Off-policy algorithm based Hierarchical optimal control for completely unknown dynamic systems
Neurocomputing(2022)
摘要
This paper proposes an online reinforcement learning(RL) for solving Stackelberg games with completely unknown dynamic systems. To deal with the hierarchical optimal control problem, the key is to find the solution of a two-level optimal control with the leader’s optimal problem being constrained. Firstly, the leader–follower coupled Hamiltonian-Jacobi(HJ) equations subject to the follower’s costate equation is derived. Secondly, an off-policy scheme is designed following the policy iteration(PI) algorithm based on model. The improved off-policy algorithm is given for obtaining the solution to the leader–follower coupled HJ equations. The algorithm is built without requiring any knowledge of the dynamic system, and the leader–follower optimal control policies are directly constructed by the extracted data. Meanwhile, the existence of the Stackelberg equilibrium is demonstrated. Lastly, NNs for each player are built, and NN learning is accomplished online with Kronecker product technique and vectorization, which simples the NN form and could decrease computation burden. Simulation examples are presented to demonstrate the proposed learning algorithm.
更多查看译文
关键词
Stackelberg games,Reinforcement learning,Hierarchical control,Off-policy
AI 理解论文
溯源树
样例
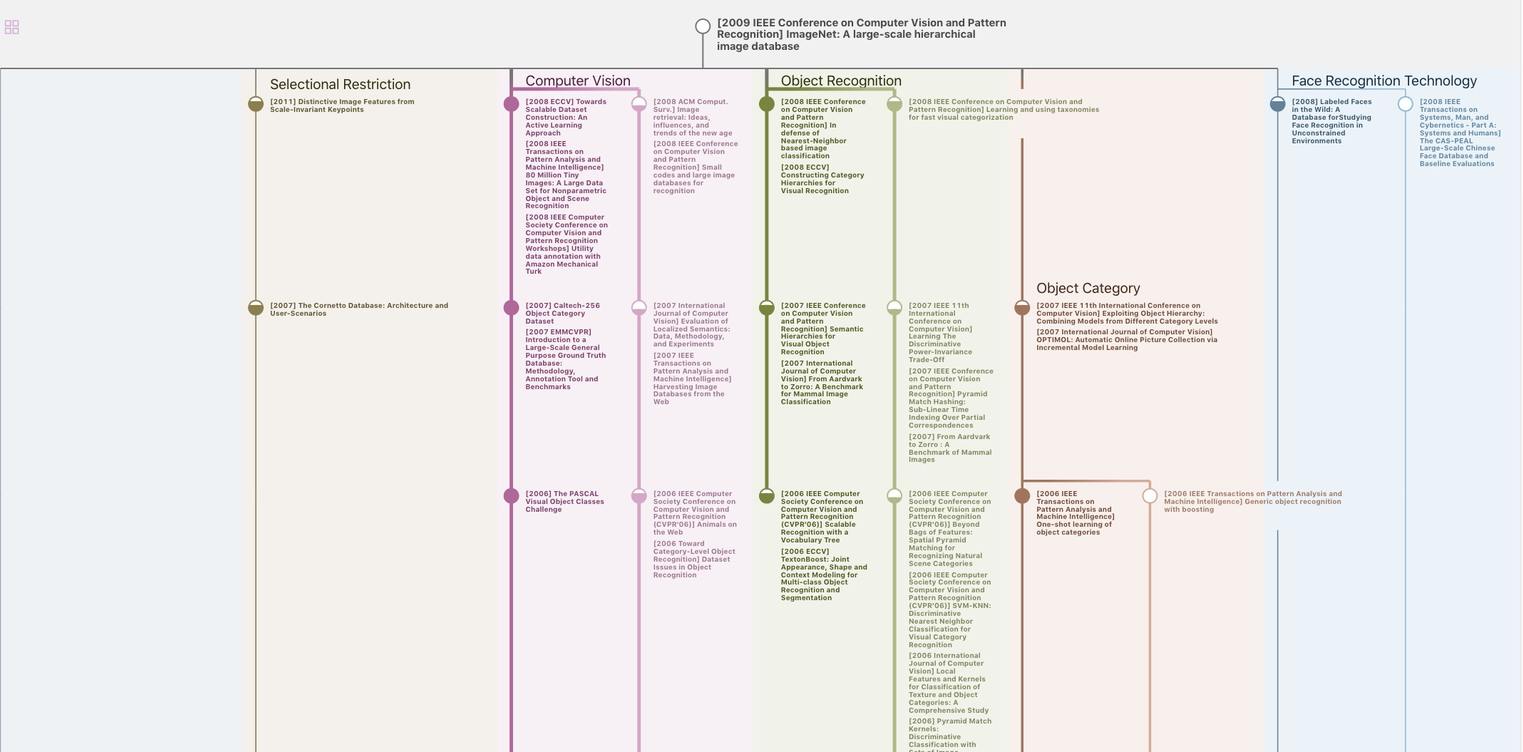
生成溯源树,研究论文发展脉络
Chat Paper
正在生成论文摘要