Learning the Spatial Perception and Obstacle Avoidance with the Monocular Vision on a Quadrotor
international conference on mechatronics and automation(2021)
摘要
We present a learning-based framework to simultaneously realize spatial perception and obstacle avoidance in this paper. It learns to extract the visual representations of obstacles from raw monocular images using unsupervised contrastive learning. Moreover, the framework utilizes the dueling double deep recurrent Q network to learn the optimal obstacle avoidance policy using the extracted representation features. The learned policy in the framework can reduce the side effects of the onboard fixed camera's limited observation capacity by adding recurrency to stack a history of observations. Compared with other typical obstacle avoidance methods, the proposed framework is more light weighted and data-efficient. The framework is trained and evaluated in several simulation scenarios, which are built in the ROS Gazebo environment. The trained framework is capable to control the quadrotor to pass through the crowded environments in evaluation.
更多查看译文
关键词
Unmanned aerial vehicle,Obstacle avoidance,Contrastive learning,Deep reinforcement learning
AI 理解论文
溯源树
样例
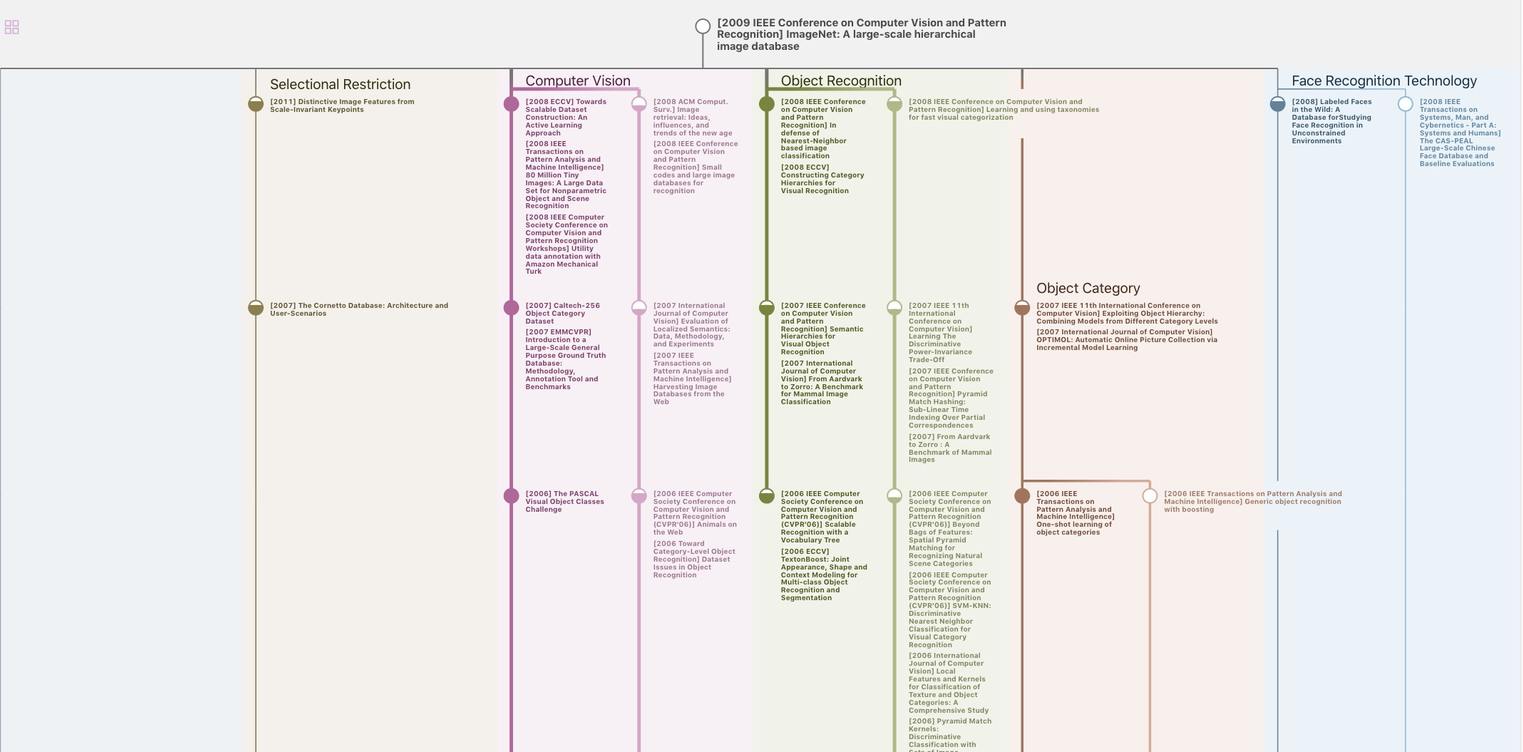
生成溯源树,研究论文发展脉络
Chat Paper
正在生成论文摘要