Local Spatial Constraint and Total Variation for Hyperspectral Anomaly Detection
IEEE TRANSACTIONS ON GEOSCIENCE AND REMOTE SENSING(2022)
摘要
Hyperspectral anomaly detection, which is aimed at locating anomaly, has received widespread attention. In this article, a new anomaly detector, named local spatial constraint and total variation (LSC-TV), is proposed for hyperspectral imagery. In anomaly detection methods based on low-rank representation, background pixels are usually considered to have a global low-dimensional structure. However, the complex background distribution in hyperspectral images (HSIs) means that this global low-dimensional structure rarely occurs. In LSC-TV, the effective local spatial information is extracted by superpixel segmentation, and the regularization based on the F-norm is used to force the background within the same superpixel to show uniform spectral features. Moreover, each pixel is given a penalty based on the degree of anomaly determined during model iteration, while the anomaly is not considered by the background constraint. In addition, the background pixels in the neighborhood often show a high correlation, whereas the anomaly does not possess this feature. Nonisotropic TV is introduced into the proposed LSC model using the correlation of first-order neighborhoods to make it easier for anomalies to be separated. The proposed LSC-TV method and current state-of-the-art methods are tested on a set of simulated data and four sets of real data. The experimental results demonstrate that the proposed method is superior to the comparative method in terms of both color map detection and quantitative evaluation.
更多查看译文
关键词
Anomaly detection,Hyperspectral imaging,Detectors,Dictionaries,TV,Matrix decomposition,Correlation,Anomaly detection,hyperspectral imagery,local idea,matrix decomposition,spatial information,superpixel segmentation,total variation
AI 理解论文
溯源树
样例
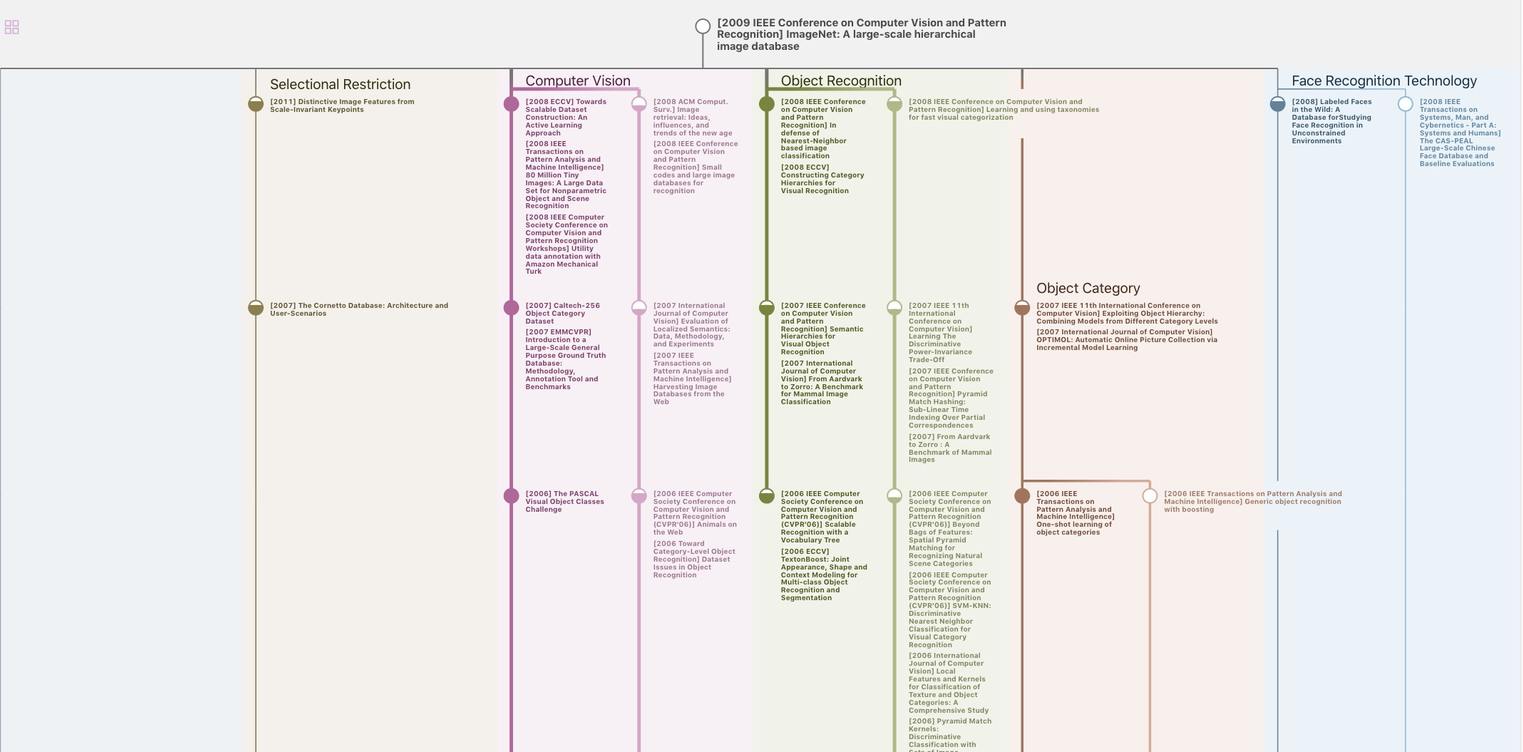
生成溯源树,研究论文发展脉络
Chat Paper
正在生成论文摘要