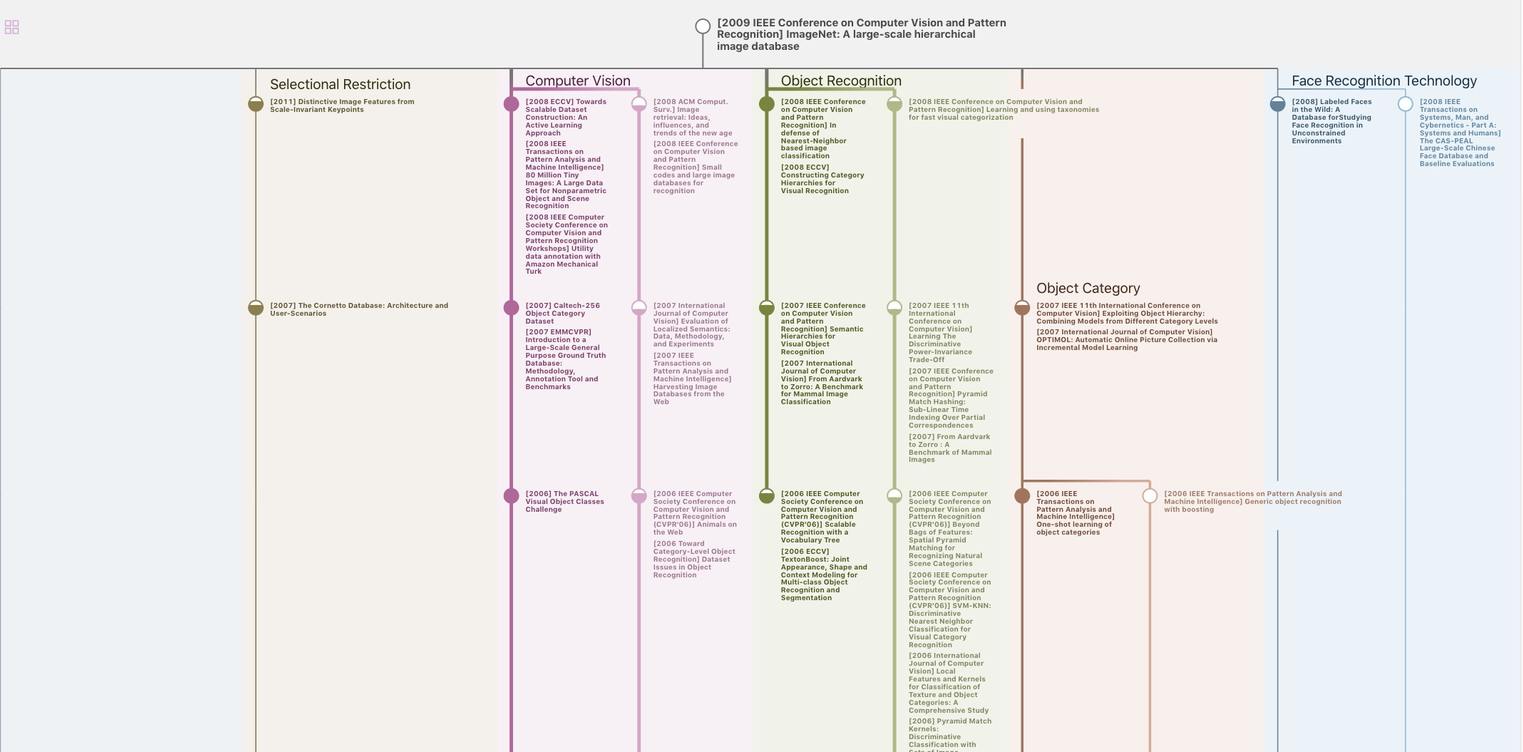
生成溯源树,研究论文发展脉络
Chat Paper
正在生成论文摘要