Large Dynamic Covariance Matrices: Enhancements Based on Intraday Data
Social Science Research Network(2021)
摘要
Multivariate GARCH models do not perform well in large dimensions due to the so-called curse of dimensionality. The recent DCC-NL model of Engle et al. (2019) is able to overcome this curse via nonlinear shrinkage estimation of the unconditional correlation matrix. In this paper, we show how performance can be increased further by using open/high/low/close (OHLC) price data instead of simply using daily returns. A key innovation, for the improved modeling of not only dynamic variances but also of dynamic correlations, is the concept of a regularized return, obtained from a volatility proxy in conjunction with a smoothed sign of the observed return.
更多查看译文
关键词
Dynamic conditional correlations,Intraday data,Markowitz portfolio selection,Multivariate GARCH,Nonlinear shrinkage
AI 理解论文
溯源树
样例
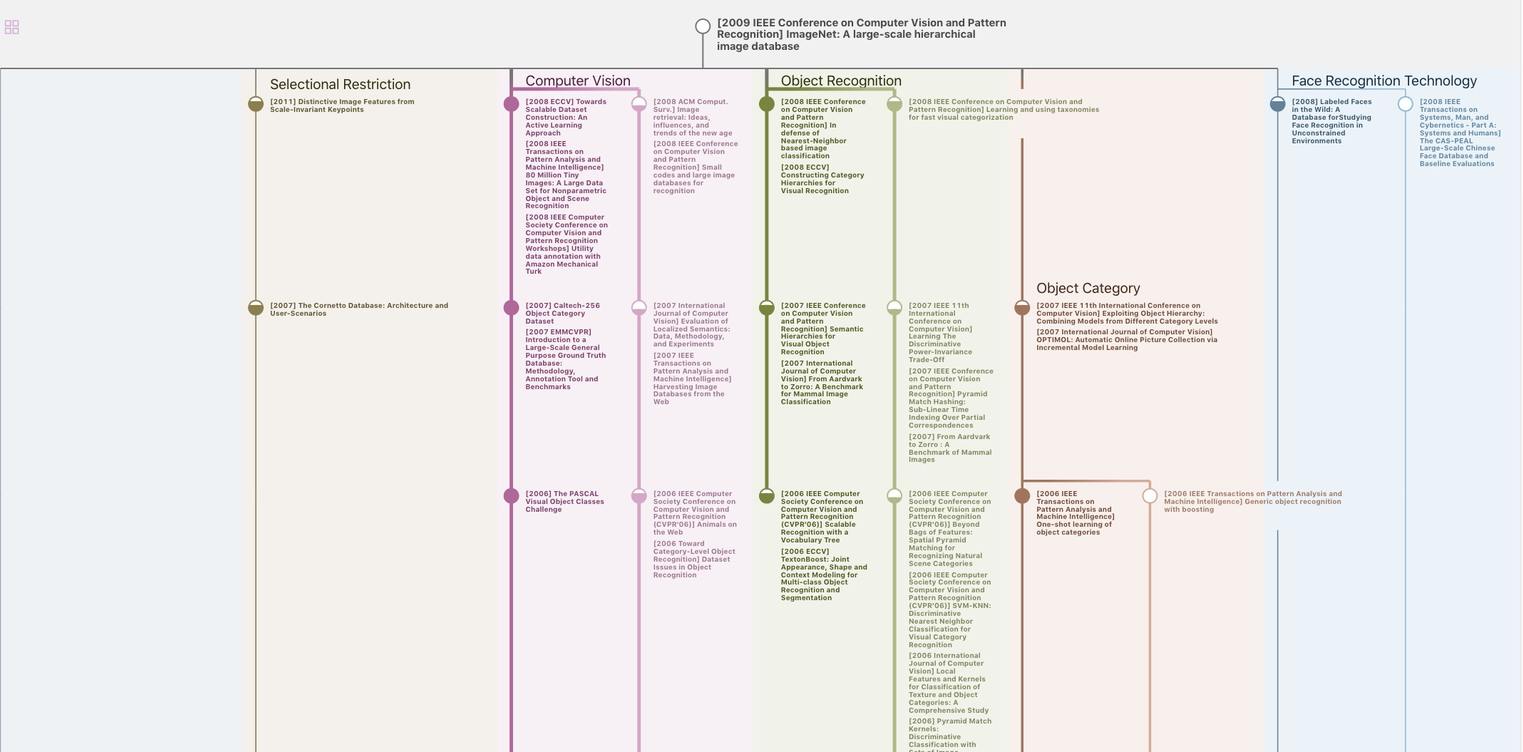
生成溯源树,研究论文发展脉络
Chat Paper
正在生成论文摘要