On-Site Colonoscopy Autodiagnosis Using Smart Internet of Medical Things
IEEE Internet of Things Journal(2022)
Abstract
Colonoscopy screening is one of the most effective diagnostic tools for detecting intestinal diseases, such as bleeding, polyp, Meckel’s diverticulum, and ulcer. However, the missed rate of manual detection is high due to the lack of experience or fatigue among clinicians. To address this issue, this work proposed a novel autodiagnosis framework built on Internet of Medical Things (IoMT) systems, which can be deployed among multiple hospitals in a distributed fusion. This work presents a two-stage knowledge distillation (TSKD) method coupled with Bayesian optimization (BO) that can exploit distributed colonoscopy data to learn a compact diagnostic model achieving a good tradeoff between predictive performance and resource consumption (e.g., memory and computation). The proposed framework is extensively evaluated in real-world data sets in comparison with its counterparts. A prototype of on-site diagnostic device is implemented to demonstrate the potential for real-world deployment.
MoreTranslated text
Key words
Colonoscopy,image segmentation,knowledge distillation,model compression
AI Read Science
Must-Reading Tree
Example
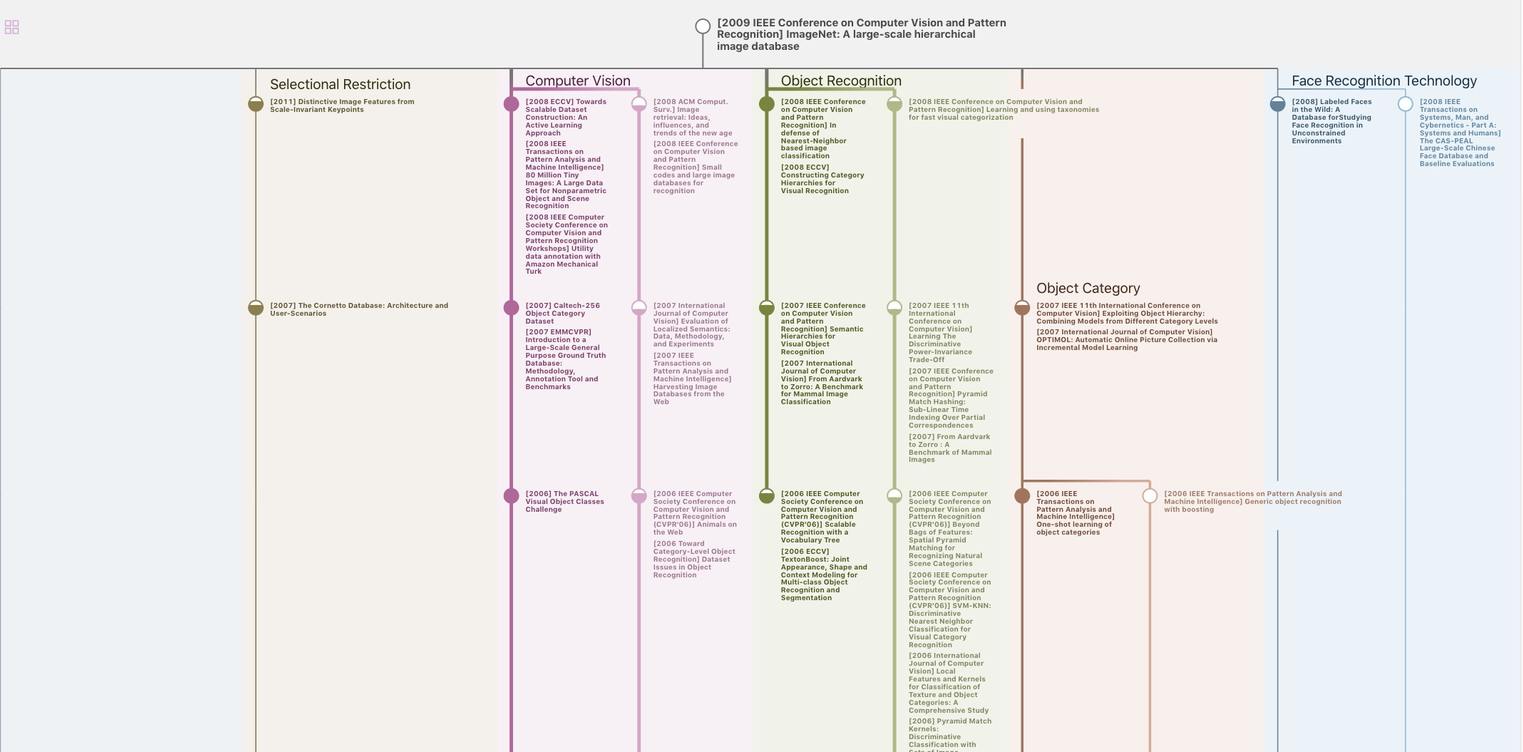
Generate MRT to find the research sequence of this paper
Chat Paper
Summary is being generated by the instructions you defined