The application of CNN-LightGBM algorithm in remaining useful life prediction
international conference on information science and control engineering(2020)
摘要
The remaining useful life (RUL) prediction is an important content of aero-engines prediction and health management. There are many single models to research the RUL. However, many complexity equipment has the character of many state variables and severe nonlinear features, and a single model faces the challenge of feature extraction inadequately. The lack of prediction accuracy makes it difficult to predict the RUL of machine’s accuracy. To address this problem, a new type of model combined with Convolutional Neural Network (CNN) and LightGBM’s integrated learning method is approached to RUL’s prognosis. The features of the raw data are extracted by convolutional neural network firstly, then the output of the convolutional neural network is used as the inputs of LightGBM model for RUL predictions. In order to validate the effectiveness of above approach, experiments on the dataset provided by NASA (C-MAPSS) are carried out, and the result shows that the prediction error after fusing CNN with LightGBM is decreased significantly compared with the same parameter settings CNN and LightGBM models alone, which validate the efficiency of the integrated model for remaining useful life prediction.
更多查看译文
关键词
Remaining useful life,Ensemble learning,CNN-LightGBM
AI 理解论文
溯源树
样例
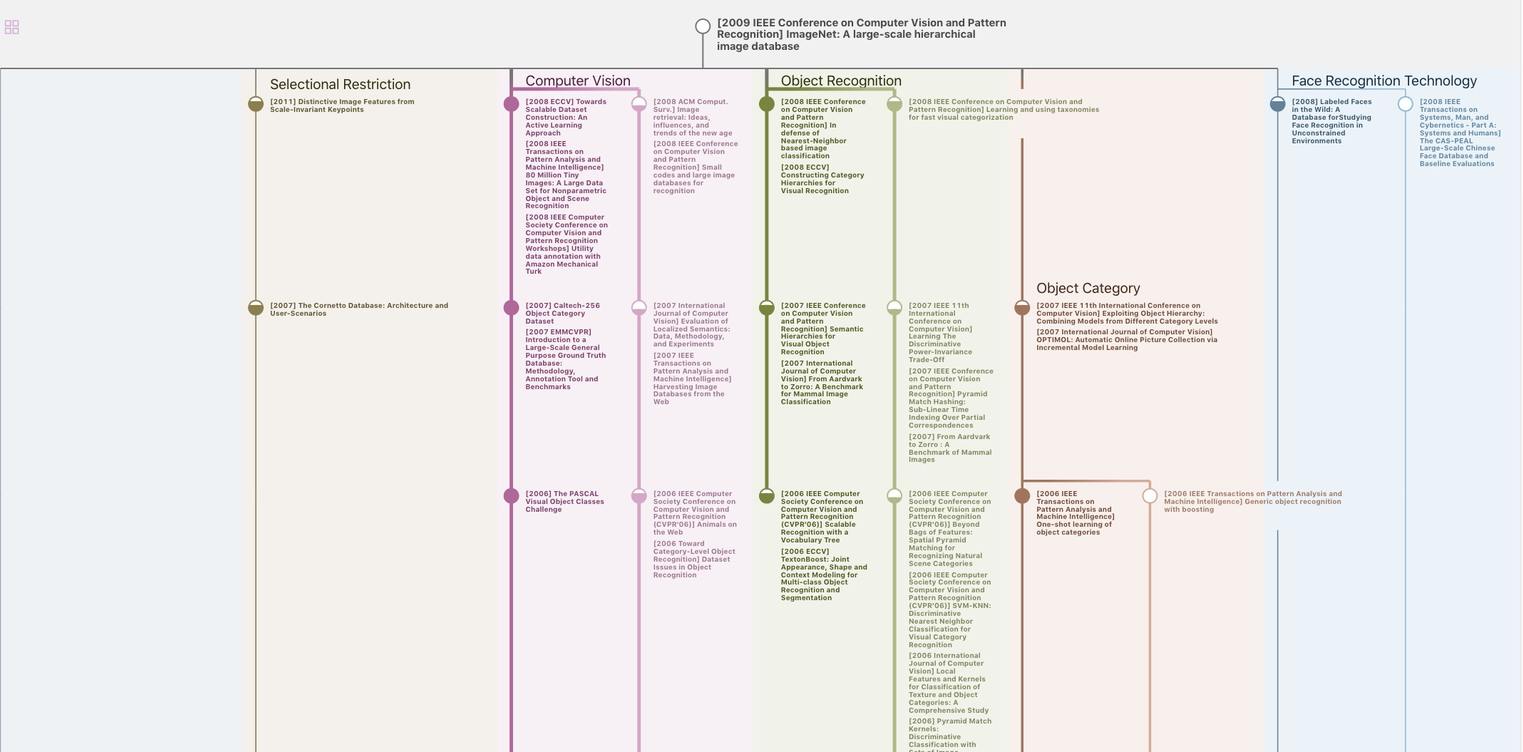
生成溯源树,研究论文发展脉络
Chat Paper
正在生成论文摘要