Oil Spill Identification and Monitoring from Sentinel-1 Sar Satellite Earth Observations: a Machine Learning Approach
Chemical engineering transactions(2021)
摘要
Identification of an oil spill is essential to evaluate the potential spread and float from the source to coastal terrains, and their continued monitoring is essential for managing the environmental protection actions to confine the pollution and avoid further damage. The SAR sensor is perceived as the most significant remote sensing apparatus for the oil slick examination. One of the main aspects of oil spreading over sea surface is that it dampens the capillary waves and so, the backscatter radio waves are suppressed. As a result, oil spills are represented as black spots, while the brighter regions are usually related with unspoiled polluted sea areas. Additionally, the wide coverage that the sensor can provide is highly significant including long-range fate, as well as contextual information, such as sensitive coastal areas or vessels, which can be enclosed in the acquired image. However, oceanic natural phenomena such as low wind speed regions, weed beds and algae blooms, wave shadows behind land, grease ice, etc. can also be depicted as dark spots. These dark regions are commonly categorized as “look-alikes and their discrimination is very challenging. Machine Learning techniques are the most appropriate choice to classify oil spills and look-alikes. In the present work, a comparison between decision trees models and NN is performed to identify and extract the appropriate set of features characterizing an oil spill allowing effective evolution monitoring and setting up proper emergency actions.
更多查看译文
AI 理解论文
溯源树
样例
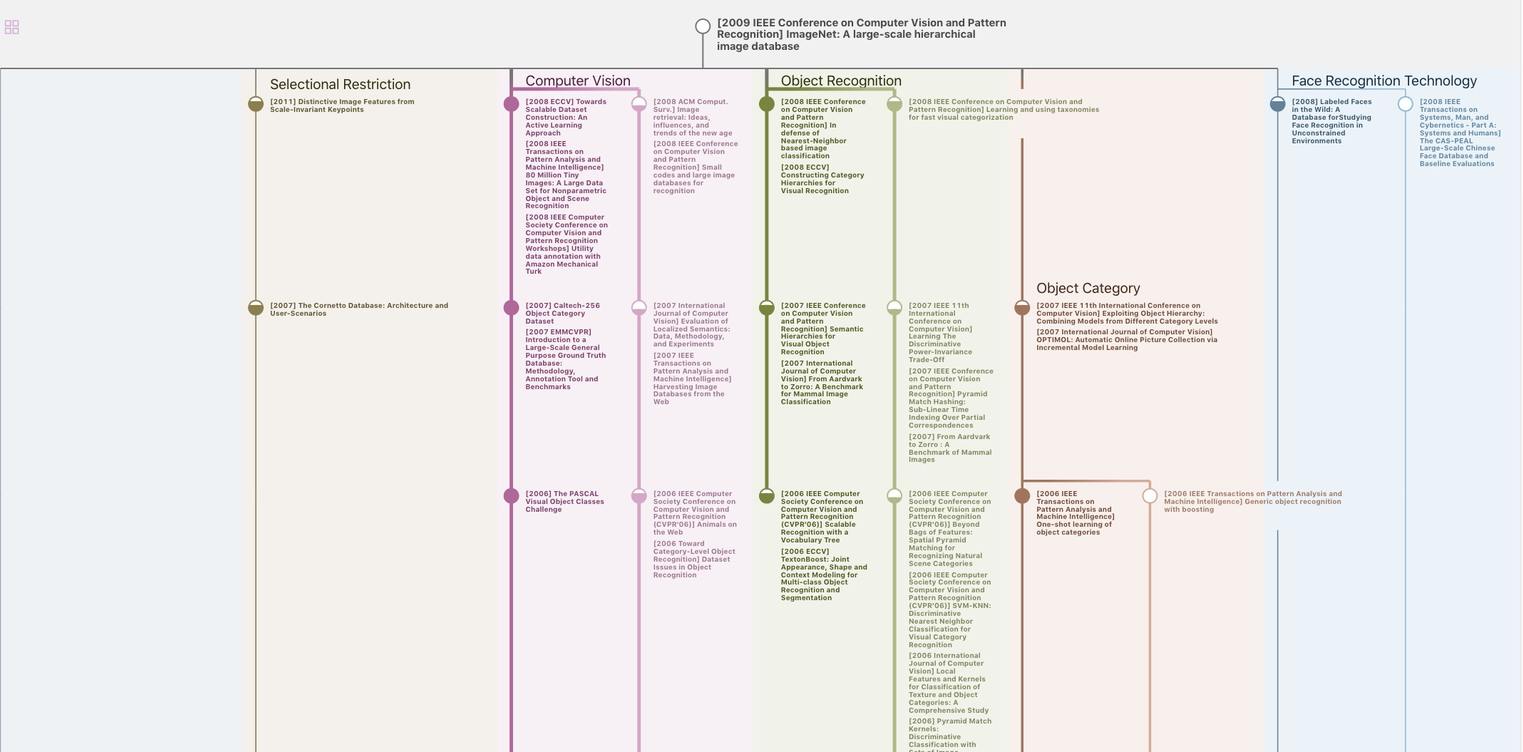
生成溯源树,研究论文发展脉络
Chat Paper
正在生成论文摘要