Tensor-Based Robust Principal Component Analysis With Locality Preserving Graph and Frontal Slice Sparsity for Hyperspectral Image Classification
IEEE TRANSACTIONS ON GEOSCIENCE AND REMOTE SENSING(2022)
摘要
Tensor-based robust principal component analysis (PCA) methods are efficient to discover the low-rank part of a hyperspectral image for reducing redundant information and guarantee good classification results. However, current methods cannot remove noise adequately, and the residual noise remaining in the low-rank image limits the further improvement of classification performance. Thus, enhancing the robustness to noise is important and helpful for tensor-based robust PCA (RPCA) methods to process hyperspectral images. To this end, we propose a tensor-based RPCA method with a locality preserving graph and frontal slice sparsity (LPGTRPCA) for hyperspectral image classification. Specifically, a tensor $l_{2,2,1}$ norm that requires the frontal slice sparsity of a tensor is defined to extract the noise in the hyperspectral image from the frontal direction. What is more, a position-based Laplacian graph that preserves the local structures of a tensor according to the spatial position is designed for relieving the impact of the residual noise remaining in the low-rank image. Based on the tensor nuclear norm, the tensor $l_{2,2,1}$ norm, and the position-based Laplacian graph, LPGTRPCA efficiently separates the low-rank part with little noise from a raw hyperspectral image and achieves more robust classification results than current methods. LPGTRPCA is optimized by the alternative direction multiplier method (ADMM), and the convergence of solutions is experimentally demonstrated. In the experiments conducted on Indian Pines, Pavia University, and Salinas datasets, LPGTRPCA outperformed various state-of-the-art and classical tensor-based RPCA methods in terms of average class classification accuracy (AA), overall classification accuracy (OA), and kappa coefficient (KC).
更多查看译文
关键词
Tensors,Hyperspectral imaging,Principal component analysis,Sparse matrices,Laplace equations,Matrix decomposition,Sun,Frontal slice sparsity,hyperspectral image classification,locality preserving graph,tensor-based robust principal component analysis (PCA)
AI 理解论文
溯源树
样例
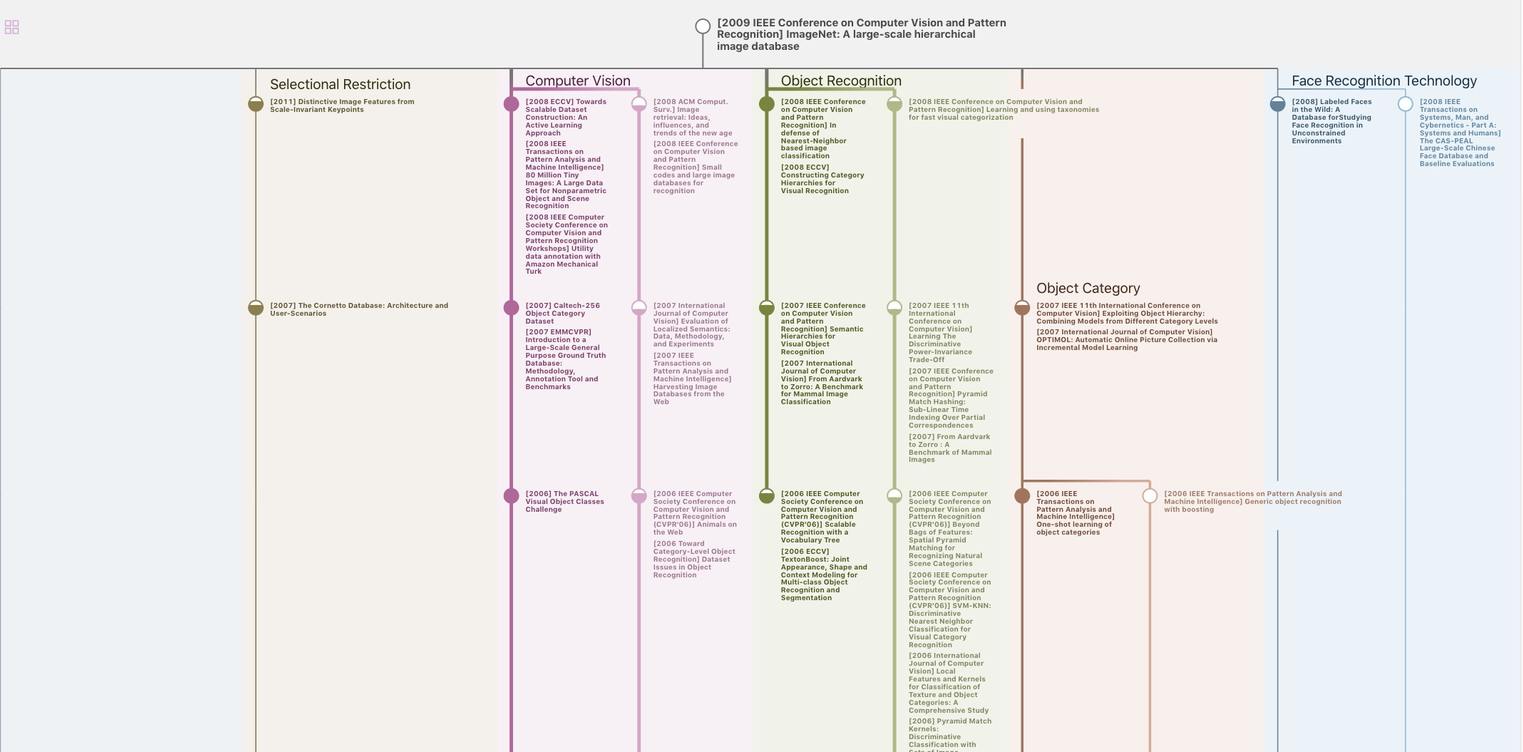
生成溯源树,研究论文发展脉络
Chat Paper
正在生成论文摘要