Tensor Product and Tensor-Singular Value Decomposition Based Multi-Exposure Fusion of Images
IEEE TRANSACTIONS ON MULTIMEDIA(2022)
摘要
Considering multidimensional structure of the multi-exposure images, a new Tensor product and Tensor-singular value decomposition based Multi-Exposure image Fusion (TT-MEF) method is proposed. The main innovation of this work is to explore a new feature representation of multi-exposure images in the new tensor domain and design the fusion strategy on this basis. Specifically, the luminance and the chrominance channels are fused separately to maintain color consistency. For the luminance fusion, the luminance channel of multi-exposure images is divided into two parts, that is, de-mean term and mean term. The de-mean term is represented as a tensor to extract the feature. Then, the tensor product and tensor-singular value decomposition (T-SVD) are used to design a tensor feature extractor. Furthermore, a fusion strategy of the de-mean term is presented according to the visual saliency model, and a fusion strategy of the mean term is defined by the local and the global visual weights to control counterpoise between the local and global luminance. For the chrominance fusion, a new fusion strategy is also designed by the tensor product and T-SVD, similar to the luminance fusion. Finally, the fused image is obtained by combining the luminance and chrominance fusion. Experimental results show that the proposed TT-MEF method generally outperforms the existing state-of-the-art in terms of subjective visual quality and objective evaluation.
更多查看译文
关键词
Tensors,Feature extraction,Multi-exposure image fusion,tensor product,tensor-singular value decomposition,visual saliency
AI 理解论文
溯源树
样例
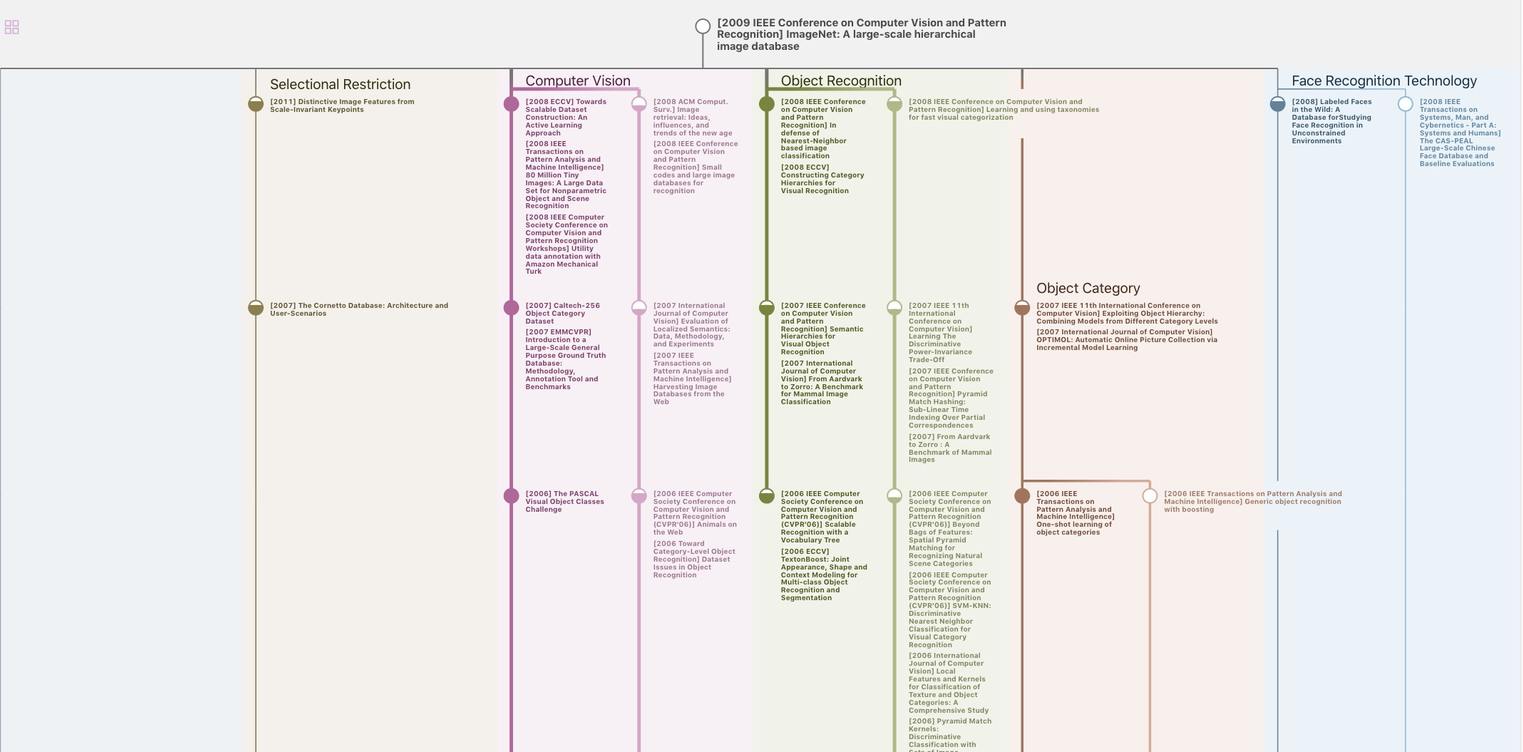
生成溯源树,研究论文发展脉络
Chat Paper
正在生成论文摘要