Unsupervised Time Series Anomaly Detection using Moving Memorial Dynamic Filter
chinese control conference(2021)
摘要
Anomaly detection on time series data is something of great importance nowadays. From the unsupervised machine learning method like K-Means to newly developed artificial neural networks, people try very hard to give a precise detection of where the anomalies locate. In this paper, a new method, the moving memorial dynamic filter, which combines Fourier seasonality decomposition, distance transformation and anomaly determination is introduced. First, the seasonal component is split off based on Fourier transformation. Then the time series data is mapped to a center-to-center distance subsequence using a dynamic circumscribed circle filter, which could use a low dimensional series to represent high dimensional information. At last, moving memorial value at risk is introduced and serves as a threshold to determine whether a certain data point should be labeled as an anomaly. Our method is tested and evaluated using the Numenta Anomaly Benchmark, an open-sourced dataset for time series anomaly detection. The result shows that our method outperforms the competing algorithms in terms of accuracy on most profiles of the NAB with a shorter latency in time consumption.
更多查看译文
关键词
anomaly detection, time series data, dynamic filter, real-time detector
AI 理解论文
溯源树
样例
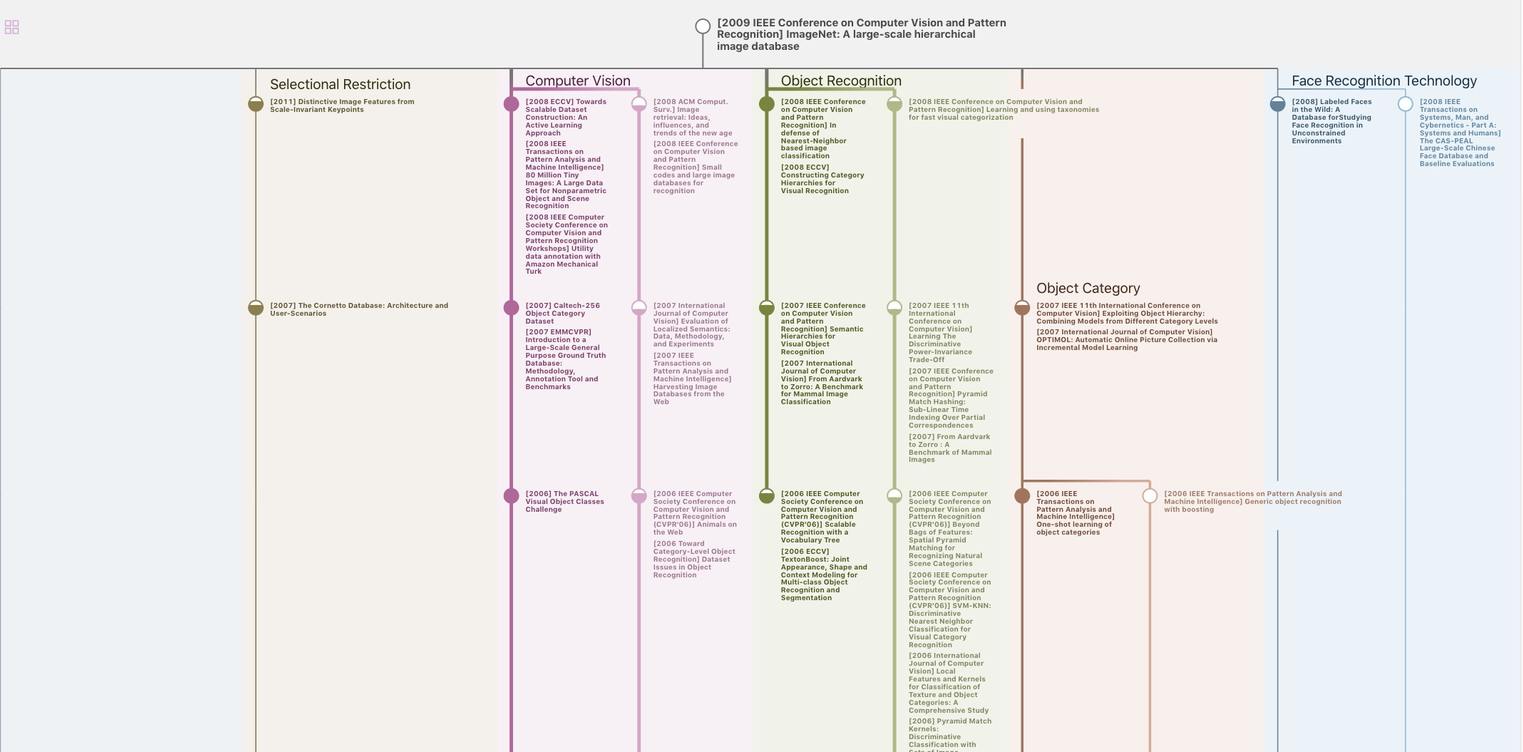
生成溯源树,研究论文发展脉络
Chat Paper
正在生成论文摘要