Semi-supervised Image Annotation with Parallel Graph Convolutional Networks
chinese control conference(2021)
摘要
Automatic image annotation has increasingly exerted a tremendous fascination on researchers with the development of digital imaging in recent years. First, most works exploit the sufficient labeled data to train the models and trigger the unfavorable experimental performance in semi-supervised learning. Second, some examinations solve the semi-supervised problem only by a samples graph or tags graph, limiting in improving the annotation results owing to the incomplete data structure. To this end, we propose a method called Semi-supervised Image Annotation with Parallel Graph Convolutional Networks (SPGCN). This algorithm combines graph convolutional networks (GCN) with image annotation to promote annotation performance under semi-supervised learning. Furthermore, SPGCN, connecting the tags graph with the samples graph, is proposed to improve annotation results, further considering tags’ distribution and features’ distribution to aggregate the features. Experiments on three benchmark image annotation datasets show that our approach outperforms other existing state-of-the-art methods.
更多查看译文
关键词
Semi-supervised,Parallel Graph Convolutional Networks,Multi-graph,Image Annotation
AI 理解论文
溯源树
样例
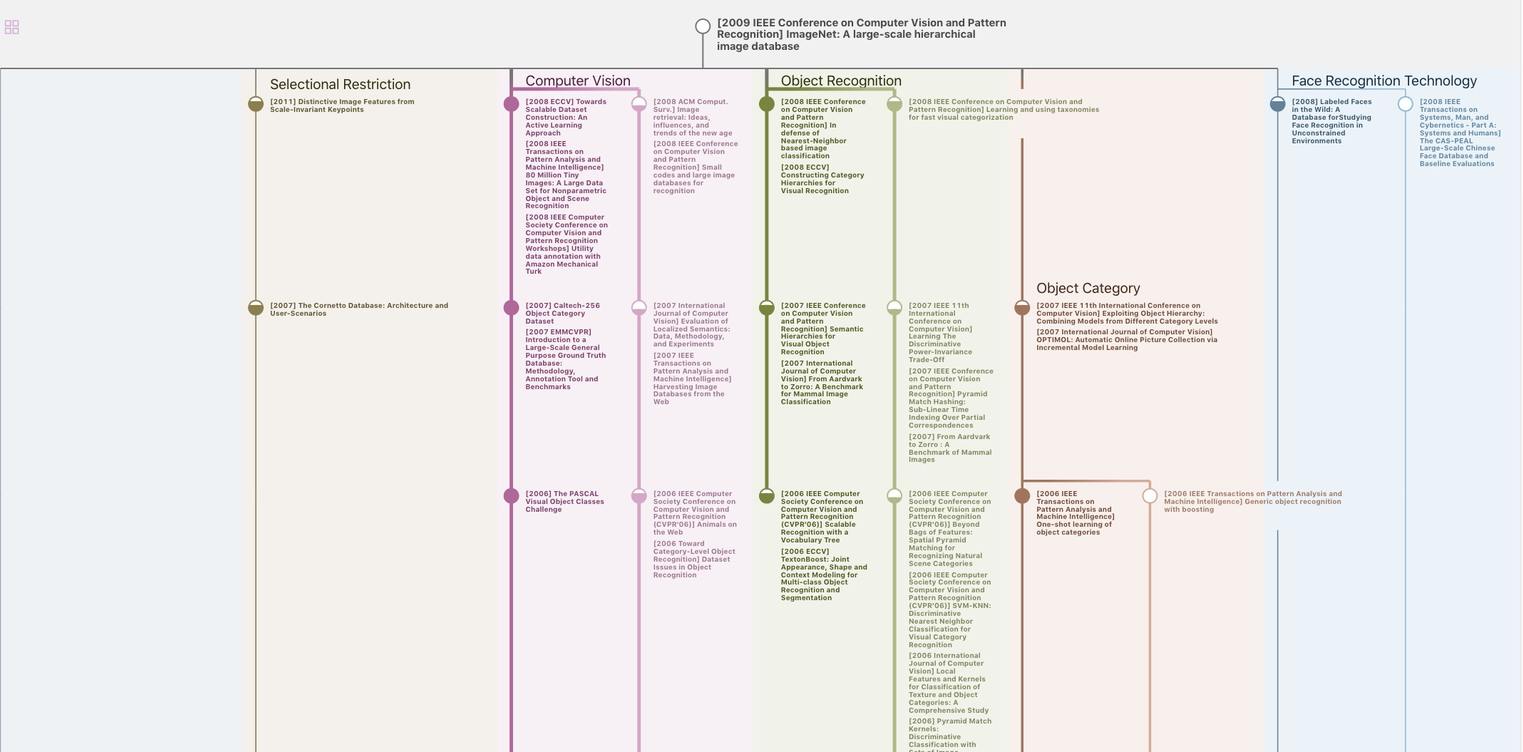
生成溯源树,研究论文发展脉络
Chat Paper
正在生成论文摘要