Cell cycle detection using phase imaging with computational specificity (PICS)
https://doi.org/10.1117/12.2584432(2021)
摘要
Quantitative phase imaging (QPI), with its capability to capture intrinsic contrast within transparent samples, has emerged as an important imaging method for biomedical research. However, due to its label-free nature, QPI lacks specificity and thus faces limitations in complex cellular systems. In our previous works, we have proposed phase imaging with computational specificity (PICS), a novel AI-enhanced imaging approach that advances QPI by utilizing deep learning for specificity. Here we present that PICS can be applied to study individual cell behavior and cellular dry mass change across different phases of the cell cycle. The cell cycle information is traditionally obtained by fluorescence microscopy with markers like Fluorescence Ubiquitin Cell Cycle Indicator (FUCCI). Our work showed that using deep learning, we can train a neural network to accurately predict the cell cycle phase (G1, S, or G2) for each individual cell.
更多查看译文
AI 理解论文
溯源树
样例
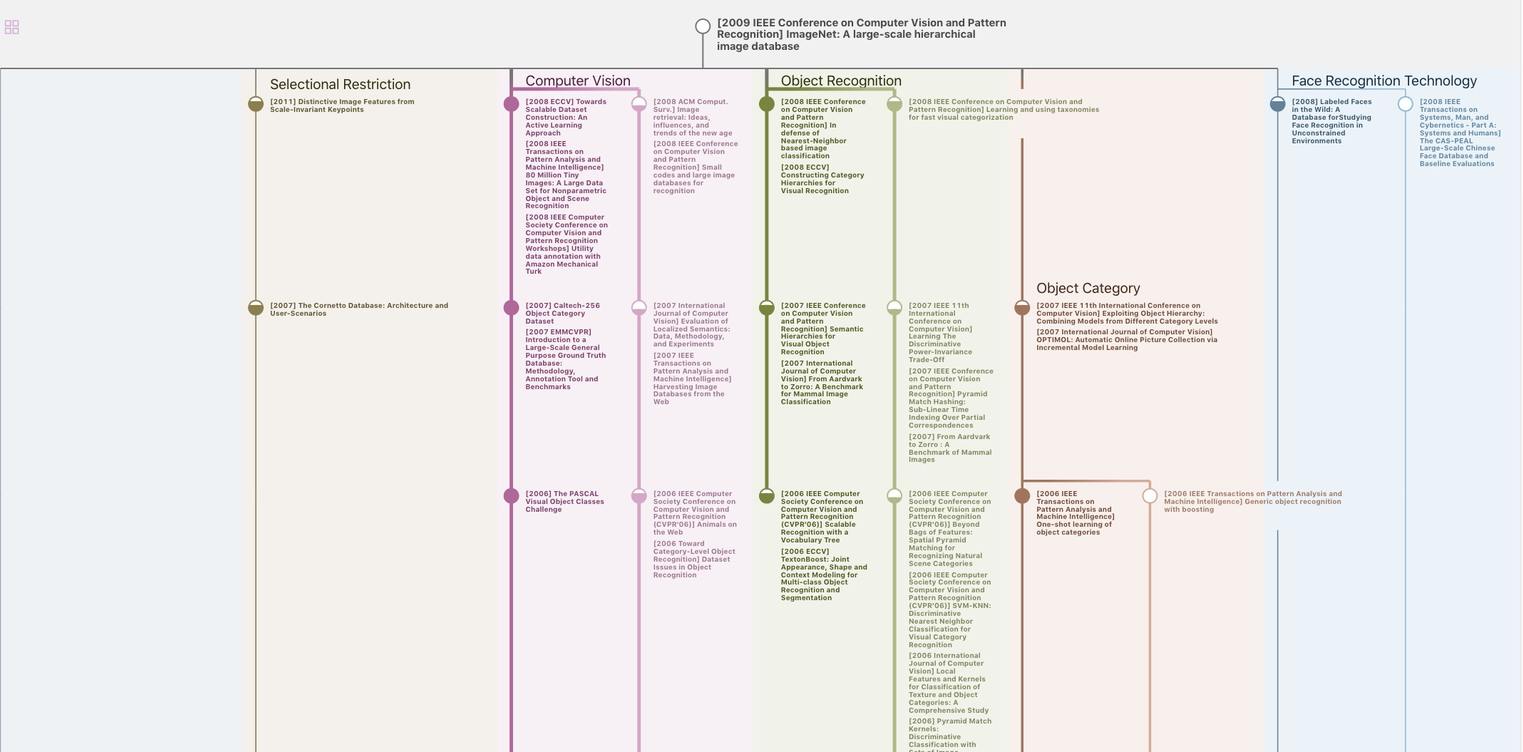
生成溯源树,研究论文发展脉络
Chat Paper
正在生成论文摘要