PRIORITIZATION ON WHOLE-SLIDE IMAGES OF CLINICAL GASTRIC CARCINOMA BIOPSIES THROUGH A WEAKLY SUPERVISED AND ANNOTATION-FREE SYSTEM
Gut(2021)
摘要
BackgroundRecent advances in digital pathology and artificial intelligence provided the potential for the development of robust clinical diagnostic tools to support pathologists. With a worldwide shortage of pathologists and increased cancer screening programs, digital pathology is expected to grow and will revolutionize the workflow of pathologists. However, the development is hindered by the time-consuming whole slide imaging (WSI) annotation process during model training and lack of experience in integration into clinical workflow. This study aims to target these issues by developing a weakly supervised and annotation-free system to triage suspicious gastric carcinoma biopsies.MethodsWe digitized 965 (2795 images) gastric biopsy cases from Prince of Wales Hospital, including 648 (2062 images) ,192 (576 images) and 125 (157 images) cases for model development and as first and second external validation sets respectively to evaluate model performance. A neural network-based binary classifier for cancer detection was trained using 41 averaged cellular features generated from WSI and case diagnostic labels. Accuracy and AUC were measured for model performance. To evaluate our model to carcinoma case prioritization, another 90 cases (122 images) were digitized. 16 pathologists from 4 centers were recruited for a randomized study to review these cases in a simulated clinical workflow, with and without the assistance of our system. The final diagnosis was compared to ground truth and time spend per case was measured to demonstrate the clinical utility of triage.ResultsWe developed a weakly supervised system (GastroFLOW), achieving the accuracy of 78-83% and AUC of 0.92-0.95 on external validation sets, which can skip 69-78% of the non-carcinoma cases while retaining 100% sensitivity. In a simulated pathology clinical setting, GastroFLOW prioritized all CA cases forward. Moreover, 52.29-54.79% time was saved from viewing all non-carcinoma cases as compared with random scenarios (IDDF2021-ABS-0100 Figure 1).ConclusionsIn summary, we developed a clinical decision support tool from annotation-free WSI, based on automatically generated cellular features for gastric carcinoma. GastroFLOW allows optimization of daily pathologist workload to meet case turn-around-time promptly for urgent cases, improving cancer patient management, paving the way for the application of computational pathology in the workflow of a histopathology laboratory.
更多查看译文
关键词
Whole Slide Imaging,Cancer Imaging,Digital Pathology,Medical Image Analysis,Histopathology Images
AI 理解论文
溯源树
样例
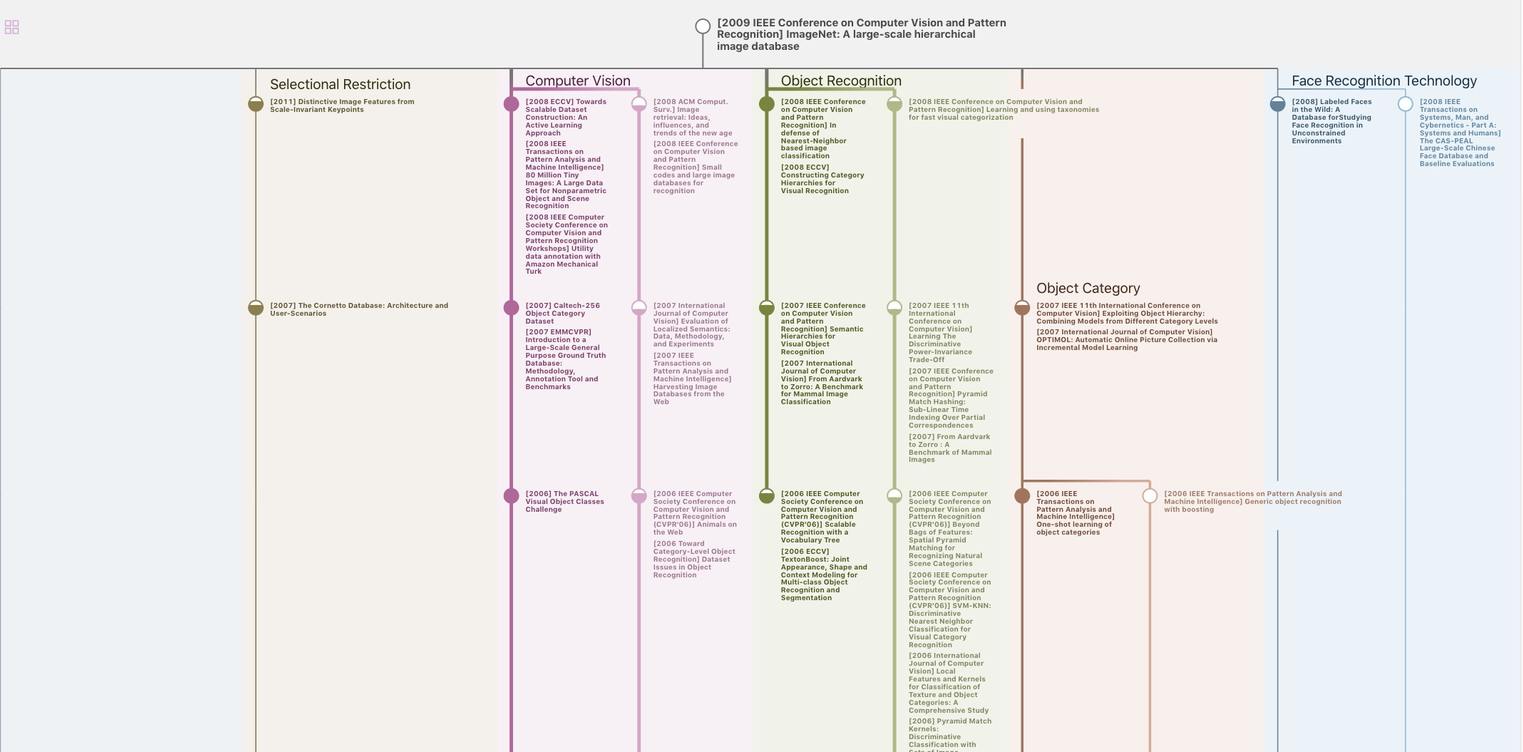
生成溯源树,研究论文发展脉络
Chat Paper
正在生成论文摘要