Remote Sensing Object Detection Based on Receptive Field Expansion Block
IEEE GEOSCIENCE AND REMOTE SENSING LETTERS(2022)
摘要
Due to the rapid development of deep learning techniques and the collection of large-scale remote sensing datasets, convolutional neural networks (CNNs) have made significant progress in remote sensing object detection. However, due to the diversity of objects in remote sensing images, multiscale object detection is still a challenging task. In this letter, a novel object detection framework based on feature pyramid network (FPN) is proposed to improve the detection performance of multiscale objects. First, a receptive field expansion block (RFEB) is designed and added on the top of the backbone to expand the receptive field of FPN adaptively. In this way, the context information around each object is well captured. Then, the features obtained via RFEB are delivered to feature maps at all pyramid levels, remedying the drawback of FPN that semantic information captured by deep layers is gradually diluted when transmitted to lower layers. Third, since the classic backbone of FPN, which produces large receptive fields based on large downsampling factors, may limit the effectiveness of RFEB, the backbone of the original FPN is modified using dilated convolution to ease the resolution drop of feature maps while maintaining a large receptive field. As a feature extractor, the proposed framework can be easily deployed in other FPN-based methods. The experiments on the benchmark for object DetectIon in Optical Remote sensing images (DIOR) dataset demonstrate the proposed method's superiority over considered state-of-the-art baseline methods in terms of detection accuracy.
更多查看译文
关键词
Convolution,Remote sensing,Object detection,Feature extraction,Semantics,Convolutional neural networks,Training,Convolutional neural networks (CNNs),dilated convolution,object detection,receptive field expansion block (RFEB),remote sensing
AI 理解论文
溯源树
样例
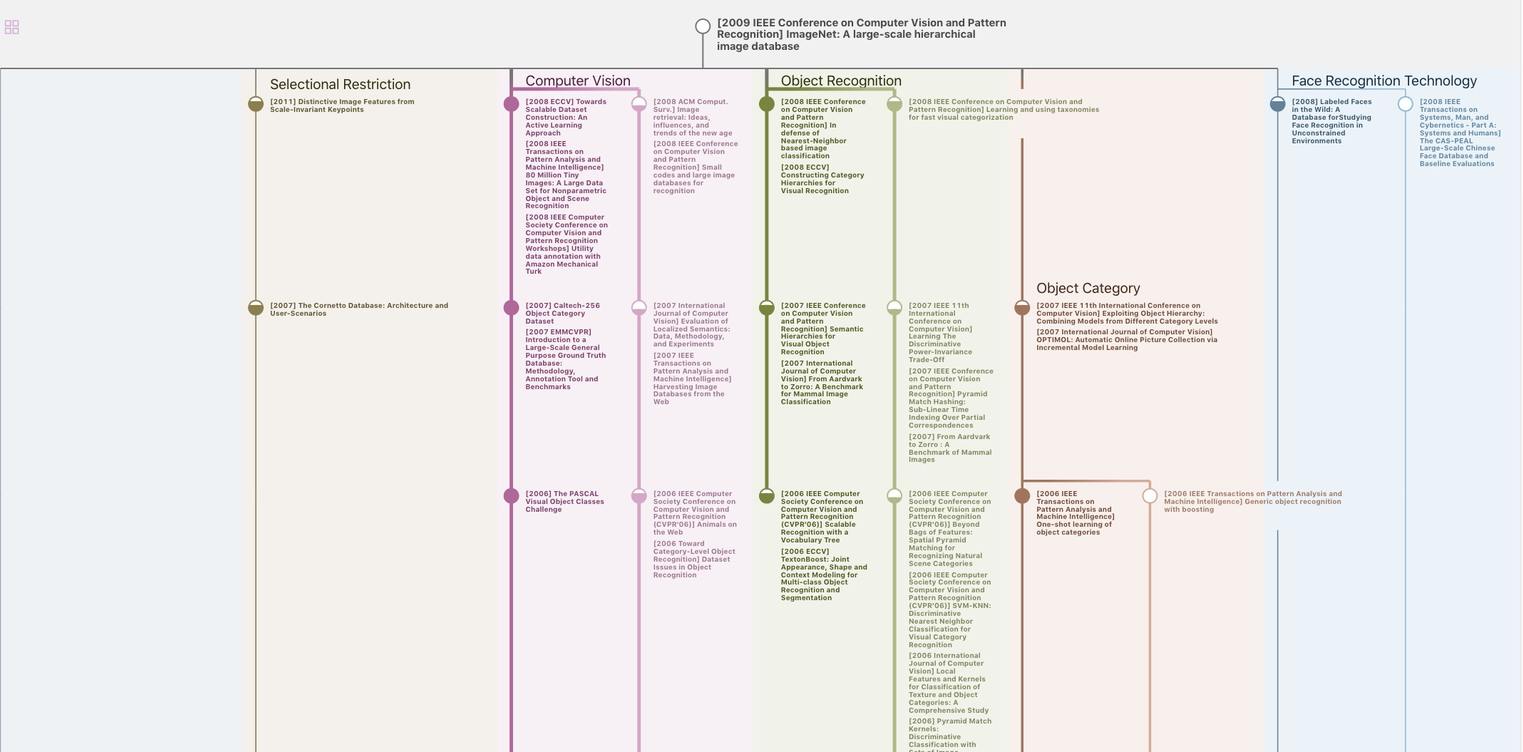
生成溯源树,研究论文发展脉络
Chat Paper
正在生成论文摘要