PCA-Domain Fused Singular Spectral Analysis for Fast and Noise-Robust Spectral–Spatial Feature Mining in Hyperspectral Classification
IEEE Geoscience and Remote Sensing Letters(2023)
摘要
The principal component analysis (PCA) and 2-D singular spectral analysis (2DSSA) are widely used for spectral- and spatial-domain feature extraction in hyperspectral images (HSIs). However, PCA itself suffers from low efficacy if no spatial information is combined, while 2DSSA can extract the spatial information yet has a high computing complexity. As a result, we propose in this letter a PCA domain 2DSSA approach for spectral–spatial feature mining in HSI. Specifically, PCA and its variation, folded PCA (FPCA) are fused with the 2DSSA, as FPCA can extract both global and local spectral features. By applying 2DSSA only on a small number of PCA components, the overall computational cost can be significantly reduced while preserving the discrimination ability of the features. In addition, with the effective fusion of spectral and spatial features, our approach can work well on the uncorrected dataset without removing the noisy and water absorption bands, even under a small number of training samples. Experiments on two publicly available datasets have fully validated the superiority of the proposed approach, in comparison to several state-of-the-art methods and deep learning models.
更多查看译文
关键词
hyperspectral classification,spectral–spatial feature mining,pca-domain,noise-robust
AI 理解论文
溯源树
样例
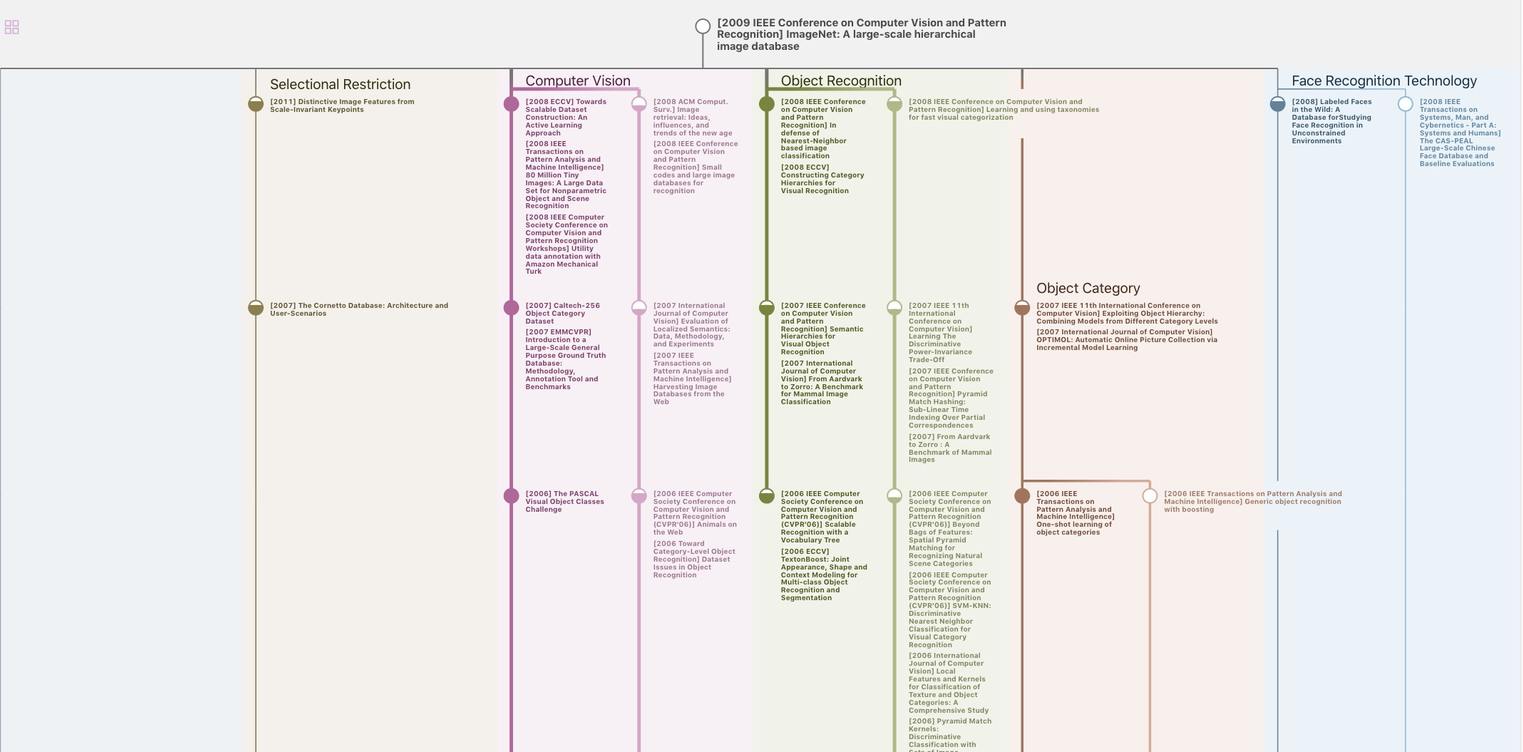
生成溯源树,研究论文发展脉络
Chat Paper
正在生成论文摘要