Artificial Intelligence for Teleophthalmology-Based Diabetic Retinopathy Screening in a National Program: A Modelled Economic Analysis Study
Social Science Research Network(2019)
摘要
Background: Diabetic retinopathy (DR) screening programs worldwide are currently challenged by manpower and financial constraints. Deep learning systems (DLS) have been shown to be a clinically effective tool to detect DR, but with unknown cost implications. The purpose of this study is to conduct an economic analysis to evaluate two screening models using DLS: a semi-automated (as a “triage” filter prior to secondary human assessment) and a fully-automated (as a complete “replacement” for all human assessment) model against the current full human assessment model (a manual grading system).
Methods: Using 14,880 consecutive patients with diabetes recruited from the Singapore Integrated DR Screening Program (SiDRP) between year 2014 and 2015, a decision tree model is used to compare the total costs associated with the three DR screening models in a hypothetical Singaporean diabetic cohort, over a 12-month time frame. Model parameters included DR prevalence rates, DR screening costs, cost of medical consultation; and diagnostic performance (i.e. sensitivity and specificity) of the three screening models. The outcome measure was patient-level total cost for each of the three screening models incurred within the health system. Deterministic sensitivity analyses were performed. All costs were converted to US dollars from Singaporean dollars.
Findings: From the health system perspective, the semi-automated screening model is the least expensive at US$56/patient compared to the fully-automated model at US$60/patient, and the current human assessment model at US$63/patient. All three screening models yielded comparable diagnostic performances. In Singapore, the annual cost saving associated with the semi-automated model is estimated to be US$4·2 million, based on projected number of diabetic cases to approximately 600,000 patients by 2025.
Interpretation: For DR screening, a semi-automated model which triages retinal photographs prior to secondary human assessment is less costly but equally effective than the current full human assessment model. This demonstrates the strong economic rationale for using DLS as an assistive tool to screen for DR.
Funding: This project received funding from National Medical Research Council (NMRC) Health Service Research Grant, Ministry of Health (MOH), Singapore (National Health Innovation Center, Innovation to Develop Grant (NHIC-I2D-1409022); SingHealth Foundation Research Grant (SHF/FG648S/2015), and the Tanoto Foundation. The Singapore Diabetic Retinopathy Program (SiDRP) received funding from the MOH, Singapore (grants AIC/RPDD/SIDRP/SERI/FY2013/0018 & AIC/HPD/FY2016/0912). The Diabetes study in Nephropathy And other Microvascular complications (DYNAMO) received funding from National Medical Research Council (NMRC) Large Collaborative Grant (LCG).
Declaration of Interest: Ting Daniel SW, Lim Gilbert, Lee Mong L, Hsu Wynne, and Wong Tien Y are coinventors of a patent on the deep learning system in this paper; potential conflicts of interests are managed according to institutional policies of the Singapore Health System (SingHealth) and the National University of Singapore. All other authors declare no competing interests.
Ethical Approval: This study was approved by the Centralized Institutional Review Board (IRB) of SingHealth, Singapore (protocol number SHF/FG648S/2015) and conducted in accordance with the Declaration of Helsinki. Patients’ informed consent was exempted by the IRB due to the retrospective nature of study using fully anonymized retinal images.
更多查看译文
AI 理解论文
溯源树
样例
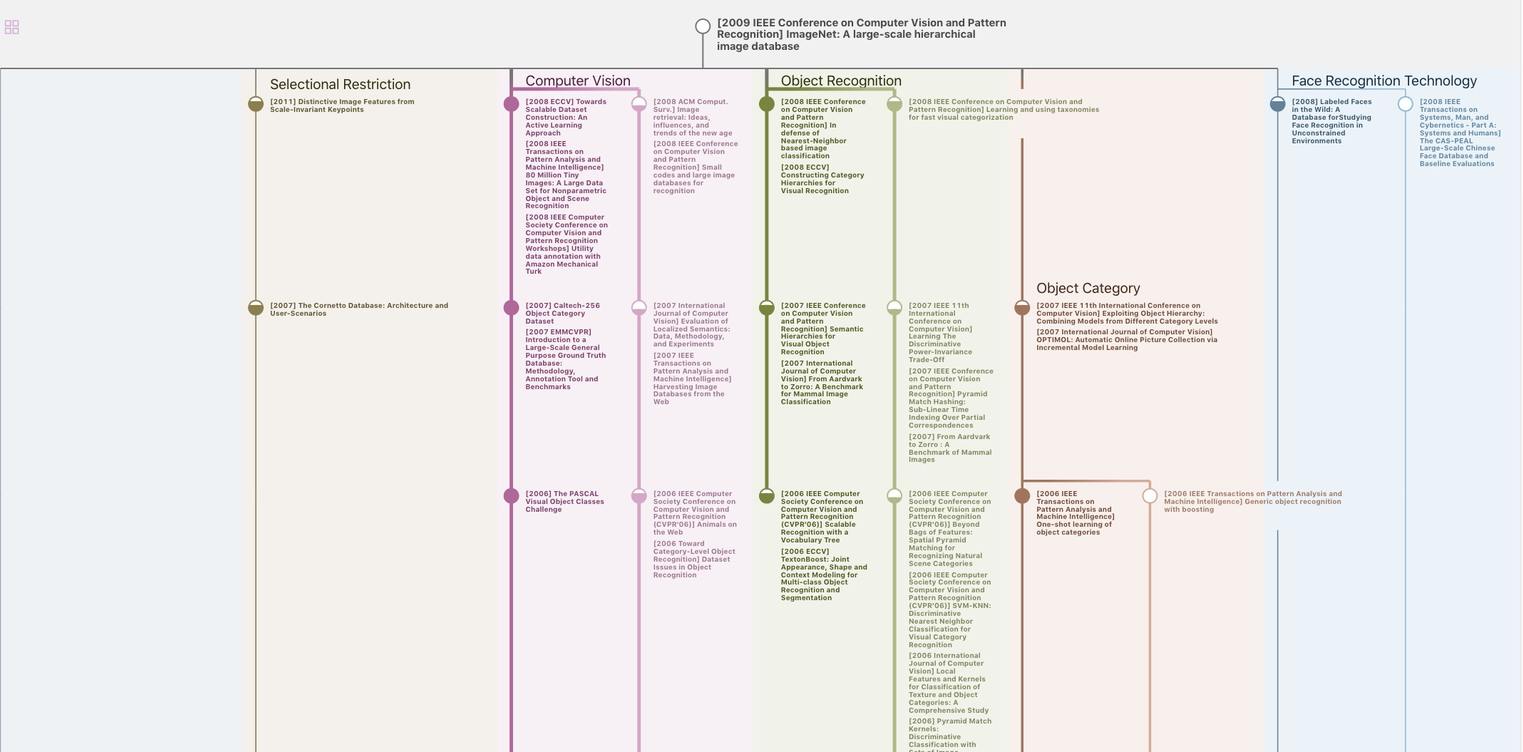
生成溯源树,研究论文发展脉络
Chat Paper
正在生成论文摘要