Memory-augmented meta-learning on meta-path for fast adaptation cold-start recommendation
CONNECTION SCIENCE(2022)
摘要
Personalised recommendation is a difficult problem that has received a lot of attention to academia and industry. Because of the sparse user-item interaction, cold-start recommendation has been a particularly difficult problem. Some efforts have been made to solve the cold-start problem by using model-agnostic meta-learning on the level of the model and heterogeneous information networks on the level of data. Moreover, using the memory-augmented meta-optimisation method effectively prevents the meta-learning model from entering the local optimum. As a result, this paper proposed memory-augmented meta-learning on meta-path, a new meta-learning method that addresses the cold-start recommendation on the meta-path furthered. The meta-path builds at the data level to enrich the relevant semantic information of the data. To achieve fast adaptation, semantic-specific memory is utilised to conduct the model with semantic parameter initialisation, and the method is optimised by a meta-optimisation method. We put this method to the test using two widely used recommended data set and three cold-start scenarios. The experimental results demonstrate the efficiency of our proposed method.
更多查看译文
关键词
Cold-start recommendation,memory-augmented,meta-path,meta-learning
AI 理解论文
溯源树
样例
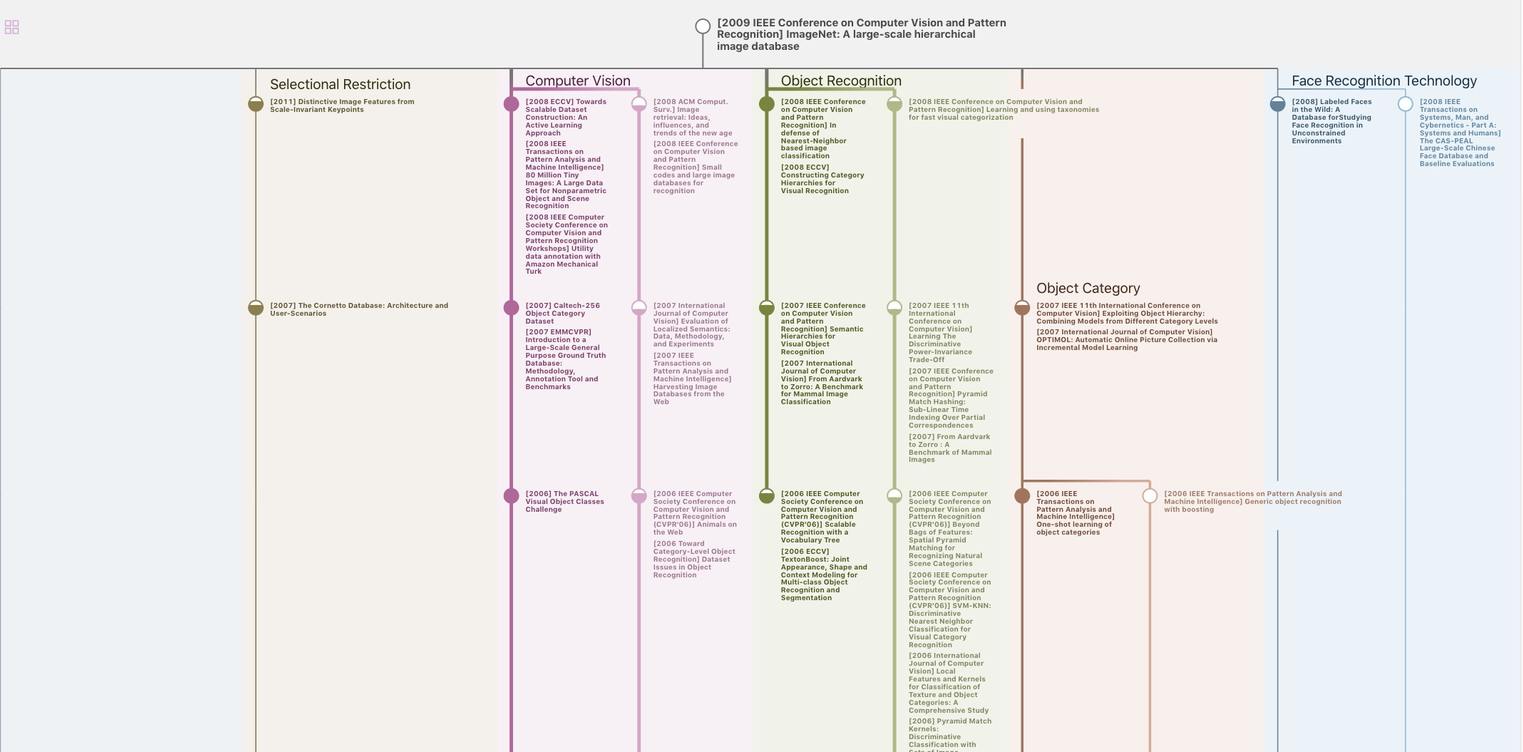
生成溯源树,研究论文发展脉络
Chat Paper
正在生成论文摘要