Machine-Learning Model for Mortality Prediction in Patients with Community-Acquired Pneumonia: Development and Validation Study
Social Science Research Network(2021)
摘要
Background: Pneumonia is the leading infectious cause of mortality globally. Artificial intelligence tools and techniques such as machine learning (ML) are increasingly seen as a suitable manner to increase the prediction capacity of currently available tools in infectious diseases. However, studies evaluating the efficacy of ML methods in enhancing the predictive capacity of existing scores for community-acquired pneumonia (CAP) are limited. We aimed to apply a causal probabilistic network (CPN) model (SepsisFinder) to predict 30-day mortality in patients with CAP. As secondary objective, we pursued validating the ML model using a large cohort of CAP patients.
Methods: This was a derivation-validation retrospective study to test the CPN model in its prediction of 30-day mortality in patients hospitalised with CAP. The derivation cohort comprised all consecutive adults with CAP admitted between 2003 and 2016 to a university hospital in Barcelona, Spain. For the validation cohort, we included all consecutive patients with CAP admitted to a university hospital in Valencia, Spain between 2012 and 2018.
Findings: The derivation cohort comprised 4,531 patients whilst the validation cohort had 1,034 patients. In the derivation cohort, the AUC of SepsisFinder, CURB-65, SOFA, PSI and qSOFA were 0.801, 0.759, 0.671, 0.799 and 0.642, respectively, for 30-day mortality prediction. In the validation study, the AUC of SepsisFinder was 0.826, concordantly with the AUC (0.801) in the derivation data (p=0.51). The AUC of SepisFinder was significantly higher than those of CURB-65 (0.764, p=0.03) and qSOFA (0.729, p=0.005). However, it did not differ significantly from PSI (0.830, p=0.92) and SOFA (0.771, p=0.14).
Interpretation: Sepsisfinder shows potential for improving mortality prediction amongst patients with CAP using structured health data. Additional external validation studies should be conducted to support generalisability.
Funding: CIBER de Enfermedades Respiratorias (CIBERES CB06/06/0028), and by 2009 Support to Research Groups of Catalonia 911, IDIBAPS.
Declaration of Interest: Dr Cilloniz is the recipient of the SEPAR fellowship 2018, and a grant from the Fondo de Investigacion Sanitaria (PI19/00207). LW is employed on a consulting basis and is a shareholder of Treat Systems ApS. MM is CEO and a shareholder of Treat Systems ApS. Treat Systems ApS is a manufacturer of medical device software for decision support in the field of infectious diseases and clinical microbiology, including TREAT-Lab. All other authors have nothing to declare.
Ethical Approval: Institutional approval was provided by the Ethics Committee of the University Hospital La Fe of Valencia (XXXXXXX) and the Comite Etic d’Investigacio Clinica of the Hospital Clinic of Barcelona (2011/0219), which waived the need for informed consent. All data set were anonymously analyzed, and the study was performed following current recommendation of the Declaration of Helsinki.
更多查看译文
关键词
artificial intelligence,community-acquired pneumonia,machine learning,mortality prediction,pneumonia
AI 理解论文
溯源树
样例
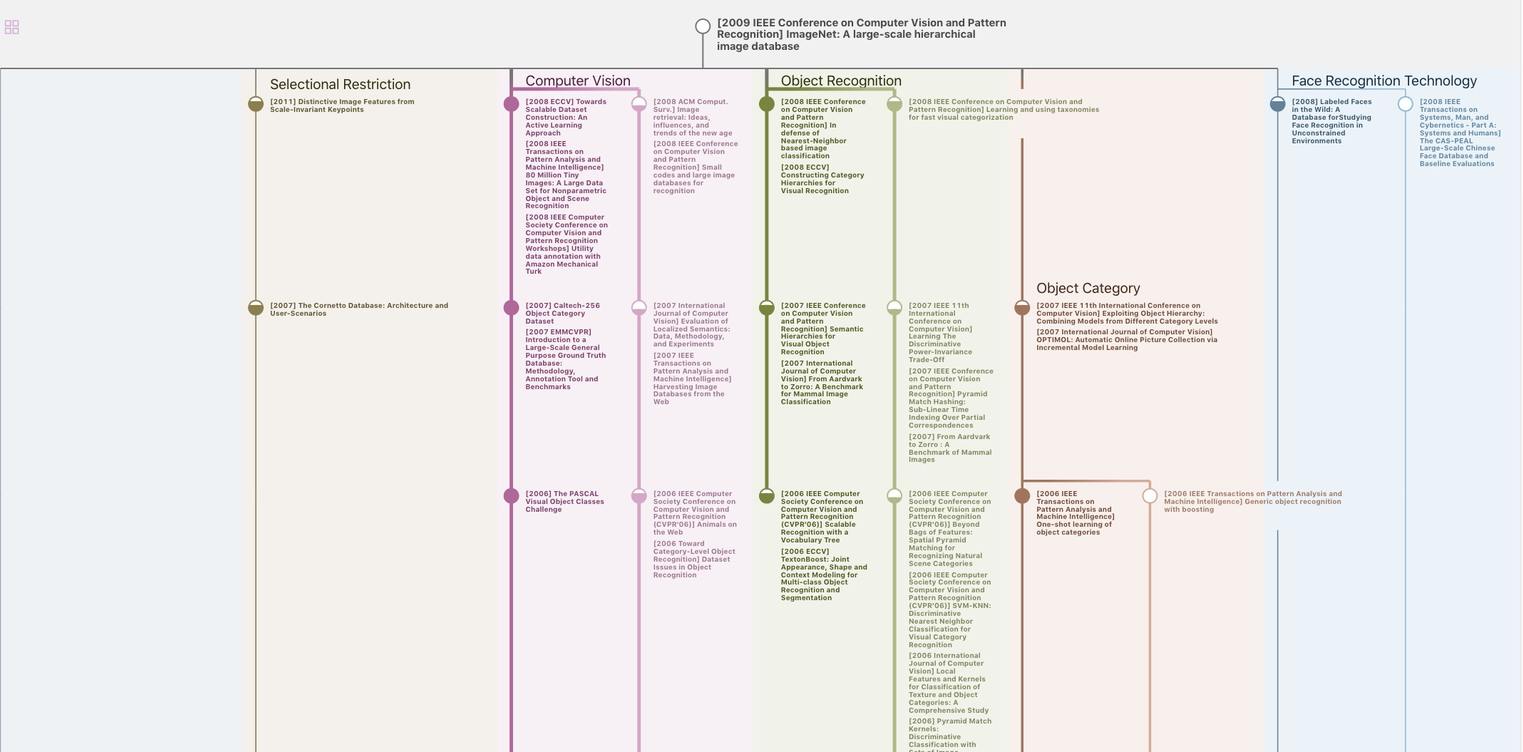
生成溯源树,研究论文发展脉络
Chat Paper
正在生成论文摘要