Dynamic Particle Filter Framework for Robust Object Tracking
IEEE Transactions on Circuits and Systems for Video Technology(2022)
摘要
Most of siamese network and correlation filter (CF) based trackers usually employ the context regression scheme to achieve appealing performance in both accuracy and efficiency. However, they are prone to drifting in challenging situations exhibiting occlusion, out-of-view and large-scale variations due to the lack of failure correction ability in these regressors. Particle filter based trackers can help to recover from tracking failures since several particles of high confidence about the target can be remained for the probability estimation of next frames, but need the large numbers of particles for each frame. In this paper, we propose a generic dynamic particle filter framework, which can reasonably control the number of particles in different scenarios, to improve the robustness of siamese and CF trackers by jointing the target classifier to relieve drifting. Our fundamental insight is that general scenarios are processed efficiently by the context regressor with few particles, while special scenarios are handled effectively by the target classifier with many particles. We propose a novel measure to determine whether to adopt the regressor and few particles to estimate target states with high measure scores, or increase the number of particles to prevent drifting with the proposed multi-template matching strategy in the classifier. In extensive experiments on eight large-scale benchmarks including OTB, UAV, TC128, VOT2017, VOT2019, LaSOT, TrackingNet and Got-10k, the proposed framework enables many basic siamese and CF trackers to operate at least over 24 frames per second and achieve superior tracking performance than themself, as well as the comparable accuracy with the state-of-the-art trackers. Furthermore, our framework is flexible and still has great potential for improvement and generalization.
更多查看译文
关键词
Object tracking,siamese network,correlation filter,dynamic particle filter
AI 理解论文
溯源树
样例
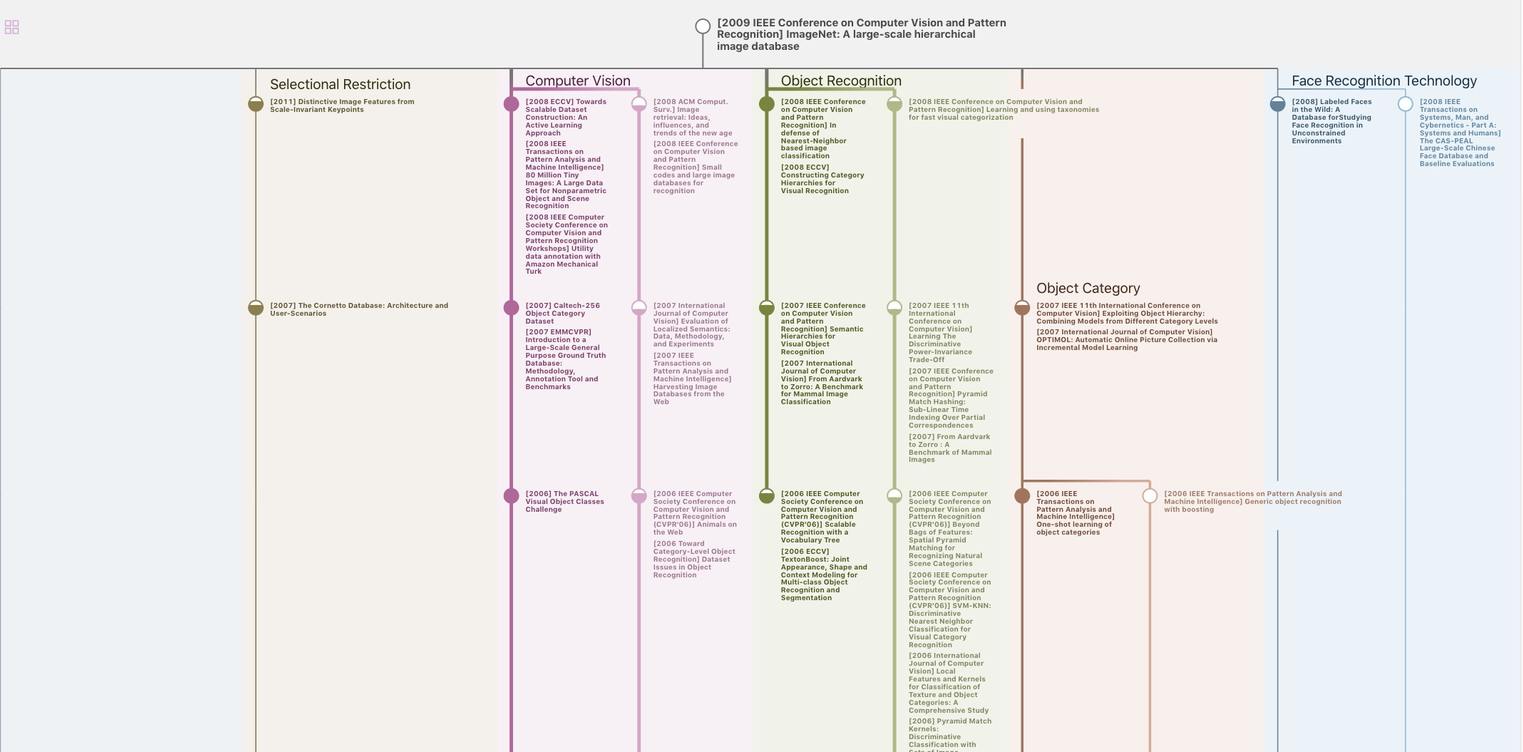
生成溯源树,研究论文发展脉络
Chat Paper
正在生成论文摘要