Backdoor Attack on Machine Learning Based Android Malware Detectors
IEEE Transactions on Dependable and Secure Computing(2022)
摘要
Machine learning (ML) has been widely used for malware detection on different operating systems, including Android. To keep up with malware's evolution, the detection models usually need to be retrained periodically (e.g., every month) based on the data collected in the wild. However, this leads to poisoning attacks, specifically backdoor attacks, which subvert the learning process and create evasion ‘tunnels’ for manipulated malware samples. To date, we have not found any prior research that explored this critical problem in Android malware detectors. Although there are already some similar works in the image classification field, most of those similar ideas cannot be borrowed to solve this problem, because the assumption that the attacker has full control of the training data collection or labelling process is not realistic in real-world malware detection scenarios. In this article, we are motivated to study the backdoor attack against Android malware detectors. The backdoor is created and injected into the model stealthily without access to the training data and activated when an app with the trigger is presented. We demonstrate the proposed attack on four typical malware detectors that have been widely discussed in academia. Our evaluation shows that the proposed backdoor attack achieves up to 99 percent evasion rate over 750 malware samples. Moreover, the above successful attack is realised by a small size of triggers (only four features) and a very low data poisoning rate (0.3 percent).
更多查看译文
关键词
Malware detection,backdoor attack,machine learning,computer security,data poisoning
AI 理解论文
溯源树
样例
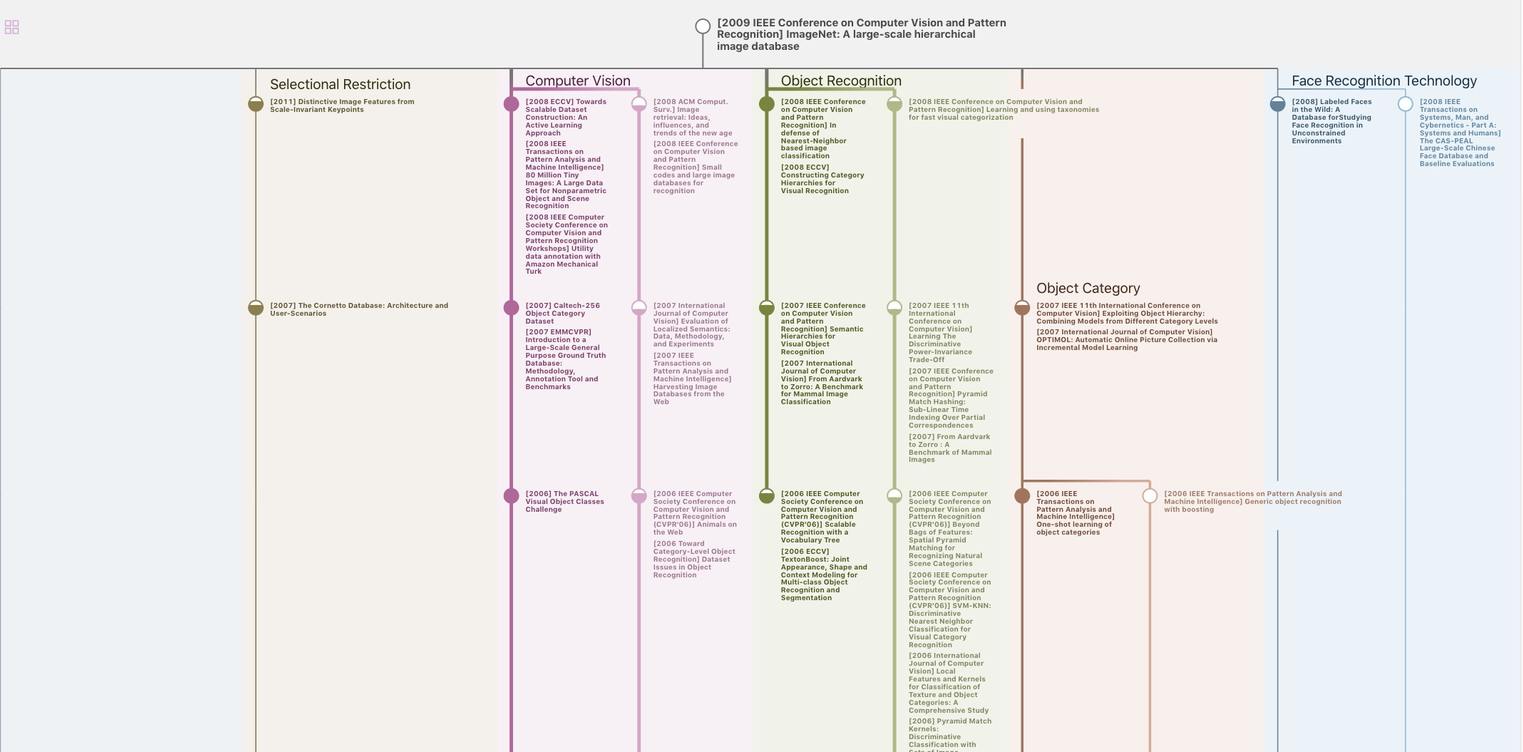
生成溯源树,研究论文发展脉络
Chat Paper
正在生成论文摘要